Of Representation Theory and Quantum Approximate Optimization Algorithm
arXiv (Cornell University)(2023)
摘要
In this paper, the Quantum Approximate Optimization Algorithm (QAOA) is analyzed by leveraging symmetries inherent in problem Hamiltonians. We focus on the generalized formulation of optimization problems defined on the sets of $n$-element $d$-ary strings. Our main contribution encompasses dimension reductions for the originally proposed QAOA. These reductions retain the same problem Hamiltonian as the original QAOA but differ in terms of their mixer Hamiltonian, and initial state. The vast QAOA space has a daunting dimension of exponential scaling in $n$, where certain reduced QAOA spaces exhibit dimensions governed by polynomial functions. This phenomenon is illustrated in this paper, by providing partitions corresponding to polynomial dimensions in the corresponding subspaces. As a result, each reduced QAOA partition encapsulates unique classical solutions absent in others, allowing us to establish a lower bound on the number of solutions to the initial optimization problem. Our novel approach opens promising practical advantages in accelerating the class of QAOA approaches, both quantum-based and classical simulation of circuits, as well as a potential tool to cope with barren plateaus problem.
更多查看译文
关键词
quantum approximate optimization algorithm,representation theory
AI 理解论文
溯源树
样例
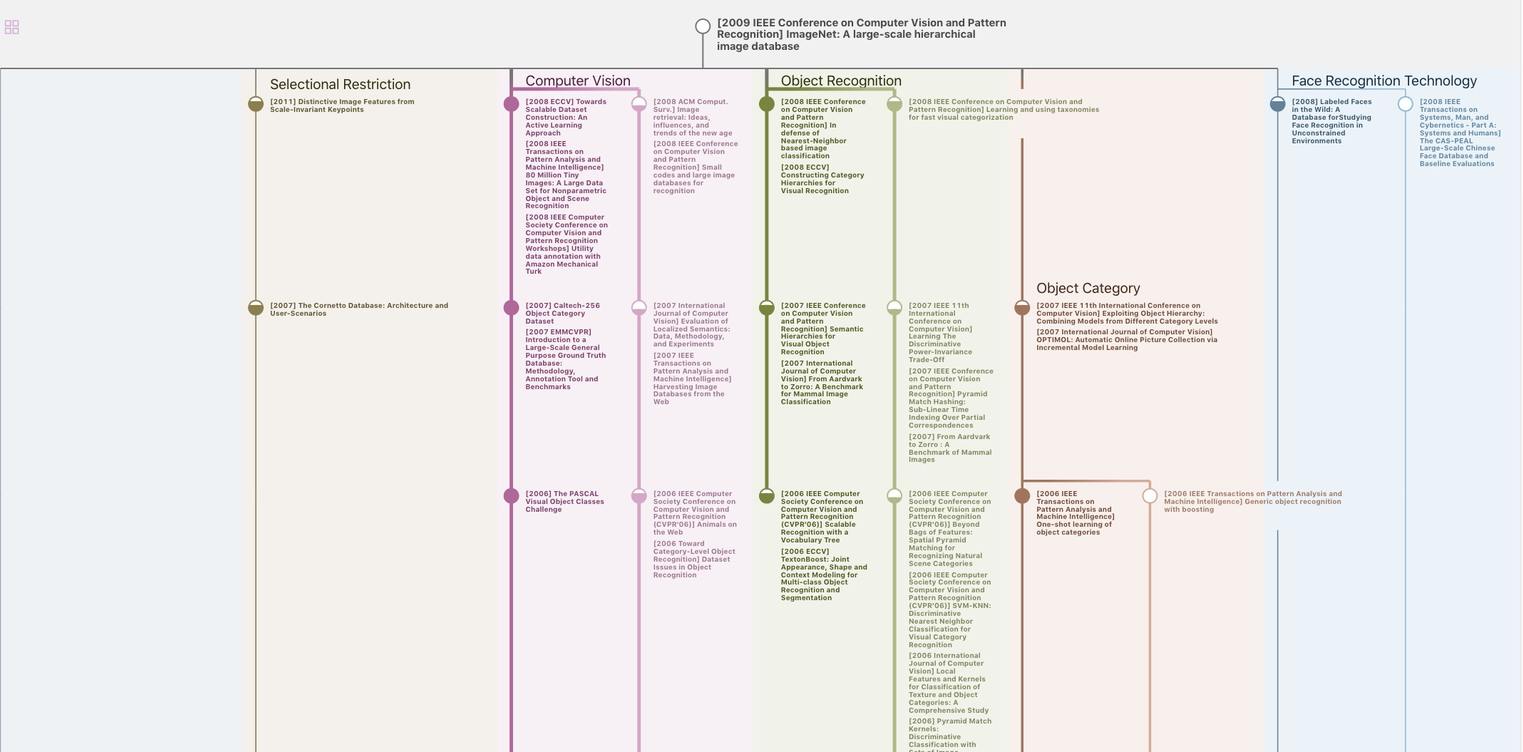
生成溯源树,研究论文发展脉络
Chat Paper
正在生成论文摘要