Further analysis of cGAN: A system for Generative Deep Learning Post-processing of Precipitation
arXiv (Cornell University)(2023)
摘要
The conditional generative adversarial rainfall model "cGAN" developed for the UK \cite{Harris22} was trained to post-process into an ensemble and downscale ERA5 rainfall to 1km resolution over three regions of the USA and the UK. Relative to radar data (stage IV and NIMROD), the quality of the forecast rainfall distribution was quantified locally at each grid point and between grid points using the spatial correlation structure. Despite only having information from a single lower quality analysis, the ensembles of post processed rainfall produced were found to be competitive with IFS ensemble forecasts with lead times of between 8 and 16 hours. Comparison to the original cGAN trained on the UK using the IFS HRES forecast indicates that improved training forecasts result in improved post-processing. The cGAN models were additionally applied to the regions that they were not trained on. Each model performed well in their own region indicating that each model is somewhat region specific. However the model trained on the Washington DC, Atlantic coast, region achieved good scores across the USA and was competitive over the UK. There are more overall rainfall events spread over the whole region so the improved scores might be simply due to increased data. A model was therefore trained using data from all four regions which then outperformed the models trained locally.
更多查看译文
关键词
cgan,generative deep learning
AI 理解论文
溯源树
样例
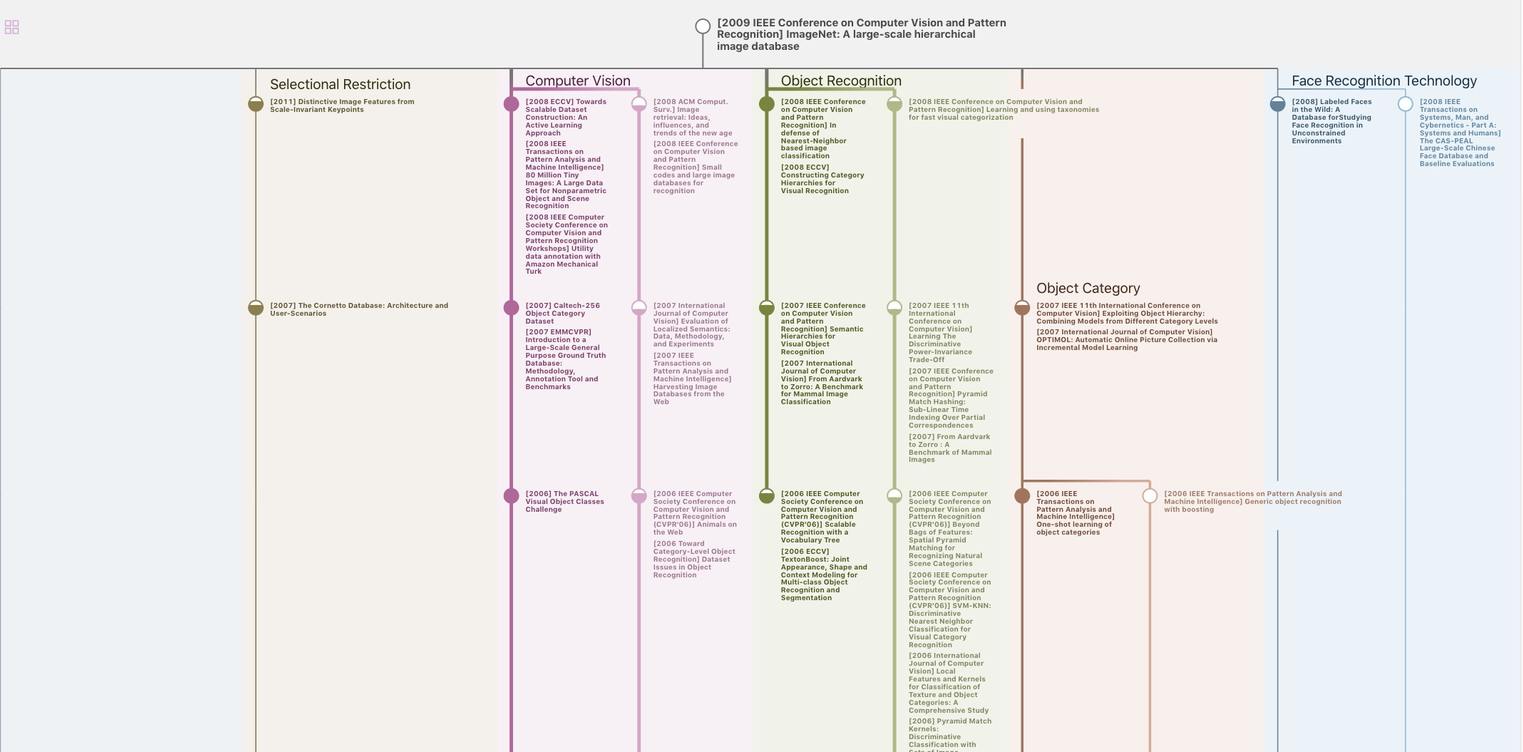
生成溯源树,研究论文发展脉络
Chat Paper
正在生成论文摘要