DGDTA: dynamic graph attention network for predicting drug–target binding affinity
BMC bioinformatics(2023)
摘要
Background Obtaining accurate drug–target binding affinity (DTA) information is significant for drug discovery and drug repositioning. Although some methods have been proposed for predicting DTA, the features of proteins and drugs still need to be further analyzed. Recently, deep learning has been successfully used in many fields. Hence, designing a more effective deep learning method for predicting DTA remains attractive. Results Dynamic graph DTA (DGDTA), which uses a dynamic graph attention network combined with a bidirectional long short-term memory (Bi-LSTM) network to predict DTA is proposed in this paper. DGDTA adopts drug compound as input according to its corresponding simplified molecular input line entry system (SMILES) and protein amino acid sequence. First, each drug is considered a graph of interactions between atoms and edges, and dynamic attention scores are used to consider which atoms and edges in the drug are most important for predicting DTA. Then, Bi-LSTM is used to better extract the contextual information features of protein amino acid sequences. Finally, after combining the obtained drug and protein feature vectors, the DTA is predicted by a fully connected layer. The source code is available from GitHub at https://github.com/luojunwei/DGDTA . Conclusions The experimental results show that DGDTA can predict DTA more accurately than some other methods.
更多查看译文
关键词
Drug-target binding affinity,Dynamic graph attention network,Long short-term memory,Drug discovery
AI 理解论文
溯源树
样例
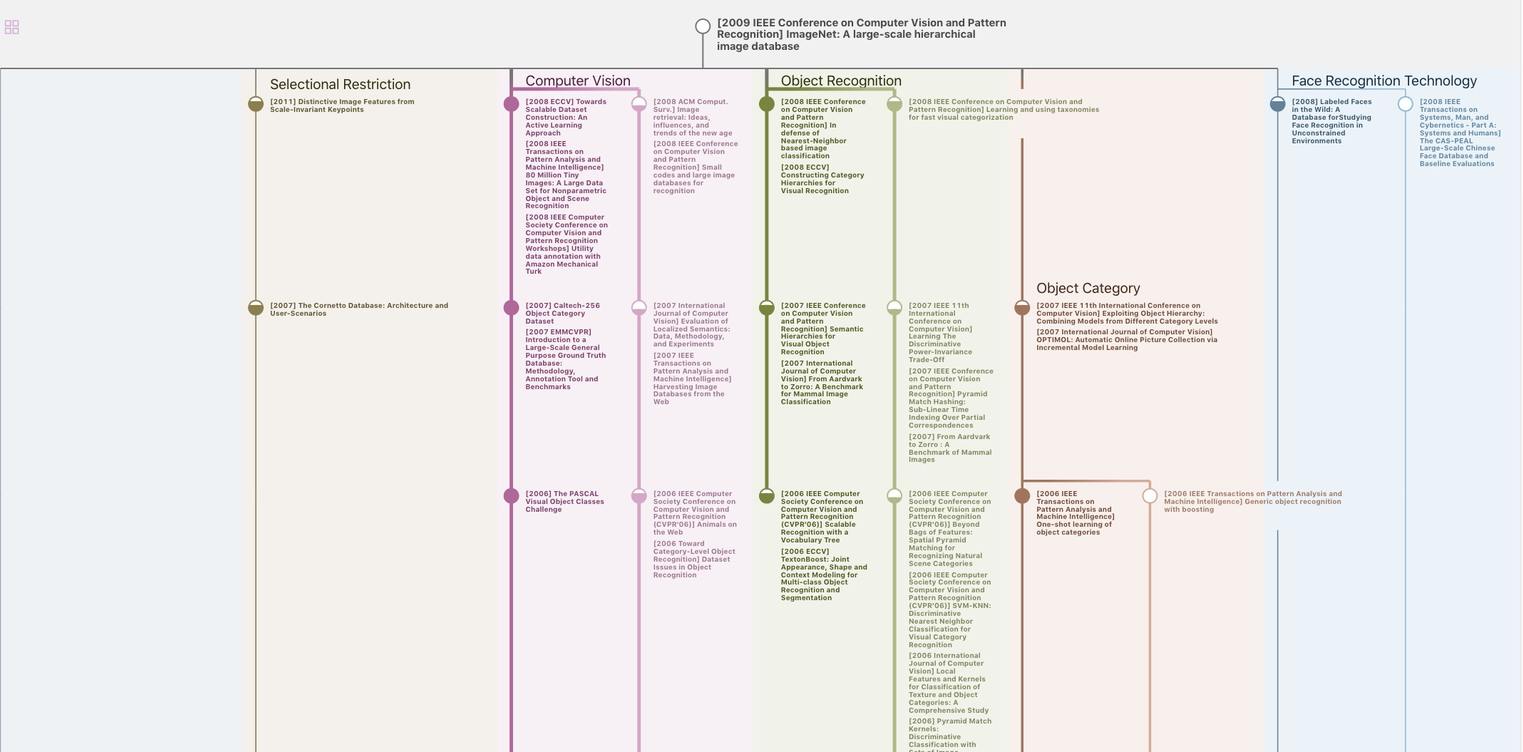
生成溯源树,研究论文发展脉络
Chat Paper
正在生成论文摘要