Compositional Sculpting of Iterative Generative Processes
NeurIPS(2023)
摘要
High training costs of generative models and the need to fine-tune them for specific tasks have created a strong interest in model reuse and composition. A key challenge in composing iterative generative processes, such as GFlowNets and diffusion models, is that to realize the desired target distribution, all steps of the generative process need to be coordinated, and satisfy delicate balance conditions. In this work, we propose Compositional Sculpting: a general approach for defining compositions of iterative generative processes. We then introduce a method for sampling from these compositions built on classifier guidance. We showcase ways to accomplish compositional sculpting in both GFlowNets and diffusion models. We highlight two binary operations $\unicode{x2014}$ the harmonic mean ($p_1 \otimes p_2$) and the contrast ($p_1 \unicode{x25D1}\,p_2$) between pairs, and the generalization of these operations to multiple component distributions. We offer empirical results on image and molecular generation tasks.
更多查看译文
关键词
compositional sculpting,iterative generative processes,generative processes
AI 理解论文
溯源树
样例
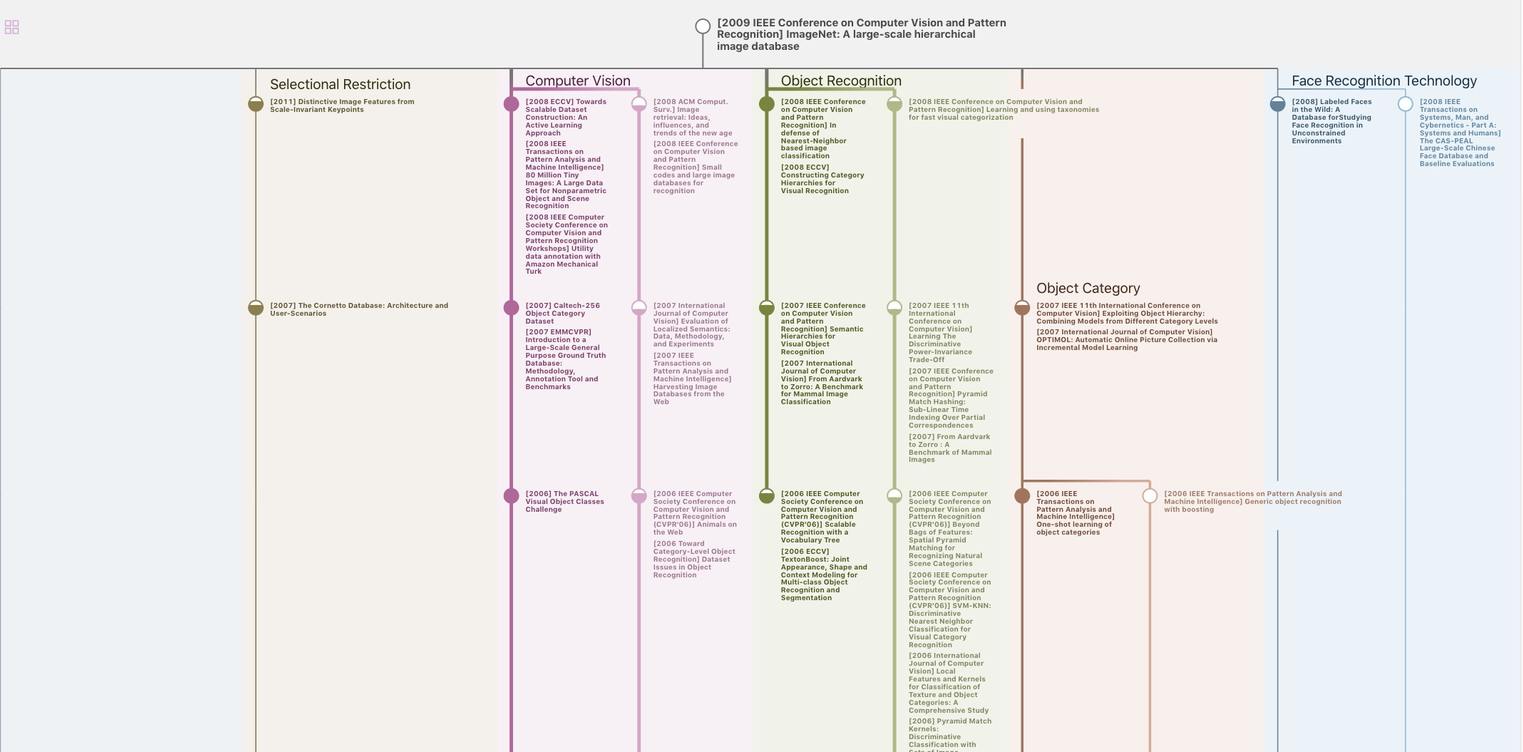
生成溯源树,研究论文发展脉络
Chat Paper
正在生成论文摘要