SONA: An Accelerator for Transform-Domain Neural Networks with Sparse-Orthogonal Weights
2023 IEEE 34th International Conference on Application-specific Systems, Architectures and Processors (ASAP)(2023)
摘要
Recent advances in model pruning have enabled sparsity-aware deep neural network accelerators that improve the energy-efficiency and performance of inference tasks. We introduce SONA, a novel transform-domain neural network accelerator in which convolution operations are replaced by element-wise multiplications with sparse-orthogonal weights. SONA employs an output stationary dataflow coupled with an energy-efficient memory organization to reduce the overhead of sparse-orthogonal transform-domain kernels that are concurrently processed without any conflicts. Weights in SONA are non-uniformly quantized with bit-sparse canonical-signed-digit representations to reduce multiplications to simple additions. Moreover, for sparse fully-connected layers (FCLs), SONA introduces column-based-block structured pruning, which is integrated into the same architecture that maintains full multiply-and-accumulate (MAC) array utilization. Compared to prior dense and sparse neural networks accelerators, SONA can reduce inference energy by
$5.1\times$
and
$2.4 \times$
and increase performance by
$5.2\times$
and
$2.1\times$
, respectively, for convolution layers. For sparse FCLs, SONA can reduce inference energy by
$2.4\times$
and increase performance by
$2\times$
compared to prior work.
更多查看译文
AI 理解论文
溯源树
样例
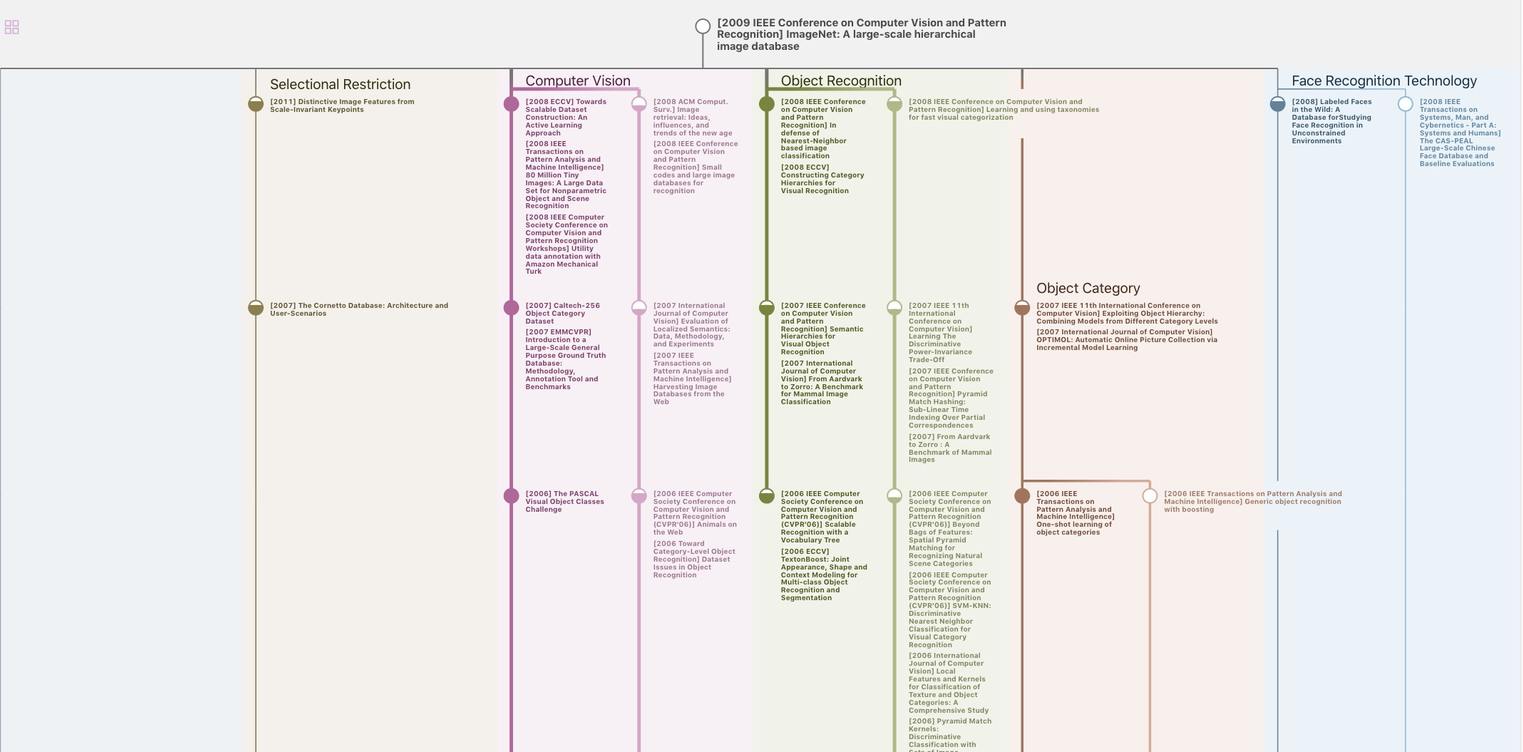
生成溯源树,研究论文发展脉络
Chat Paper
正在生成论文摘要