3D Reconstruction with Generalizable Neural Fields using Scene Priors
ICLR 2024(2023)
摘要
High-fidelity 3D scene reconstruction has been substantially advanced by recent progress in neural fields. However, most existing methods train a separate network from scratch for each individual scene. This is not scalable, inefficient, and unable to yield good results given limited views. While learning-based multi-view stereo methods alleviate this issue to some extent, their multi-view setting makes it less flexible to scale up and to broad applications. Instead, we introduce training generalizable Neural Fields incorporating scene Priors (NFPs). The NFP network maps any single-view RGB-D image into signed distance and radiance values. A complete scene can be reconstructed by merging individual frames in the volumetric space WITHOUT a fusion module, which provides better flexibility. The scene priors can be trained on large-scale datasets, allowing for fast adaptation to the reconstruction of a new scene with fewer views. NFP not only demonstrates SOTA scene reconstruction performance and efficiency, but it also supports single-image novel-view synthesis, which is underexplored in neural fields. More qualitative results are available at: https://oasisyang.github.io/neural-prior
更多查看译文
关键词
3D Reconstruction,Generalizable Neural Fields
AI 理解论文
溯源树
样例
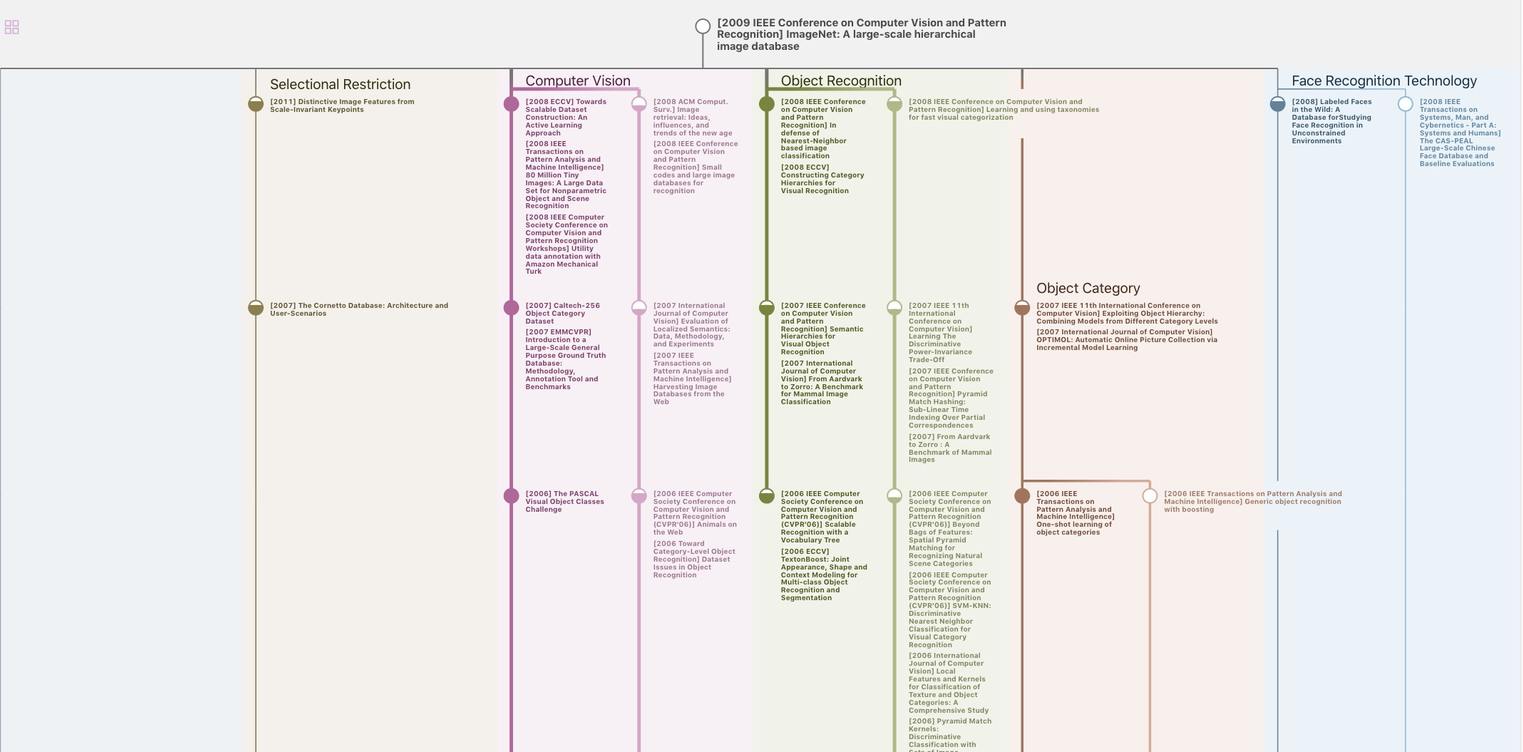
生成溯源树,研究论文发展脉络
Chat Paper
正在生成论文摘要