Towards Best Practices of Activation Patching in Language Models: Metrics and Methods
ICLR 2024(2023)
摘要
Mechanistic interpretability seeks to understand the internal mechanisms of
machine learning models, where localization – identifying the important model
components – is a key step. Activation patching, also known as causal tracing
or interchange intervention, is a standard technique for this task (Vig et al.,
2020), but the literature contains many variants with little consensus on the
choice of hyperparameters or methodology. In this work, we systematically
examine the impact of methodological details in activation patching, including
evaluation metrics and corruption methods. In several settings of localization
and circuit discovery in language models, we find that varying these
hyperparameters could lead to disparate interpretability results. Backed by
empirical observations, we give conceptual arguments for why certain metrics or
methods may be preferred. Finally, we provide recommendations for the best
practices of activation patching going forwards.
更多查看译文
关键词
language model interpretability,interpretability,mechanistic interpretability,circuit analysis,activation patching,large language models
AI 理解论文
溯源树
样例
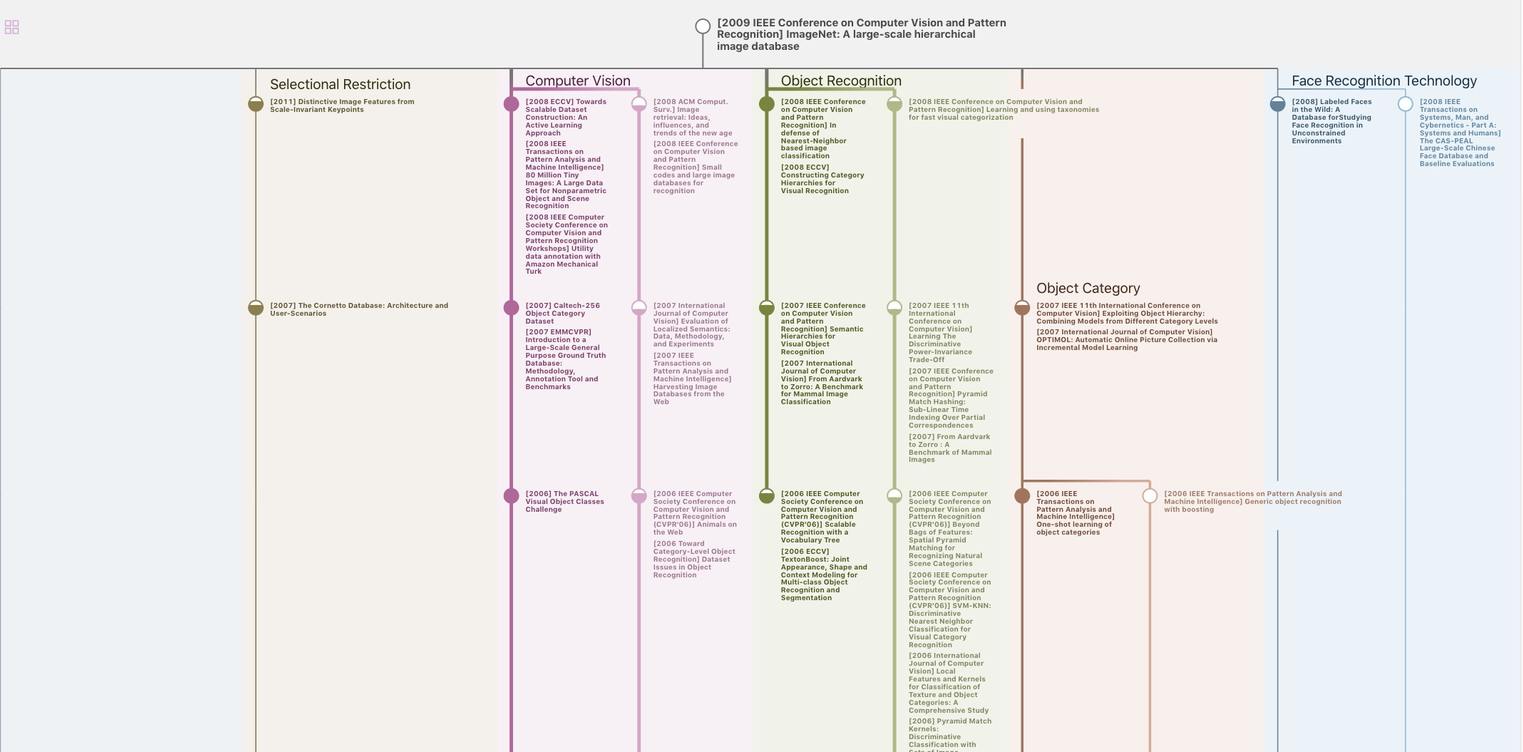
生成溯源树,研究论文发展脉络
Chat Paper
正在生成论文摘要