Prediction of adverse events risk in patients with comorbid post-traumatic stress disorder and alcohol use disorder using electronic medical records by deep learning models
DRUG AND ALCOHOL DEPENDENCE(2024)
摘要
Background: Identifying co-occurring mental disorders and elevated risk is vital for optimization of healthcare processes. In this study, we will use DeepBiomarker2, an updated version of our deep learning model to predict the adverse events among patients with comorbid post-traumatic stress disorder (PTSD) and alcohol use disorder (AUD), a high-risk population. Methods: We analyzed electronic medical records of 5565 patients from University of Pittsburgh Medical Center to predict adverse events (opioid use disorder, suicide related events, depression, and death) within 3 months at any encounter after the diagnosis of PTSD+AUD by using DeepBiomarker2. We integrated multimodal information including: lab tests, medications, co-morbidities, individual and neighborhood level social determinants of health (SDoH), psychotherapy and veteran data. Results: DeepBiomarker2 achieved an area under the receiver operator curve (AUROC) of 0.94 on the prediction of adverse events among those PTSD+AUD patients. Medications such as vilazodone, dronabinol, tenofovir, suvorexant, modafinil, and lamivudine showed potential for risk reduction. SDoH parameters such as cognitive behavioral therapy and trauma focused psychotherapy lowered risk while active veteran status, income segregation, limited access to parks and greenery, low Gini index, limited English-speaking capacity, and younger patients increased risk. Conclusions: Our improved version of DeepBiomarker2 demonstrated its capability of predicting multiple adverse event risk with high accuracy and identifying potential risk and beneficial factors.
更多查看译文
关键词
Post traumatic stress disorder,Alcohol use disorder,Social determinants of health,Artificial intelligence,Biomarker identification
AI 理解论文
溯源树
样例
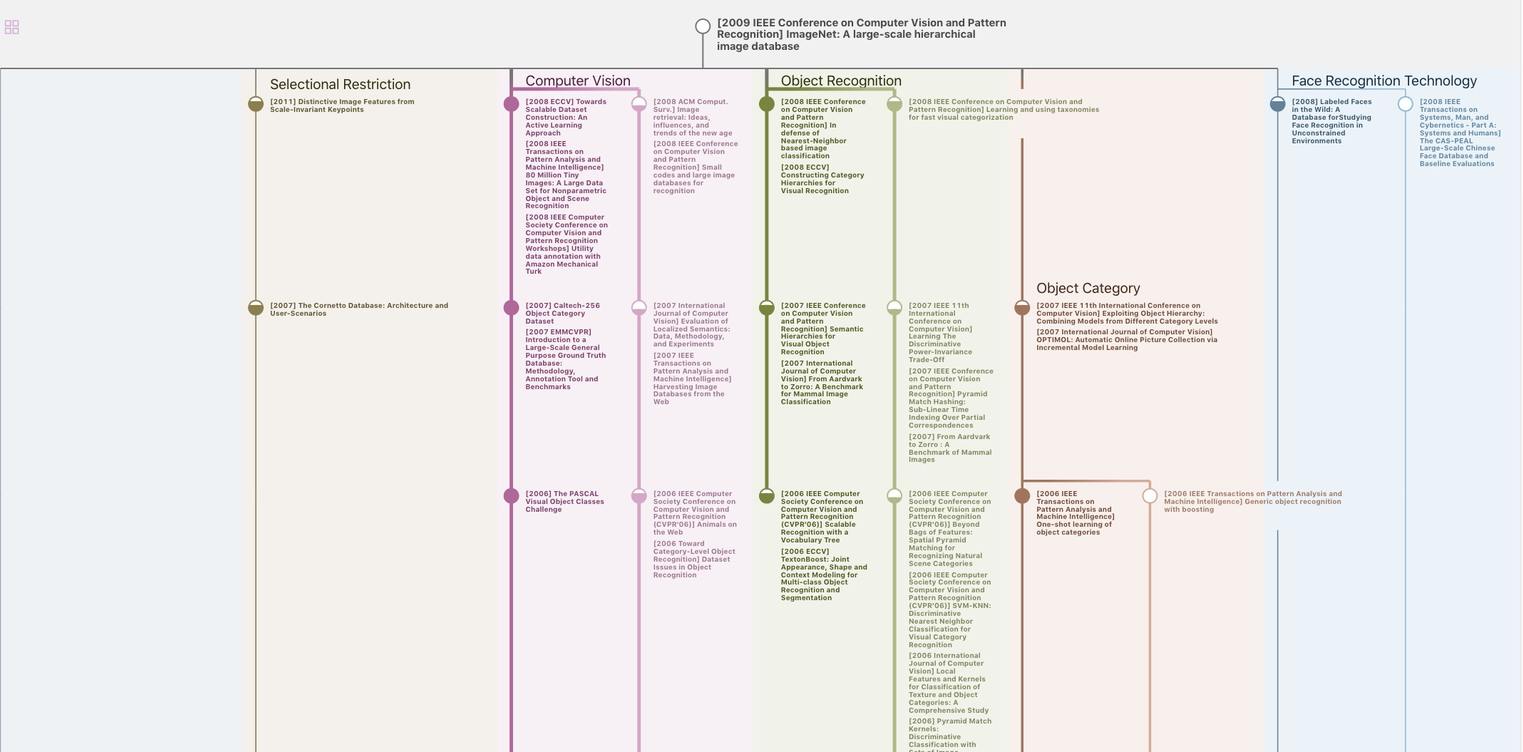
生成溯源树,研究论文发展脉络
Chat Paper
正在生成论文摘要