Intelligent Sampling: A Novel Approach to Optimize Workload Scheduling in Large-Scale Heterogeneous Computing Continuum
2023 IEEE International Conference on Service-Oriented System Engineering (SOSE)(2023)
摘要
Scheduling workloads on large-scale infrastructures, such as in the Edge-Cloud continuum is a challenging task. Usually, the scheduling algorithm considers only a limited sample of the infrastructure nodes, typically obtained through random sampling. The sampling reduces the number of nodes, which need to be evaluated in the scheduling pipeline, making the scheduling process more saleable. Unfortunately, current sampling approaches become largely inefficient when the infrastructure is heterogeneous and specific, scarce node characteristics are required to successfully execute a workload. Computing continuum infrastructures are heterogeneous, hence, we need to re-think the sampling process to keep it viable at scale while also being able to identify and leverage the heterogeneity of the Edge-Cloud continuum resources. In this article, we present Intelligent Sampling - a novel technique for improving sampling in large-scale and heterogeneous infrastructures. We develop a model for any heterogeneous infrastructure. Based on this model, we provide a method to sample the infrastructure nodes more accurately, considering the specific task at hand. Finally, we leverage the Alibaba PAI dataset to show that our approach is 2.5x times more accurate compared with other state-of-the-art sampling mechanisms while retaining comparable performance and scalability.
更多查看译文
关键词
Computing continuum,Intelligent sampling,Workloads scheduling,Heterogeneous infrastructure model
AI 理解论文
溯源树
样例
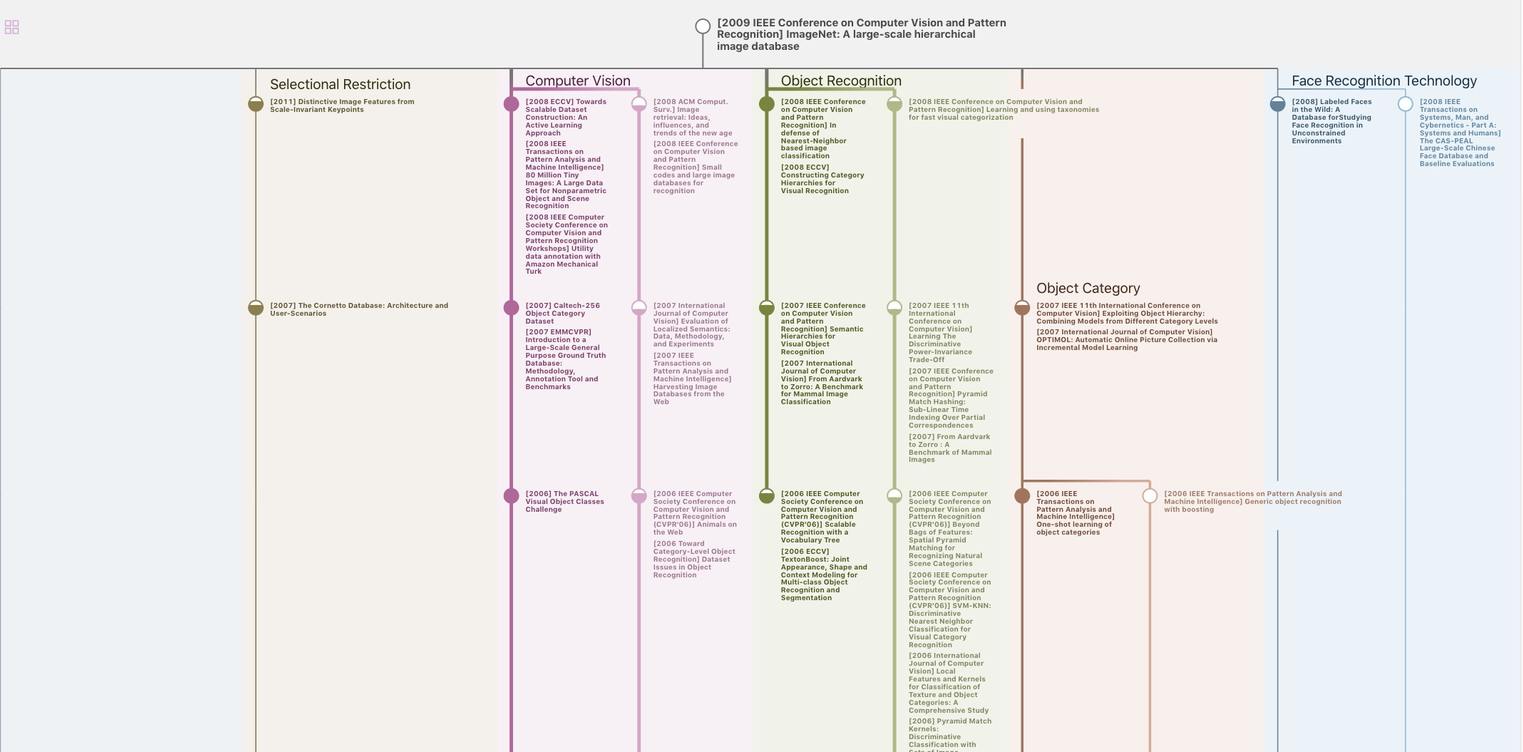
生成溯源树,研究论文发展脉络
Chat Paper
正在生成论文摘要