Startup success prediction and VC portfolio simulation using CrunchBase data
CoRR(2023)
摘要
Predicting startup success presents a formidable challenge due to the inherently volatile landscape of the entrepreneurial ecosystem. The advent of extensive databases like Crunchbase jointly with available open data enables the application of machine learning and artificial intelligence for more accurate predictive analytics. This paper focuses on startups at their Series B and Series C investment stages, aiming to predict key success milestones such as achieving an Initial Public Offering (IPO), attaining unicorn status, or executing a successful Merger and Acquisition (M\&A). We introduce novel deep learning model for predicting startup success, integrating a variety of factors such as funding metrics, founder features, industry category. A distinctive feature of our research is the use of a comprehensive backtesting algorithm designed to simulate the venture capital investment process. This simulation allows for a robust evaluation of our model's performance against historical data, providing actionable insights into its practical utility in real-world investment contexts. Evaluating our model on Crunchbase's, we achieved a 14 times capital growth and successfully identified on B round high-potential startups including Revolut, DigitalOcean, Klarna, Github and others. Our empirical findings illuminate the importance of incorporating diverse feature sets in enhancing the model's predictive accuracy. In summary, our work demonstrates the considerable promise of deep learning models and alternative unstructured data in predicting startup success and sets the stage for future advancements in this research area.
更多查看译文
关键词
vc portfolio simulation,startup success prediction,crunchbase
AI 理解论文
溯源树
样例
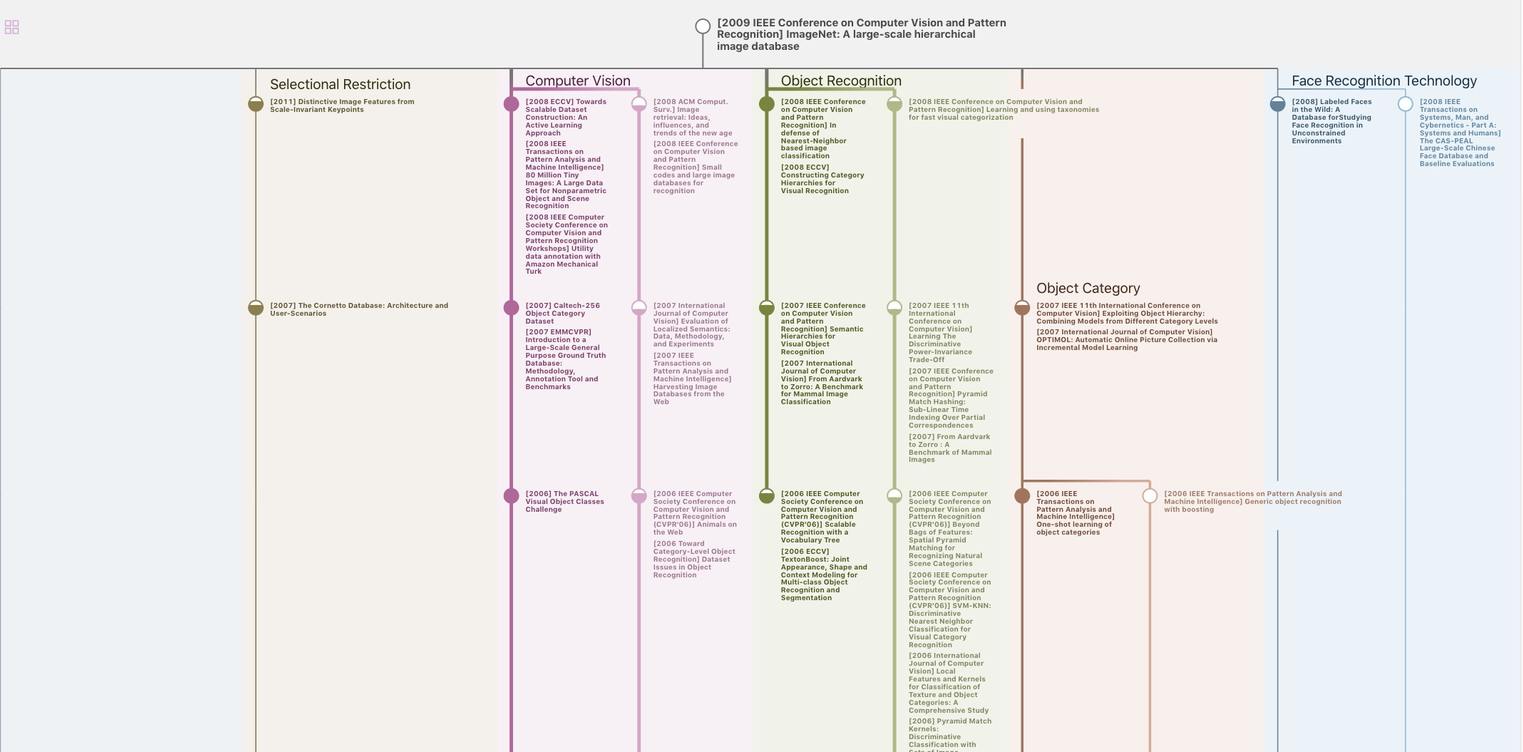
生成溯源树,研究论文发展脉络
Chat Paper
正在生成论文摘要