Oscillation Damping Using Reinforcement Learning Controlled HVDC Transmission
2023 IEEE PES GTD International Conference and Exposition (GTD)(2023)
摘要
This paper presents a novel deep reinforcement learning based control method for damping power system inter-area oscillations. A guided surrogate-gradient-based evolutionary strategy (GSES) is used to control the transferred power on high voltage DC (HVDC) transmissions, which could help improve the damping effect when inter-area oscillations occur. The GSES algorithm trains a reinforcement learning agent to learn the best parameters of the HVDC controller, with an objective of reducing the magnitude and duration of inter-area oscillations. Unlike many existing reinforcement learning methods, the GSES algorithm does not require a traditional back-propagation process to update the policy parameters, enabling an easier, more robust and efficient training procedure with less required hyper-parameters. In addition, as an evolutionary strategy, the proposed GSES-based HVDC oscillation damping control approach can engage with multiple individual workers during the training process through parallel computation techniques, which significantly accelerates the computational speed and improves the training efficiency. The proposed GSES-based HVDC controller is compared with a conventional oscillation damping method on the IEEE 39-Bus New England system. Results indicate that the proposed GSES-based HVDC control approach performs better and it can effectively damp power system inter-area oscillations.
更多查看译文
关键词
High voltage DC transmission,inter-area oscillation,deep reinforcement learning,transient stability
AI 理解论文
溯源树
样例
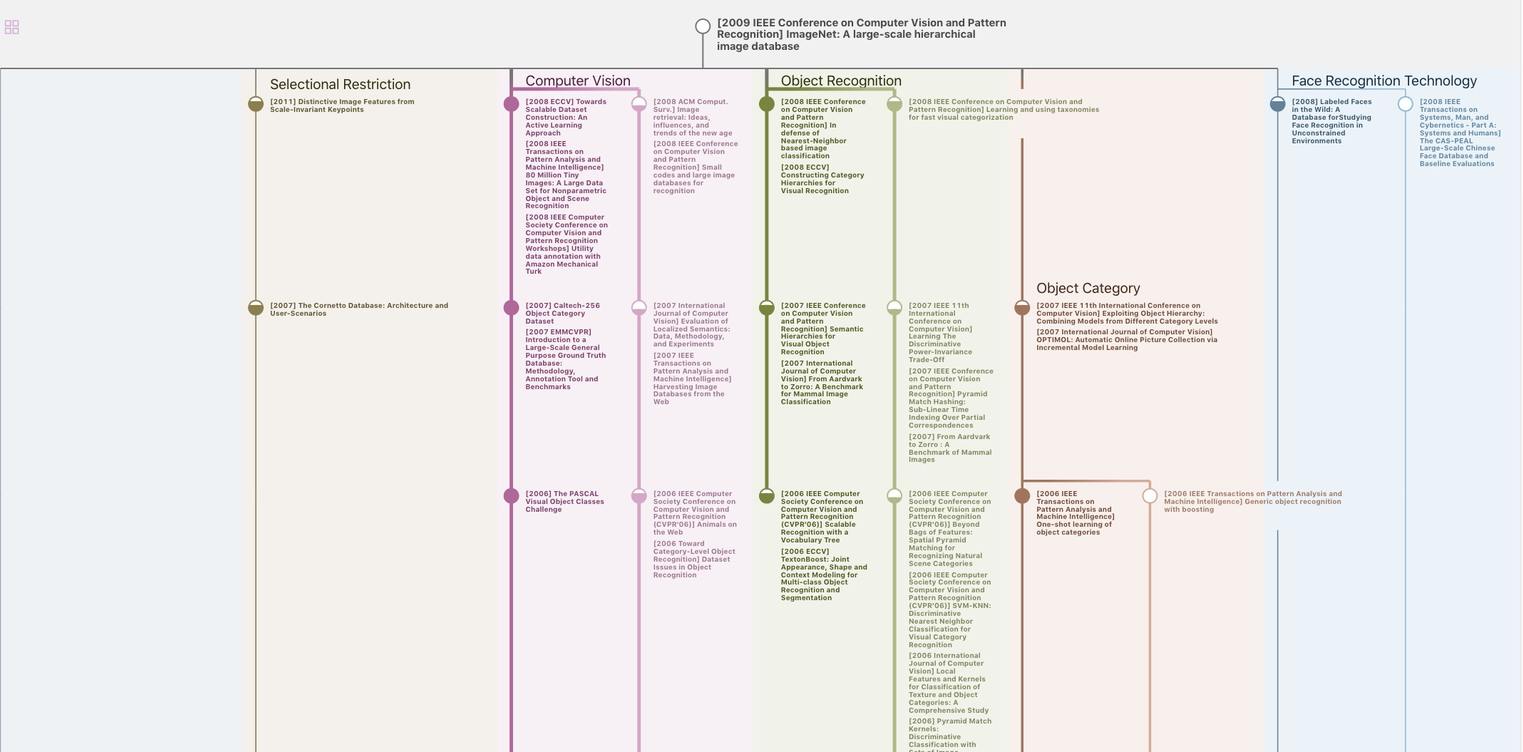
生成溯源树,研究论文发展脉络
Chat Paper
正在生成论文摘要