Pink: Unveiling the Power of Referential Comprehension for Multi-modal LLMs
arXiv (Cornell University)(2023)
摘要
Multi-modal Large Language Models (MLLMs) have shown remarkable capabilities
in various multi-modal tasks. Nevertheless, their performance in fine-grained
image understanding tasks is still limited. To address this issue, this paper
proposes a new framework to enhance the fine-grained image understanding
abilities of MLLMs. Specifically, we present a new method for constructing the
instruction tuning dataset at a low cost by leveraging annotations in existing
datasets. A self-consistent bootstrapping method is also introduced to extend
existing dense object annotations into high-quality
referring-expression-bounding-box pairs. These methods enable the generation of
high-quality instruction data which includes a wide range of fundamental
abilities essential for fine-grained image perception. Moreover, we argue that
the visual encoder should be tuned during instruction tuning to mitigate the
gap between full image perception and fine-grained image perception.
Experimental results demonstrate the superior performance of our method. For
instance, our model exhibits a 5.2
and surpasses the accuracy of Kosmos-2 by 24.7
attained the top rank on the leaderboard of MMBench. This promising performance
is achieved by training on only publicly available data, making it easily
reproducible. The models, datasets, and codes are publicly available at
https://github.com/SY-Xuan/Pink.
更多查看译文
关键词
referential comprehension,multi-modal
AI 理解论文
溯源树
样例
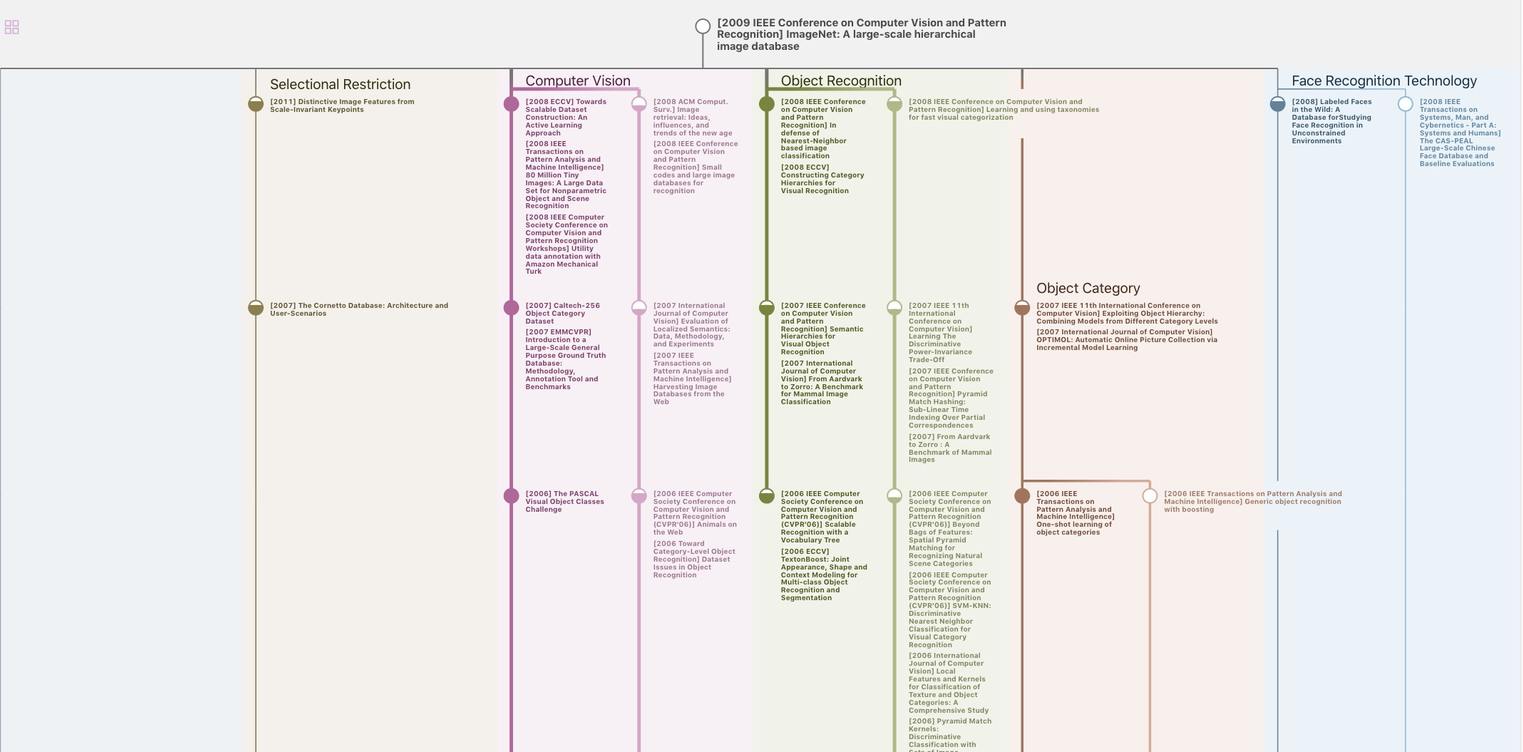
生成溯源树,研究论文发展脉络
Chat Paper
正在生成论文摘要