Stackelberg Driver Model for Continual Policy Improvement in Scenario-Based Closed-Loop Autonomous Driving
CoRR(2023)
摘要
The deployment of autonomous vehicles (AVs) has faced hurdles due to the dominance of rare but critical corner cases within the long-tail distribution of driving scenarios, which negatively affects their overall performance. To address this challenge, adversarial generation methods have emerged as a class of efficient approaches to synthesize safety-critical scenarios for AV testing. However, these generated scenarios are often underutilized for AV training, resulting in the potential for continual AV policy improvement remaining untapped, along with a deficiency in the closed-loop design needed to achieve it. Therefore, we tailor the Stackelberg Driver Model (SDM) to accurately characterize the hierarchical nature of vehicle interaction dynamics, facilitating iterative improvement by engaging background vehicles (BVs) and AV in a sequential game-like interaction paradigm. With AV acting as the leader and BVs as followers, this leader-follower modeling ensures that AV would consistently refine its policy, always taking into account the additional information that BVs play the best response to challenge AV. Extensive experiments have shown that our algorithm exhibits superior performance compared to several baselines especially in higher dimensional scenarios, leading to substantial advancements in AV capabilities while continually generating progressively challenging scenarios.
更多查看译文
关键词
continual policy improvement,driver,scenario-based,closed-loop
AI 理解论文
溯源树
样例
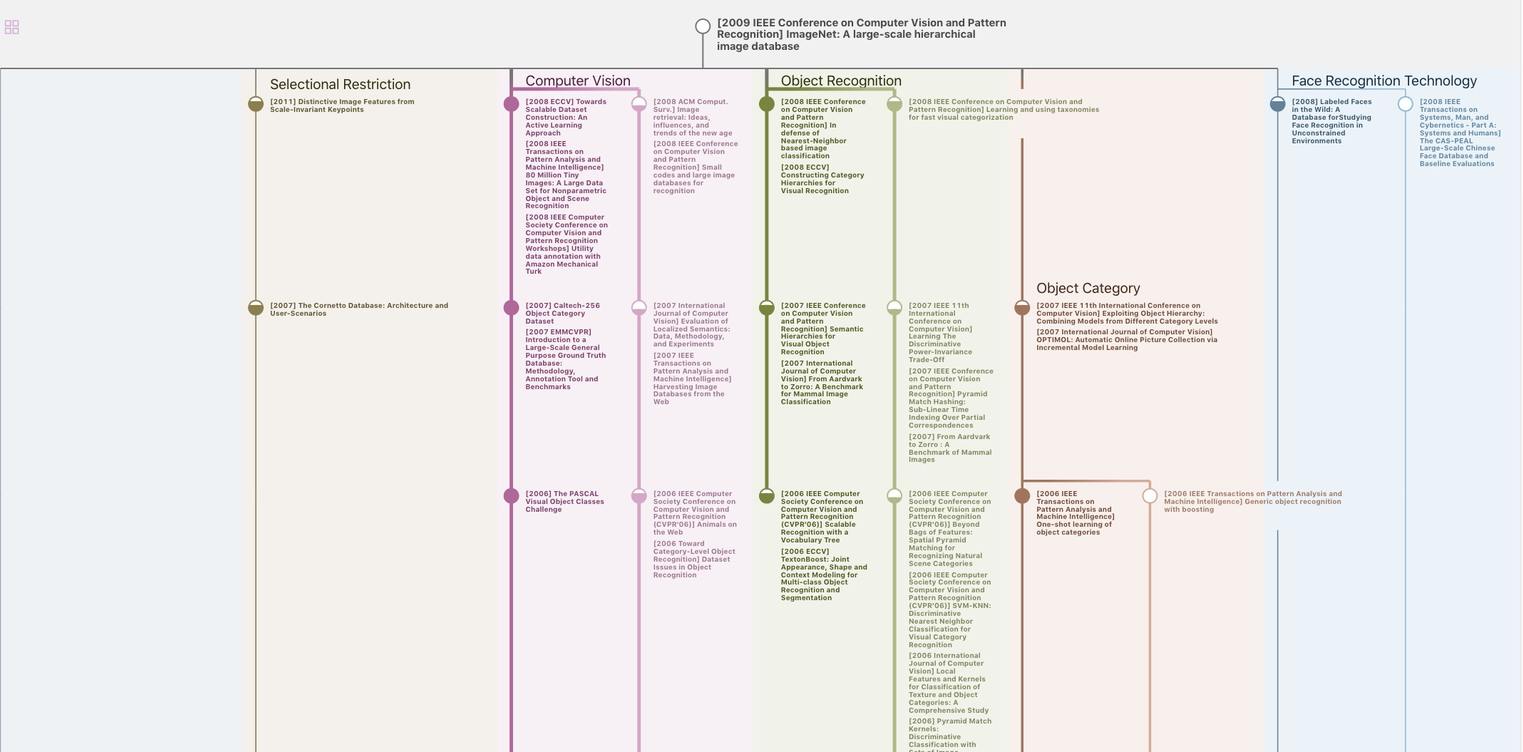
生成溯源树,研究论文发展脉络
Chat Paper
正在生成论文摘要