SIM-CNN: Self-supervised Individualized Multimodal Learning for Stress Prediction on Nurses Using Biosignals
MACHINE LEARNING FOR MULTIMODAL HEALTHCARE DATA, ML4MHD 2023(2024)
摘要
Precise stress recognition from biosignals is inherently challenging due to the heterogeneous nature of stress, individual physiological differences, and scarcity of labeled data. To address these issues, we developed SIM-CNN, a self-supervised learning (SSL) method for personalized stress-recognition models using multimodal biosignals. SIM-CNN involves training a multimodal 1D convolutional neural network (CNN) that leverages SSL to utilize massive unlabeled data, optimizing individual parameters and hyperparameters for precision health. SIM-CNN is evaluated on a real-world multimodal dataset collected from nurses that consists of 1,250 h of biosignals, 83 h of which are explicitly labeled with stress levels. SIM-CNN is pre-trained on the unlabeled biosignal data with next-step time series forecasting and fine-tuned on the labeled data for stress classification. Compared to SVMs and baseline CNNs with an identical architecture but without self-supervised pre-training, SIM-CNN shows clear improvements in the average AUC and accuracy, but a further examination of the data also suggests some intrinsic limitations of patient-specific stress recognition using biosignals recorded in the wild.
更多查看译文
关键词
Self-Supervised learning,Multimodality,Precision health,Stress,Biosignals
AI 理解论文
溯源树
样例
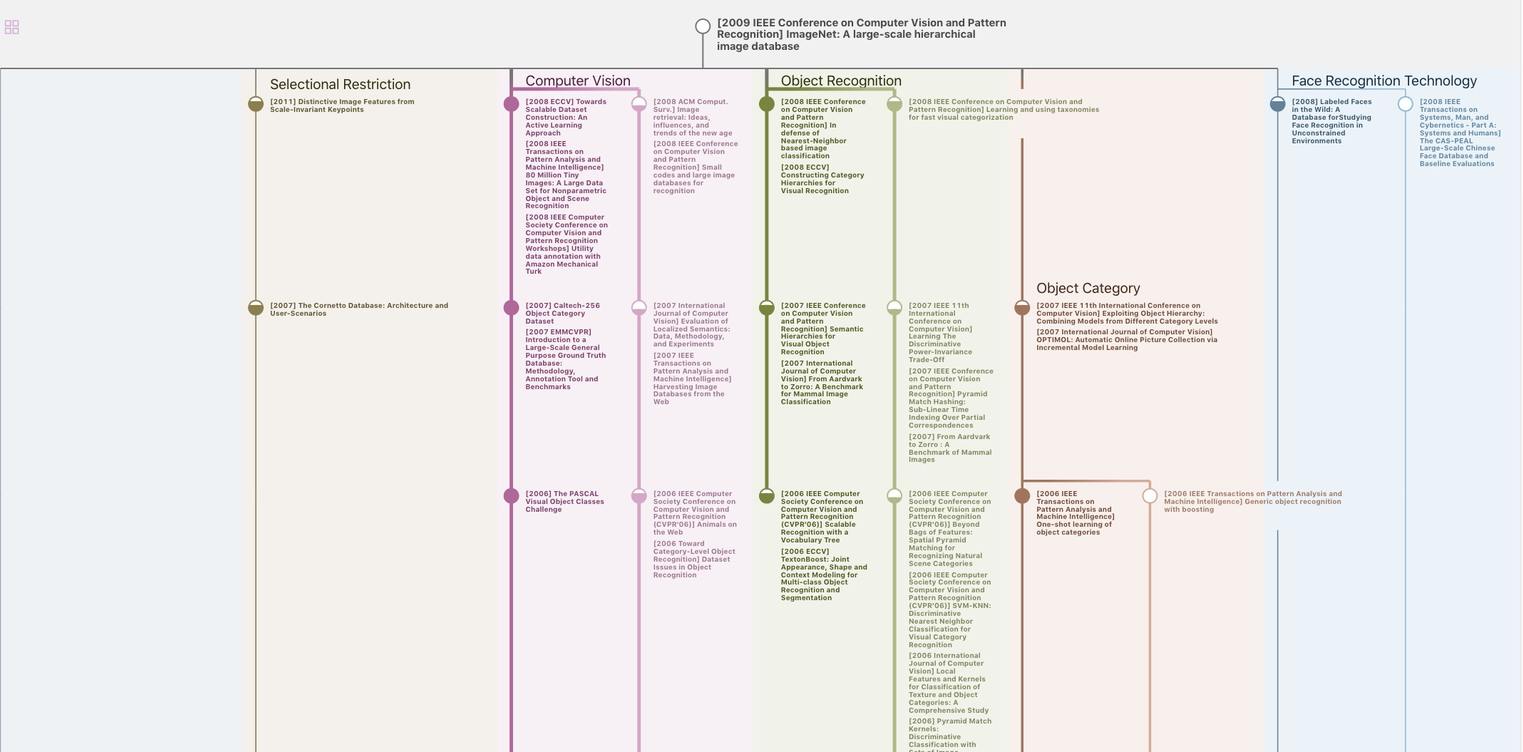
生成溯源树,研究论文发展脉络
Chat Paper
正在生成论文摘要