Reinforcement Learning for Robust Header Compression under Model Uncertainty
arXiv (Cornell University)(2023)
摘要
Robust header compression (ROHC), critically positioned between the network and the MAC layers, plays an important role in modern wireless communication systems for improving data efficiency. This work investigates bi-directional ROHC (BD-ROHC) integrated with a novel architecture of reinforcement learning (RL). We formulate a partially observable \emph{Markov} decision process (POMDP), in which agent is the compressor, and the environment consists of the decompressor, channel and header source. Our work adopts the well-known deep Q-network (DQN), which takes the history of actions and observations as inputs, and outputs the Q-values of corresponding actions. Compared with the ideal dynamic programming (DP) proposed in the existing works, our method is scalable to the state, action and observation spaces. In contrast, DP often suffers from formidable computational complexity when the number of states becomes large due to long decompressor feedback delay and complex channel models. In addition, our method does not require prior knowledge of the transition dynamics and accurate observation dependency of the model, which are often not available in many practical applications.
更多查看译文
关键词
robust header compression,reinforcement learning,uncertainty
AI 理解论文
溯源树
样例
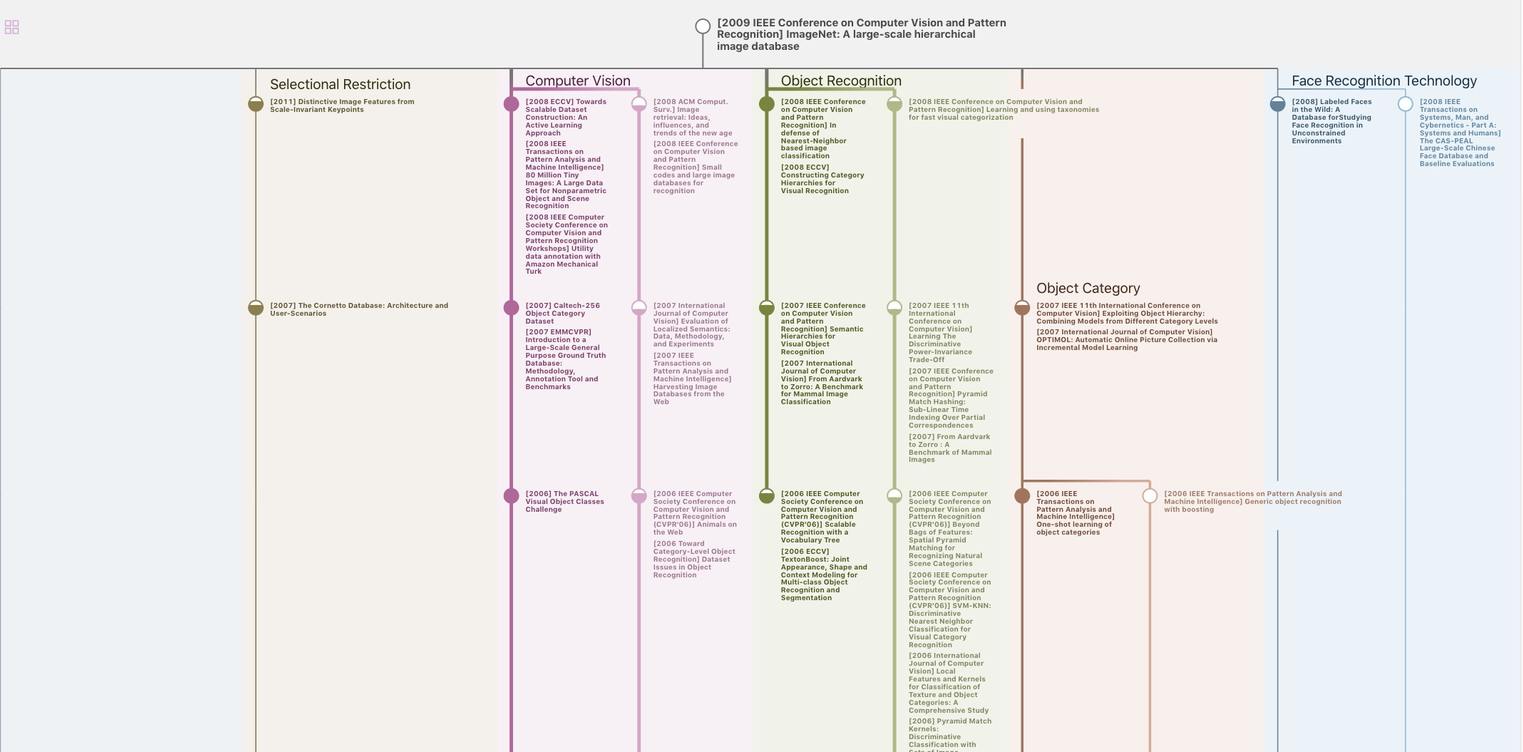
生成溯源树,研究论文发展脉络
Chat Paper
正在生成论文摘要