Proximal Policy Optimization-Based Reinforcement Learning Approach for DC-DC Boost Converter Control: A Comparative Evaluation Against Traditional Control Techniques
CoRR(2023)
摘要
This article presents a proximal policy optimization (PPO) based reinforcement learning (RL) approach for DC-DC boost converter control, which is compared to traditional control methods. The performance of the PPO algorithm is evaluated using MATLAB Simulink co-simulation, and the results demonstrate that the most efficient approach for achieving short settling time and stability is to combine the PPO algorithm with reinforcement learning based control method. The simulation results indicate that the step response characteristics provided using the control method based on RL with the PPO algorithm outperform traditional control approaches, which can be used to improve DC-DC boost converter control. This research also highlights the inherent capability of the reinforcement learning method to enhance the performance of boost converter control.
更多查看译文
关键词
learning approach,reinforcement,optimization-based
AI 理解论文
溯源树
样例
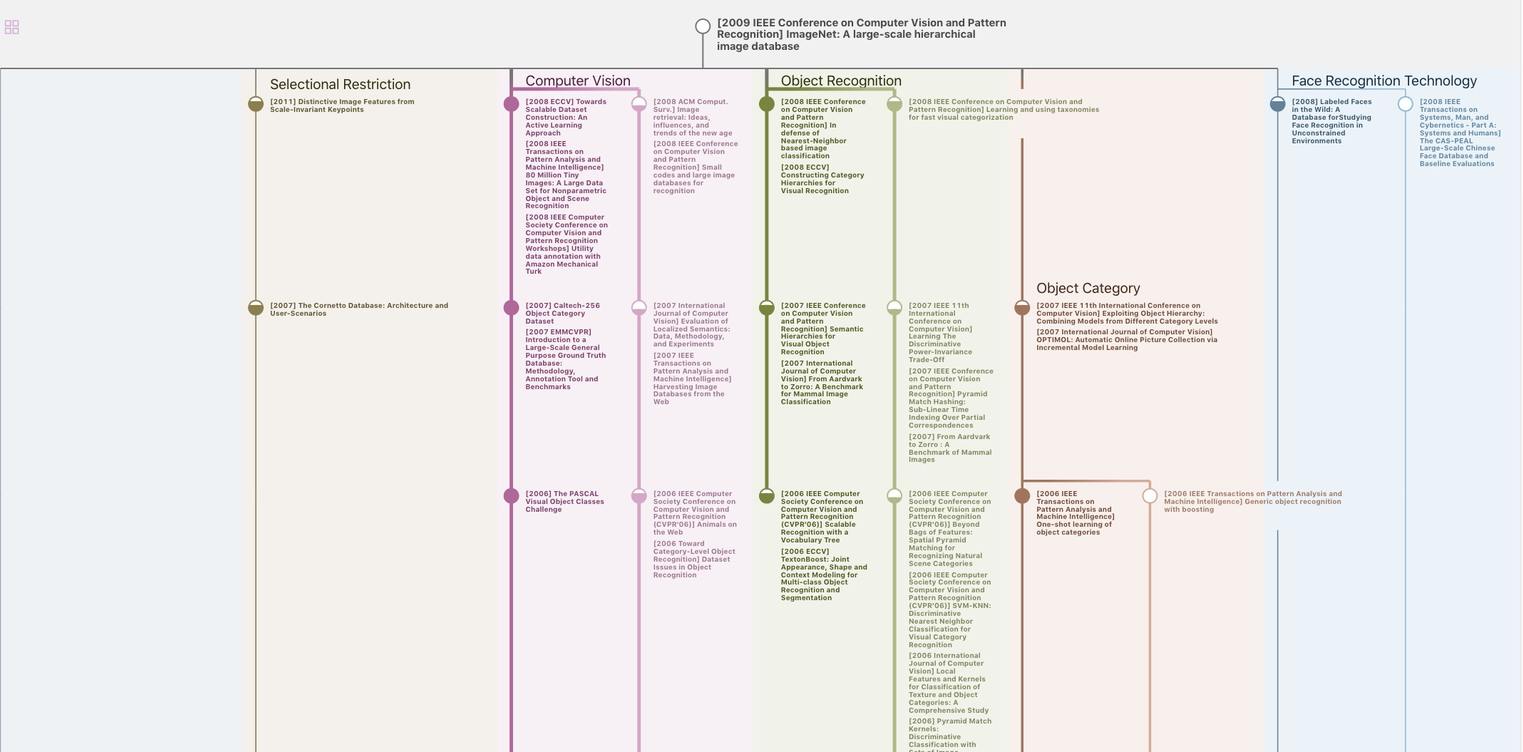
生成溯源树,研究论文发展脉络
Chat Paper
正在生成论文摘要