Impact of Training Set Size on Resource Usage of Machine Learning Models for IoT Network Intrusion Detection
2023 19th International Conference on Distributed Computing in Smart Systems and the Internet of Things (DCOSS-IoT)(2023)
摘要
Security is a critical concern in Internet-of-Things (IoT) environments, including industrial IoT and one solution to enhance security is to deploy Network Intrusion Detection Systems (NIDS) using machine learning (ML) models in edge such as gateway devices. However, the resource constraints of these devices can pose challenges for implementing ML models. This study examines the impact of different training set sizes on the performance and resource usage of One-Class ML models that seem particularly adequate to this use case. The results indicate that One-Class Support Vector Machine, Isolation Forest, and Elliptic Envelope models are suitable for resource-constrained devices due to their low model size, classification time, and consistent performance with increasing sample size. The Local Outlier Factor model exhibited a high detection rate and low false alarm rate at the cost of high model size and classification time. Our results can help develop more efficient and effective network intrusion detection for IoT systems.
更多查看译文
关键词
classification time,intrusion detection,IoT network,machine learning,computing resources usage
AI 理解论文
溯源树
样例
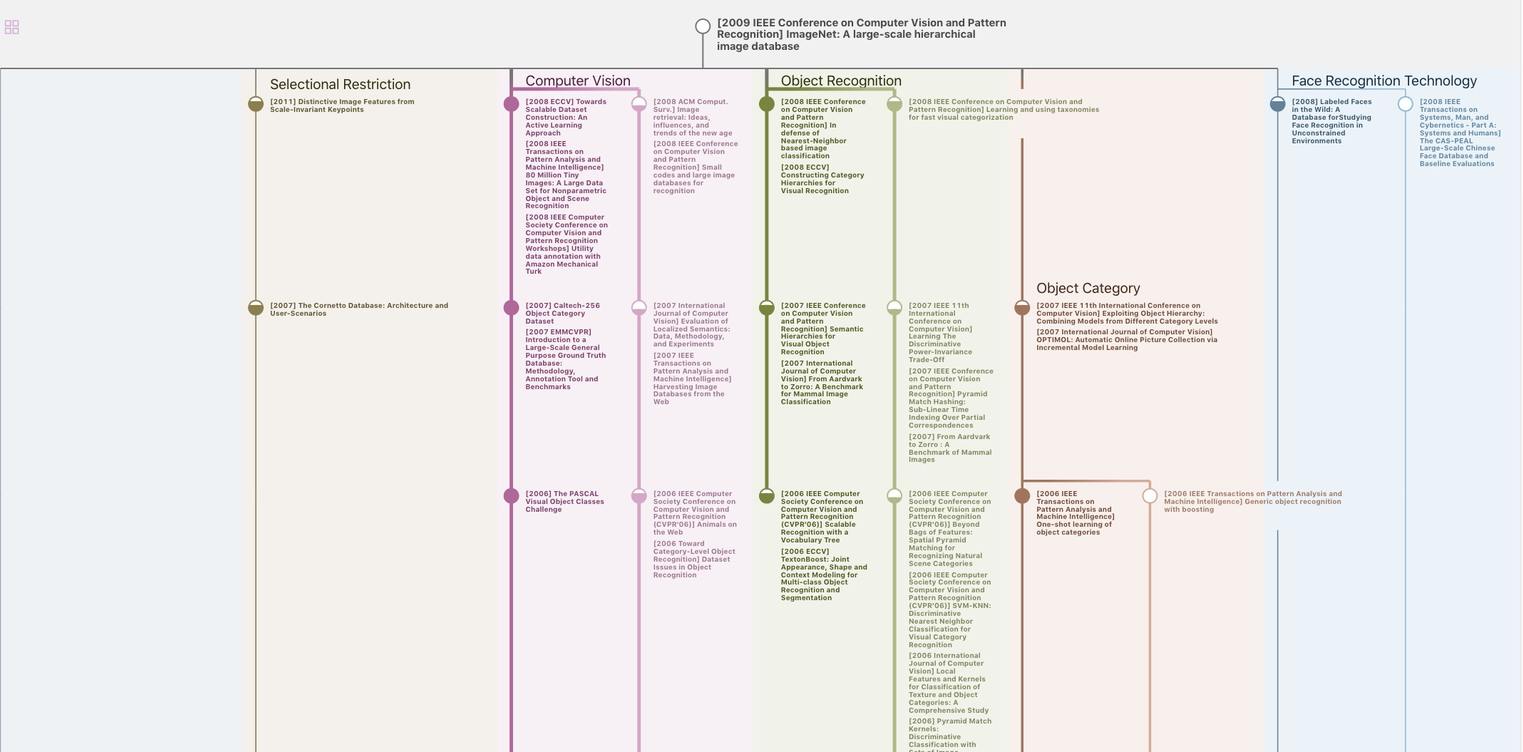
生成溯源树,研究论文发展脉络
Chat Paper
正在生成论文摘要