Multi-Class Industrial Texture-less Object Detection Method in Cluttered and Occluded Scenes
2023 WRC Symposium on Advanced Robotics and Automation (WRC SARA)(2023)
摘要
in industrial scenes, template matching algorithms have the advantages of robustness and fastness, but most of them tend to focus on single-class object detection. When facing multi-class object detection tasks, the performance degrades significantly. Deep neural networks are naturally suitable for this task, but rotation regression is still a problem that has yet to be solved perfectly, making it challenging to meet the requirements of industrial robot sorting and grasping scenes. We proposed a hybrid two-stage method called key-pair matching (KPM) algorithm, which can perform robust, fast, and accurate detection of multi-class industrial workpieces. In the first stage, we choose one off-the-shelf CNN detector to predict the horizontal bounding boxes to solve the classification and coarse localization problems in complex scenarios, in the second stage, features based on key-shapes primitives are used to calculate translations and rotations. Experiments show that our proposed method outperform the other comparison methods regarding detection performance and localization accuracy under reasonable runtime, making it suitable for industrial objects detection in cluttered and occlusion scenes.
更多查看译文
AI 理解论文
溯源树
样例
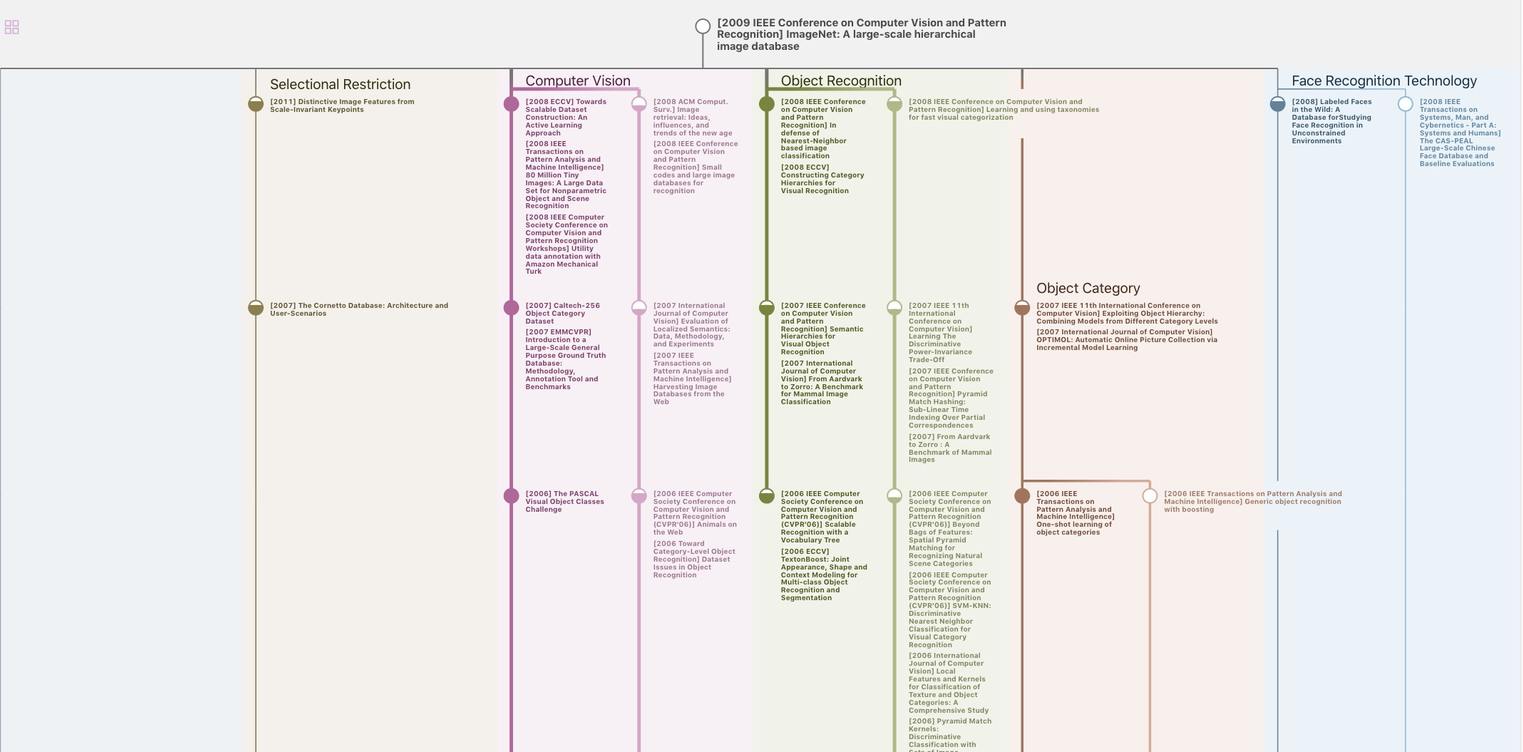
生成溯源树,研究论文发展脉络
Chat Paper
正在生成论文摘要