Large Language Models Meet Knowledge Graphs to Answer Factoid Questions
CoRR(2023)
摘要
Recently, it has been shown that the incorporation of structured knowledge into Large Language Models significantly improves the results for a variety of NLP tasks. In this paper, we propose a method for exploring pre-trained Text-to-Text Language Models enriched with additional information from Knowledge Graphs for answering factoid questions. More specifically, we propose an algorithm for subgraphs extraction from a Knowledge Graph based on question entities and answer candidates. Then, we procure easily interpreted information with Transformer-based models through the linearization of the extracted subgraphs. Final re-ranking of the answer candidates with the extracted information boosts Hits@1 scores of the pre-trained text-to-text language models by 4-6%.
更多查看译文
关键词
large language models,factoid questions,language models,knowledge graphs
AI 理解论文
溯源树
样例
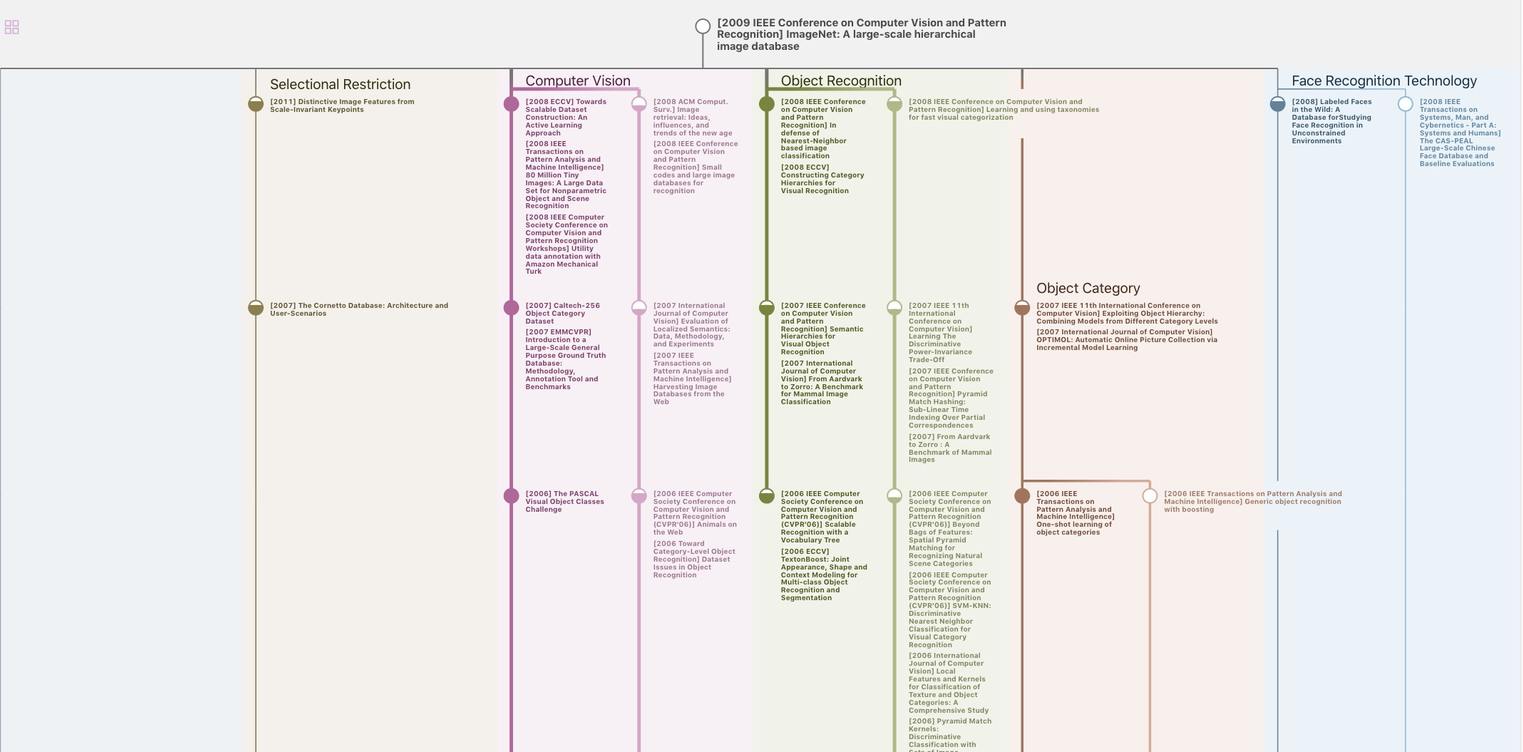
生成溯源树,研究论文发展脉络
Chat Paper
正在生成论文摘要