Heterogeneous Federated Learning Using Knowledge Codistillation
CoRR(2023)
摘要
Federated Averaging, and many federated learning algorithm variants which build upon it, have a limitation: all clients must share the same model architecture. This results in unused modeling capacity on many clients, which limits model performance. To address this issue, we propose a method that involves training a small model on the entire pool and a larger model on a subset of clients with higher capacity. The models exchange information bidirectionally via knowledge distillation, utilizing an unlabeled dataset on a server without sharing parameters. We present two variants of our method, which improve upon federated averaging on image classification and language modeling tasks. We show this technique can be useful even if only out-of-domain or limited in-domain distillation data is available. Additionally, the bi-directional knowledge distillation allows for domain transfer between the models when different pool populations introduce domain shift.
更多查看译文
关键词
heterogeneous federated learning,knowledge codistillation
AI 理解论文
溯源树
样例
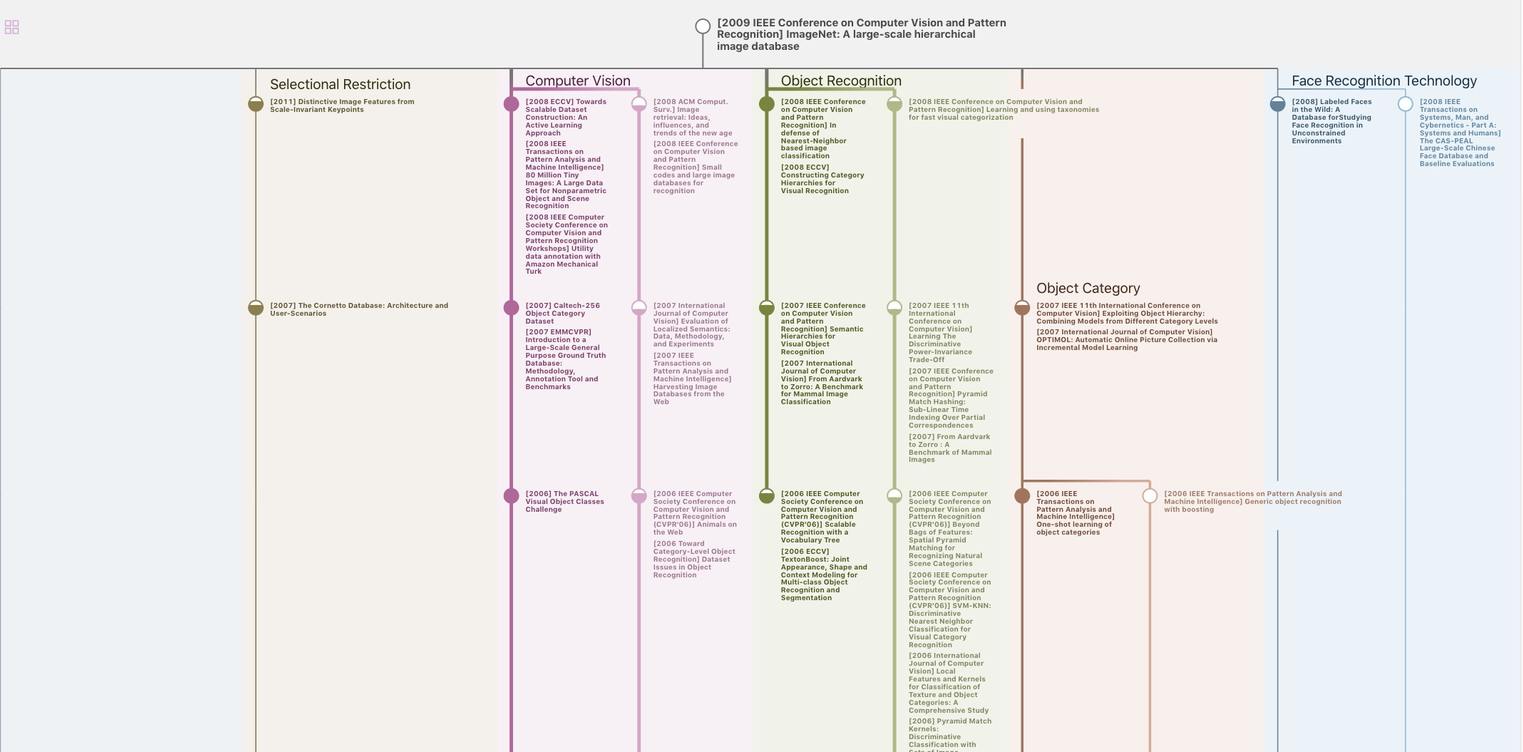
生成溯源树,研究论文发展脉络
Chat Paper
正在生成论文摘要