Prompting-based Temporal Domain Generalization
CoRR(2023)
摘要
Machine learning traditionally assumes that the training and testing data are
distributed independently and identically. However, in many real-world
settings, the data distribution can shift over time, leading to poor
generalization of trained models in future time periods. This paper presents a
novel prompting-based approach to temporal domain generalization that is
parameter-efficient, time-efficient, and does not require access to future data
during training. Our method adapts a trained model to temporal drift by
learning global prompts, domain-specific prompts, and drift-aware prompts that
capture underlying temporal dynamics. Experiments on classification,
regression, and time series forecasting tasks demonstrate the generality of the
proposed approach. The code repository will be publicly shared.
更多查看译文
关键词
generalization,prompting-based
AI 理解论文
溯源树
样例
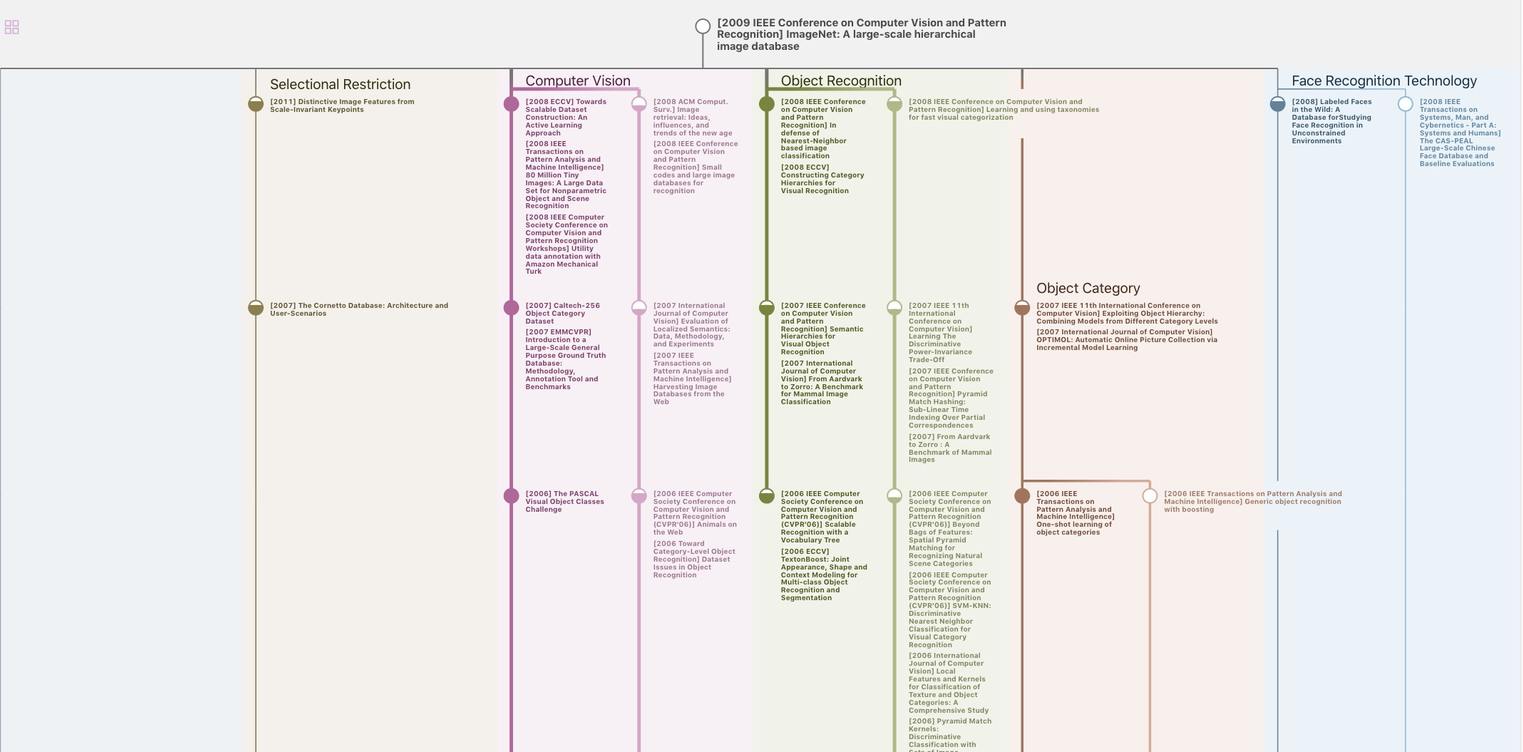
生成溯源树,研究论文发展脉络
Chat Paper
正在生成论文摘要