Machine learning models based on residue interaction network for ABCG2 transportable compounds recognition
Environmental pollution (Barking, Essex : 1987)(2023)
摘要
As the one of the most important protein of placental transport of environmental substances, the identification of ABCG2 transport molecules is the key step for assessing the risk of placental exposure to environmental chemicals. Here, residue interaction network (RIN) was used to explore the difference of ABCG2 binding conformations between transportable and non-transportable compounds. The RIN were treated as a kind of special quantitative data of protein conformation, which not only reflected the changes of single amino acid conformation in protein, but also indicated the changes of distance and action type between amino acids. Based on the quantitative RIN, four machine learning algorithms were applied to establish the classification and recognition model for 1100 compounds with transported by ABCG2 potential. The random forest (RF) models constructed with RIN presented the best and satisfied predictive ability with an accuracy of training set of 0.97 and the test set of 0.96 respectively. In conclusion, the construction of residue interaction network provided a new perspective for the quantitative characterization of protein conformation and the establishment of prediction models for transporter molecular recognition. The ABCG2 transport molecular recognition model based on residue interaction network provides a possible way for screening environmental chemistry transported through placenta.
更多查看译文
关键词
ABCG2,Placental transport,Residue interaction network,Machine learning
AI 理解论文
溯源树
样例
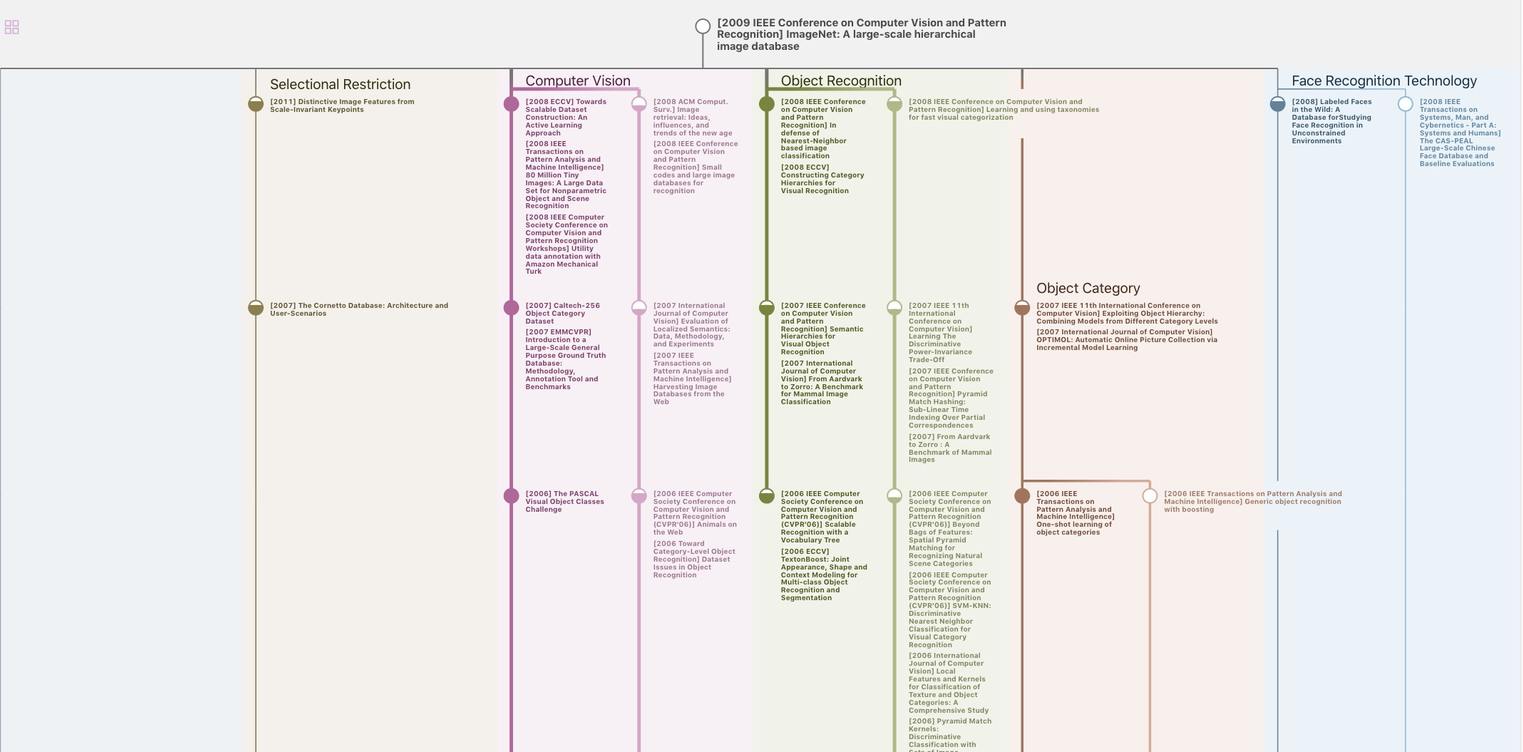
生成溯源树,研究论文发展脉络
Chat Paper
正在生成论文摘要