TacoGFN: Target-conditioned GFlowNet for Structure-based Drug Design
arxiv(2023)
摘要
Searching the vast chemical space for drug-like and synthesizable molecules
with high binding affinity to a protein pocket is a challenging task in drug
discovery. Recently, molecular deep generative models have been introduced
which promise to be more efficient than exhaustive virtual screening, by
directly generating molecules based on the protein structure. However, since
they learn the distribution of a limited protein-ligand complex dataset, the
existing methods struggle with generating novel molecules with significant
property improvements. In this paper, we frame the generation task as a
Reinforcement Learning task, where the goal is to search the wider chemical
space for molecules with desirable properties as opposed to fitting a training
data distribution. More specifically, we propose TacoGFN, a Generative Flow
Network conditioned on protein pocket structure, using binding affinity,
drug-likeliness and synthesizability measures as our reward. Empirically, our
method outperforms state-of-art methods on the CrossDocked2020 benchmark for
every molecular property (Vina score, QED, SA), while significantly improving
the generation time. TacoGFN achieves -8.82 in median docking score and
52.63% in Novel Hit Rate.
更多查看译文
AI 理解论文
溯源树
样例
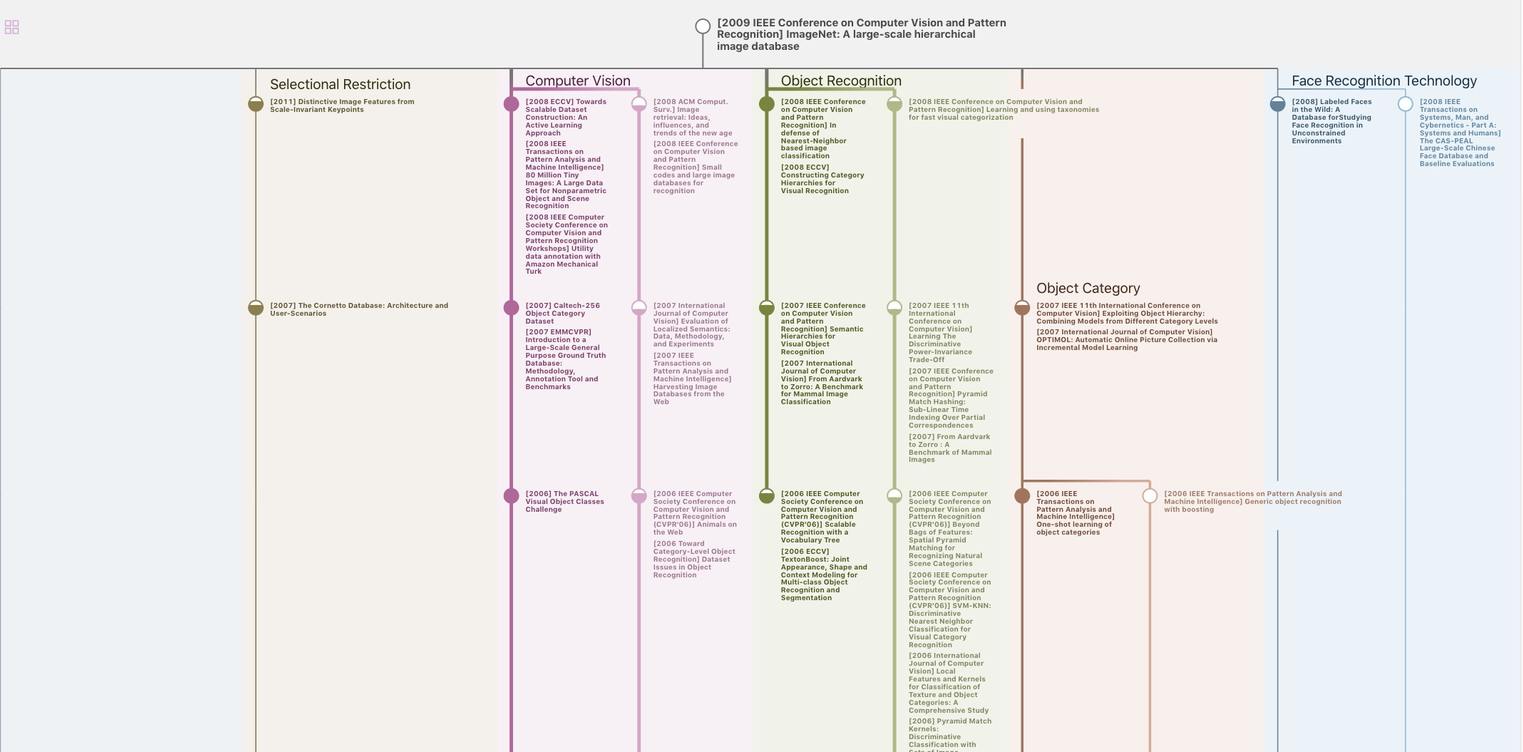
生成溯源树,研究论文发展脉络
Chat Paper
正在生成论文摘要