RepCo: Replenish sample views with better consistency for contrastive learning
Neural networks : the official journal of the International Neural Network Society(2023)
摘要
Contrastive learning methods aim to learn shared representations by minimizing distances between positive pairs, and maximizing distances between negative pairs in the embedding space. To achieve better performance of contrastive learning, one of the key problems is to design appropriate sample pairs. In most previous works, random cropping on the input image is utilized to obtain two views as positive pairs. However, such strategies lead to suboptimal performance since the sampled crops may have inconsistent semantic information, which consequently degrades the quality of contrastive views. To address this limitation, we explore to replenish sample views with better consistency of the image and propose a novel self-supervised learning (SSL) framework RepCo. Instead of searching for semantically consistent patches between two different views, we select patches on the same image as the replenishment of positive/negative pairs, encourage patches that are similar but come from different positions as positive pairs, and force patches that are dissimilar but come from adjacent positions to have different representations, i.e. construct negative pairs to enrich the learned representations. Our method effectively generates high-quality contrastive views, explores the untapped semantic consistency on images, and provides more informative representations for downstream tasks. Experiments on adequate downstream tasks have shown that, our approach achieves +2.1 AP50 (COCO pre-trained) and +1.6 AP50 (ImageNet pre-trained) gains on Pascal VOC object detection, +2.3 mIoU gains on Cityscapes semantic segmentation, respectively.
更多查看译文
关键词
Contrastive learning,Sampling strategy,Self-supervised pretraining
AI 理解论文
溯源树
样例
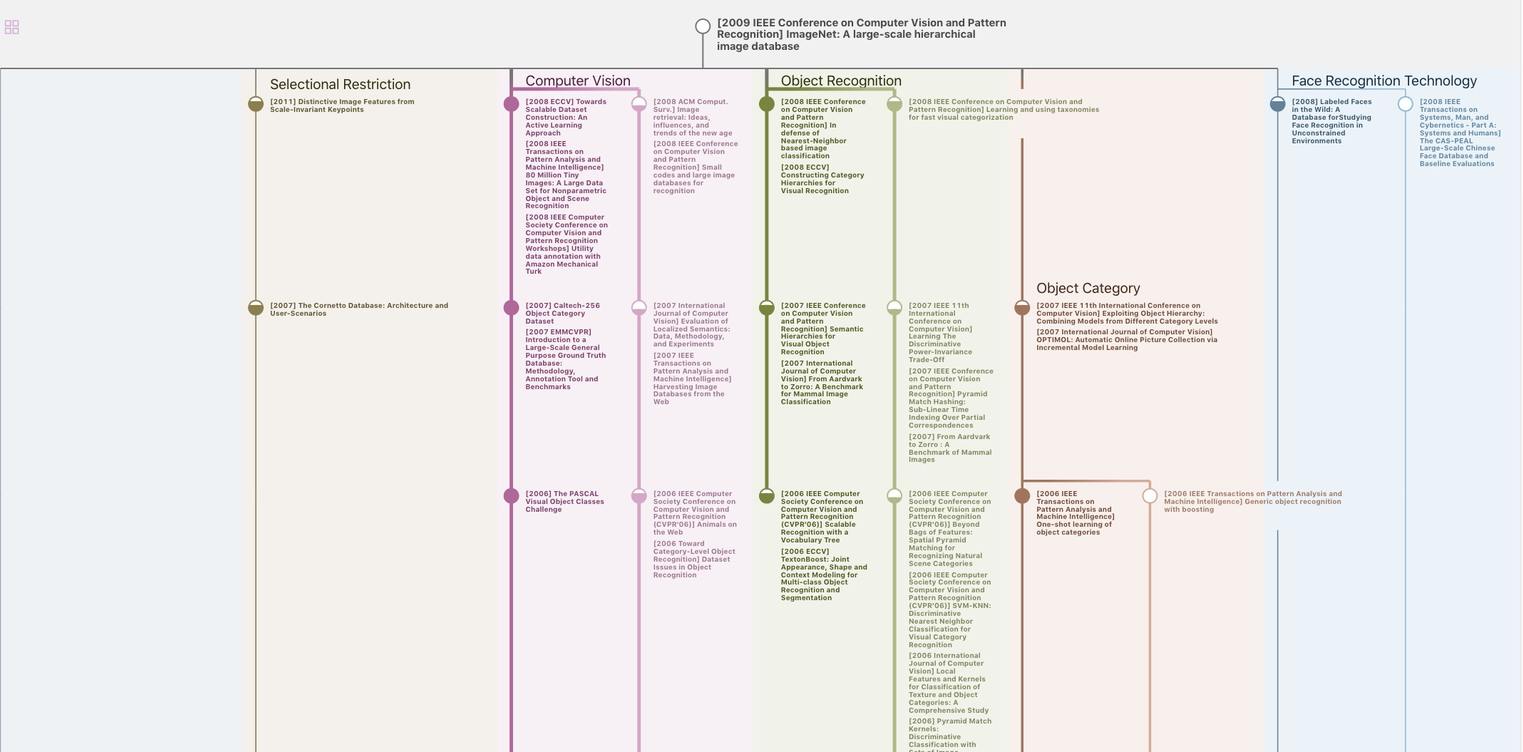
生成溯源树,研究论文发展脉络
Chat Paper
正在生成论文摘要