Low-rank Adaptation of Large Language Model Rescoring for Parameter-Efficient Speech Recognition
CoRR(2023)
摘要
We propose a neural language modeling system based on low-rank adaptation
(LoRA) for speech recognition output rescoring. Although pretrained language
models (LMs) like BERT have shown superior performance in second-pass
rescoring, the high computational cost of scaling up the pretraining stage and
adapting the pretrained models to specific domains limit their practical use in
rescoring. Here we present a method based on low-rank decomposition to train a
rescoring BERT model and adapt it to new domains using only a fraction (0.08
of the pretrained parameters. These inserted matrices are optimized through a
discriminative training objective along with a correlation-based regularization
loss. The proposed low-rank adaptation Rescore-BERT (LoRB) architecture is
evaluated on LibriSpeech and internal datasets with decreased training times by
factors between 5.4 and 3.6.
更多查看译文
关键词
large language model rescoring,adaptation,speech,low-rank,parameter-efficient
AI 理解论文
溯源树
样例
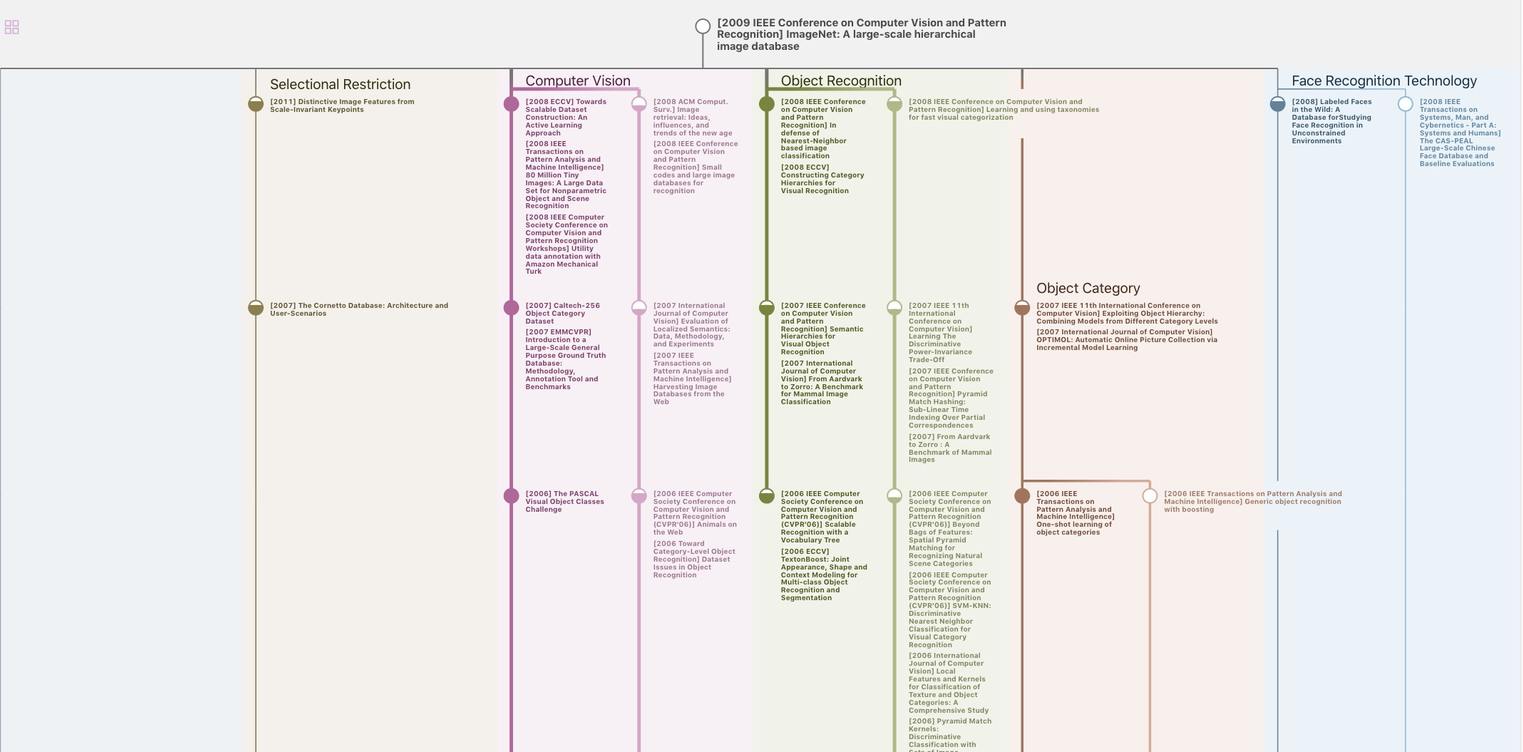
生成溯源树,研究论文发展脉络
Chat Paper
正在生成论文摘要