Two-Step Active Learning for Instance Segmentation with Uncertainty and Diversity Sampling
CoRR(2023)
摘要
Training high-quality instance segmentation models requires an abundance of labeled images with instance masks and classifications, which is often expensive to procure. Active learning addresses this challenge by striving for optimum performance with minimal labeling cost by selecting the most informative and representative images for labeling. Despite its potential, active learning has been less explored in instance segmentation compared to other tasks like image classification, which require less labeling. In this study, we propose a post-hoc active learning algorithm that integrates uncertainty-based sampling with diversity-based sampling. Our proposed algorithm is not only simple and easy to implement, but it also delivers superior performance on various datasets. Its practical application is demonstrated on a real-world overhead imagery dataset, where it increases the labeling efficiency fivefold.
更多查看译文
关键词
instance segmentation,active,learning,two-step
AI 理解论文
溯源树
样例
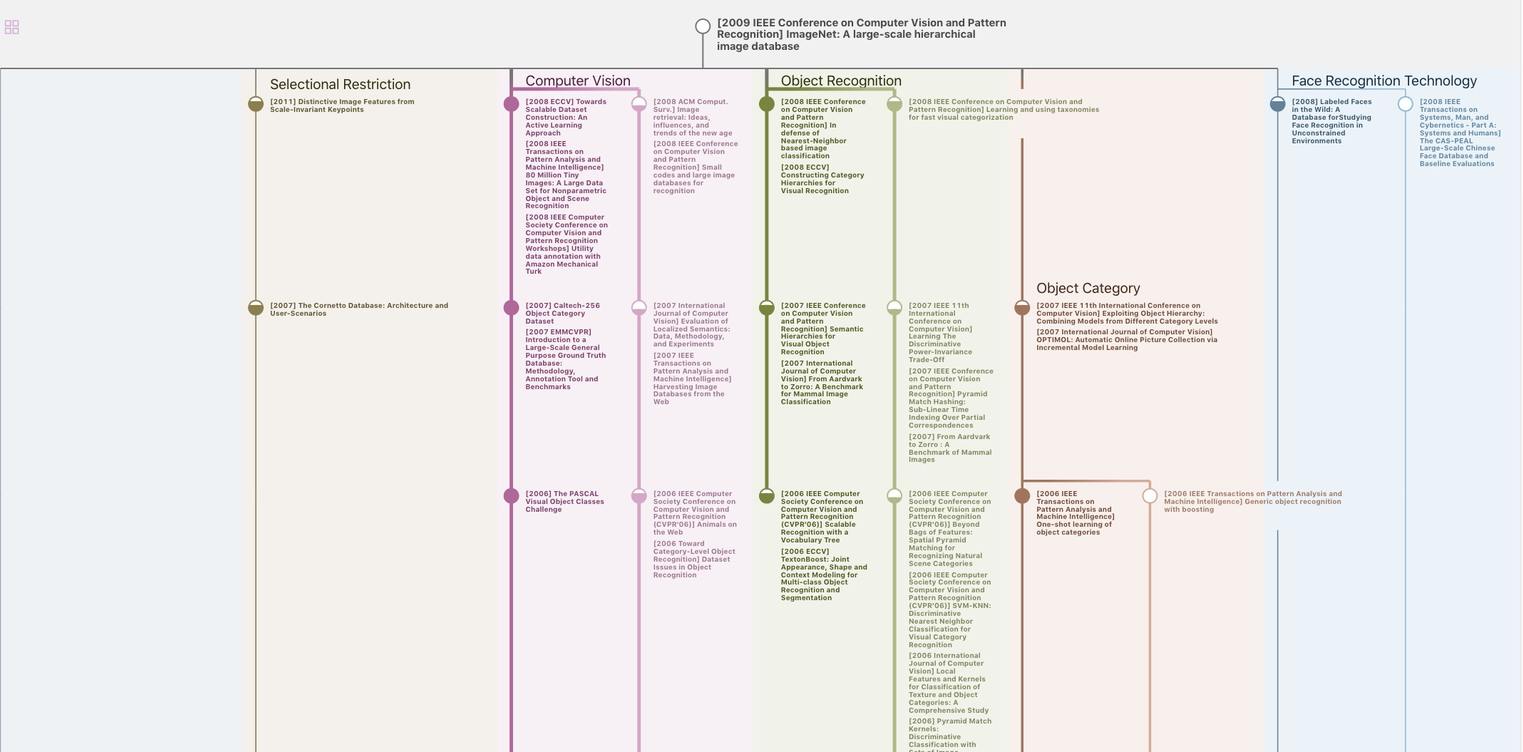
生成溯源树,研究论文发展脉络
Chat Paper
正在生成论文摘要