FireFace: Leveraging Internal Function Features for Configuration of Functions on Serverless Edge Platforms
Sensors(2023)
摘要
The emerging serverless computing has become a captivating paradigm for deploying cloud applications, alleviating developers' concerns about infrastructure resource management by configuring necessary parameters such as latency and memory constraints. Existing resource configuration solutions for cloud-based serverless applications can be broadly classified into modeling based on historical data or a combination of sparse measurements and interpolation/modeling. In pursuit of service response and conserving network bandwidth, platforms have progressively expanded from the traditional cloud to the edge. Compared to cloud platforms, serverless edge platforms often lead to more running overhead due to their limited resources, resulting in undesirable financial costs for developers when using the existing solutions. Meanwhile, it is extremely challenging to handle the heterogeneity of edge platforms, characterized by distinct pricing owing to their varying resource preferences. To tackle these challenges, we propose an adaptive and efficient approach called FireFace, consisting of prediction and decision modules. The prediction module extracts the internal features of all functions within the serverless application and uses this information to predict the execution time of the functions under specific configuration schemes. Based on the prediction module, the decision module analyzes the environment information and uses the Adaptive Particle Swarm Optimization algorithm and Genetic Algorithm Operator (APSO-GA) algorithm to select the most suitable configuration plan for each function, including CPU, memory, and edge platforms. In this way, it is possible to effectively minimize the financial overhead while fulfilling the Service Level Objectives (SLOs). Extensive experimental results show that our prediction model obtains optimal results under all three metrics, and the prediction error rate for real-world serverless applications is in the range of 4.25 similar to 9.51%. Our approach can find the optimal resource configuration scheme for each application, which saves 7.2 similar to 44.8% on average compared to other classic algorithms. Moreover, FireFace exhibits rapid adaptability, efficiently adjusting resource allocation schemes in response to dynamic environments.
更多查看译文
关键词
serverless computing,function as a service,configuration optimization,SLO
AI 理解论文
溯源树
样例
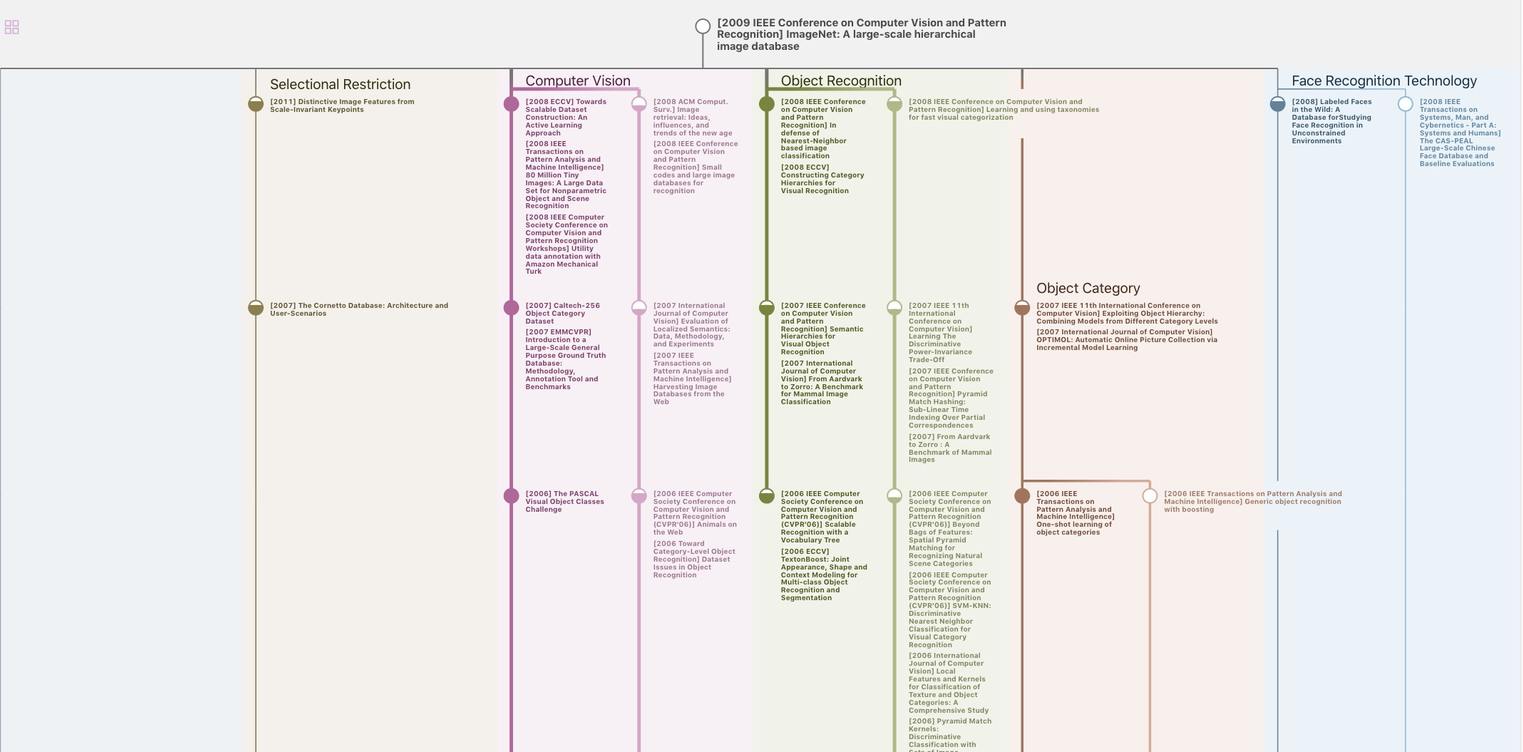
生成溯源树,研究论文发展脉络
Chat Paper
正在生成论文摘要