Optimal Stopping with Multi-Dimensional Comparative Loss Aversion
CoRR(2023)
摘要
Despite having the same basic prophet inequality setup and model of loss aversion, conclusions in our multi-dimensional model differs considerably from the one-dimensional model of Kleinberg et al. For example, Kleinberg et al. gives a tight closed-form on the competitive ratio that an online decision-maker can achieve as a function of $\lambda$, for any $\lambda \geq 0$. In our multi-dimensional model, there is a sharp phase transition: if $k$ denotes the number of dimensions, then when $\lambda \cdot (k-1) \geq 1$, no non-trivial competitive ratio is possible. On the other hand, when $\lambda \cdot (k-1) < 1$, we give a tight bound on the achievable competitive ratio (similar to Kleinberg et al.). As another example, Kleinberg et al. uncovers an exponential improvement in their competitive ratio for the random-order vs. worst-case prophet inequality problem. In our model with $k\geq 2$ dimensions, the gap is at most a constant-factor. We uncover several additional key differences in the multi- and single-dimensional models.
更多查看译文
关键词
optimal stopping,loss,multi-dimensional
AI 理解论文
溯源树
样例
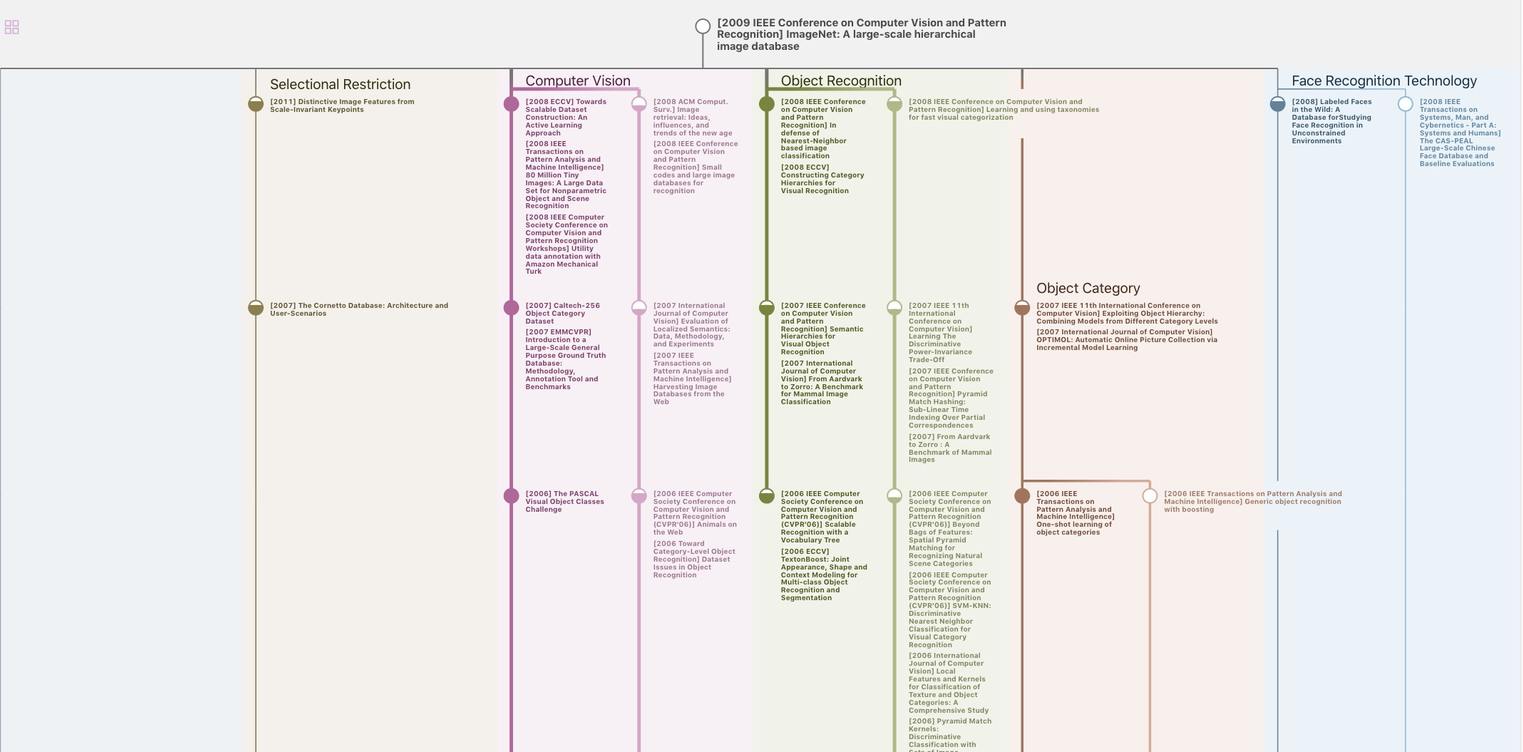
生成溯源树,研究论文发展脉络
Chat Paper
正在生成论文摘要