Zeroth-order Riemannian Averaging Stochastic Approximation Algorithms
CoRR(2023)
摘要
We present Zeroth-order Riemannian Averaging Stochastic Approximation (\texttt{Zo-RASA}) algorithms for stochastic optimization on Riemannian manifolds. We show that \texttt{Zo-RASA} achieves optimal sample complexities for generating $\epsilon$-approximation first-order stationary solutions using only one-sample or constant-order batches in each iteration. Our approach employs Riemannian moving-average stochastic gradient estimators, and a novel Riemannian-Lyapunov analysis technique for convergence analysis. We improve the algorithm's practicality by using retractions and vector transport, instead of exponential mappings and parallel transports, thereby reducing per-iteration complexity. Additionally, we introduce a novel geometric condition, satisfied by manifolds with bounded second fundamental form, which enables new error bounds for approximating parallel transport with vector transport.
更多查看译文
AI 理解论文
溯源树
样例
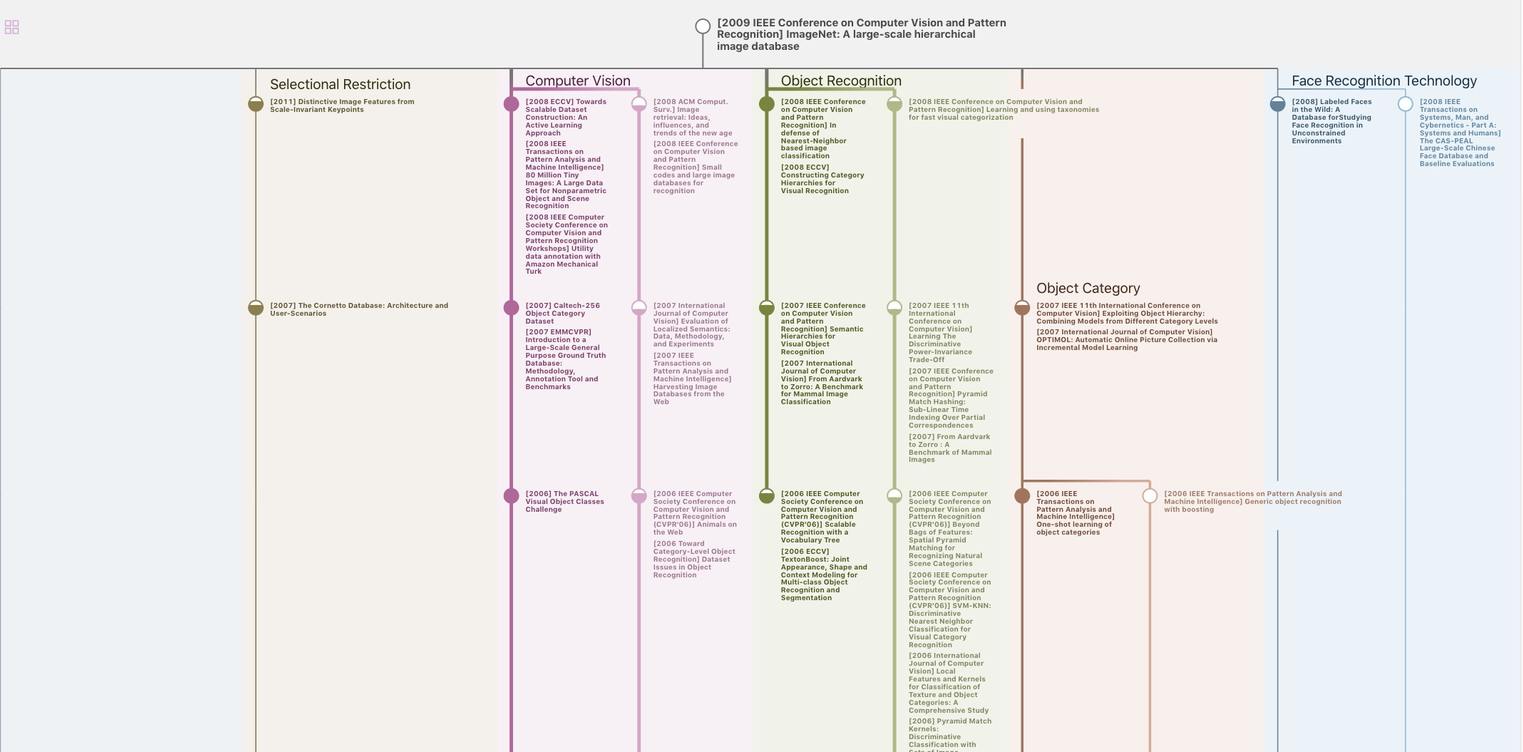
生成溯源树,研究论文发展脉络
Chat Paper
正在生成论文摘要