Realizing Predictive Maintenance in Production Machinery Through Low-Cost IIoT Framework and Anomaly Detection: A Case Study in a Real-World Manufacturing Environment
2023 19th International Conference on Distributed Computing in Smart Systems and the Internet of Things (DCOSS-IoT)(2023)
摘要
Digital transformation and predictive maintenance are still some of the key challenges faced by the industrial sector as it moves towards Industry 4.0. However, the lack of resources, experienced personnel, and the absence of digital-savvy culture in manufacturing companies, often hinder the adoption of the required technologies. In this context, maintenance is a required process to ensure the smooth and continuous operation of the production, but to this day, engineers are still required to physically inspect equipment on site. By applying digital technologies, a predictive maintenance framework will enable the industry to maximize the life of the equipment and to act on time in order to prevent failures and thus reduce costs and optimize maintenance efficiency. In this effort, integrating such technologies and the required modern computing devices for monitoring legacy industrial equipment remains a challenge. Therefore, in this paper, we present a low-cost IIoT system for an AI-based predictive maintenance of machinery in a real-world industrial environment. In specific, a data collection system, consisting of a Raspberry Pi device and several sensors (namely, an accelerometer, temperature sensors and a microphone), was designed and developed. The collected data was then utilized to feed a deep-learning LSTM-autoencoder model for anomaly detection through a semi-supervised learning approach. Despite the small amount of data collected, the model is able to effectively detect anomalies. Finally, ways in which the existing system can be improved are proposed.
更多查看译文
关键词
predictive maintenance,anomaly detection,Industrial Internet of Things,machine learning,motor reducer
AI 理解论文
溯源树
样例
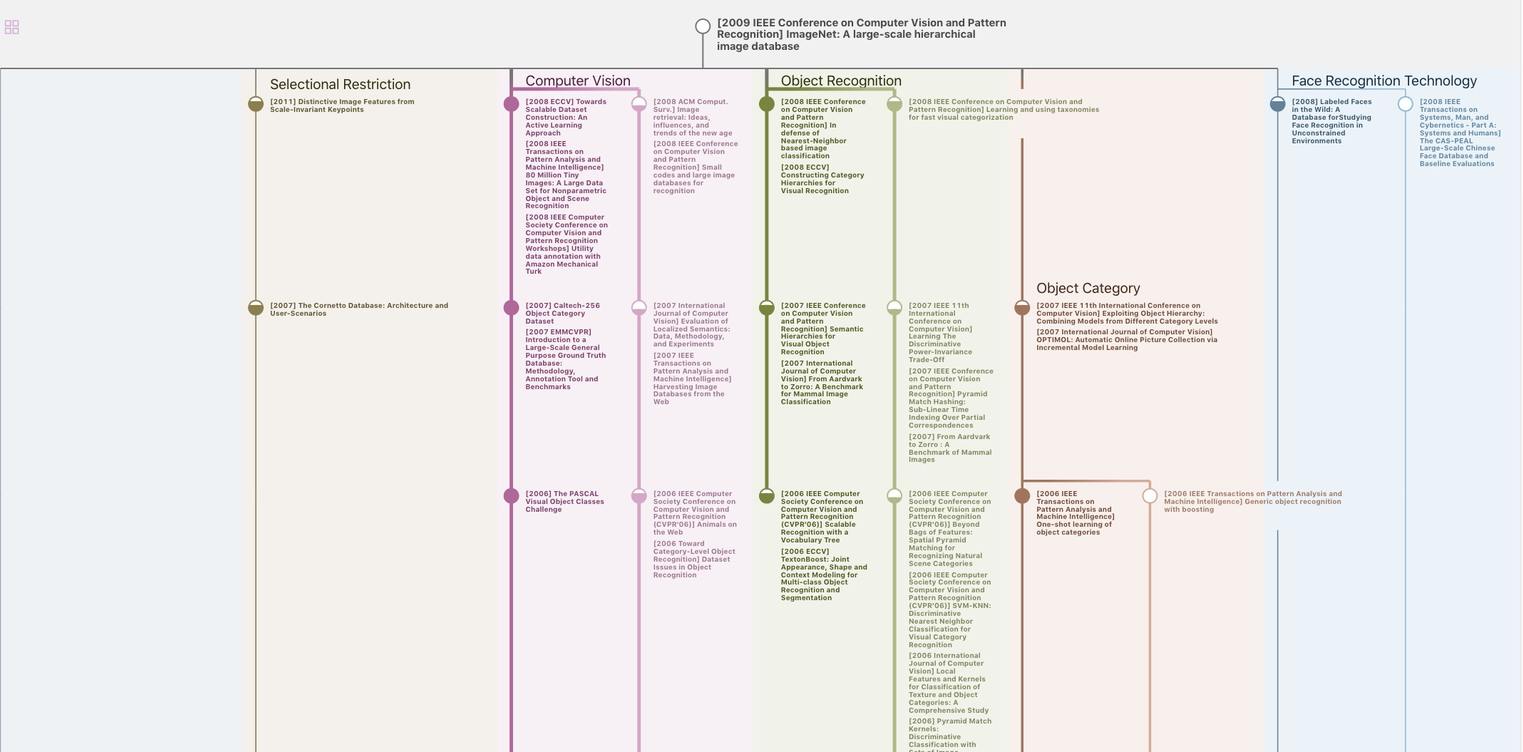
生成溯源树,研究论文发展脉络
Chat Paper
正在生成论文摘要