Zeroth-Order Federated Methods for Stochastic MPECs and Nondifferentiable Nonconvex Hierarchical Optimization
arxiv(2023)
摘要
Motivated by the emergence of federated learning (FL), we design and analyze
federated methods for addressing: (i) Nondifferentiable nonconvex optimization;
(ii) Bilevel optimization; (iii) Minimax problems; and (iv) Two-stage
stochastic mathematical programs with equilibrium constraints (2s-SMPEC).
Research on these problems has been limited and afflicted by reliance on strong
assumptions, including the need for differentiability of the implicit function
and the absence of constraints in the lower-level problem, among others. We
make the following contributions. In (i), by leveraging convolution-based
smoothing and Clarke's subdifferential calculus, we devise a randomized
smoothing-enabled zeroth-order FL method and derive communication and iteration
complexity guarantees for computing an approximate Clarke stationary point. To
contend with (ii) and (iii), we devise a unifying randomized implicit
zeroth-order FL framework, equipped with explicit communication and iteration
complexities. Importantly, our method utilizes delays during local steps to
skip calls to the inexact lower-level FL oracle. This results in significant
reduction in communication overhead. In (iv), we devise an inexact implicit
variant of the method in (i). Remarkably, this method achieves a total
communication complexity matching that of single-level nonsmooth nonconvex
optimization in FL. We empirically validate the theoretical findings on
instances of federated nonsmooth and hierarchical problems.
更多查看译文
AI 理解论文
溯源树
样例
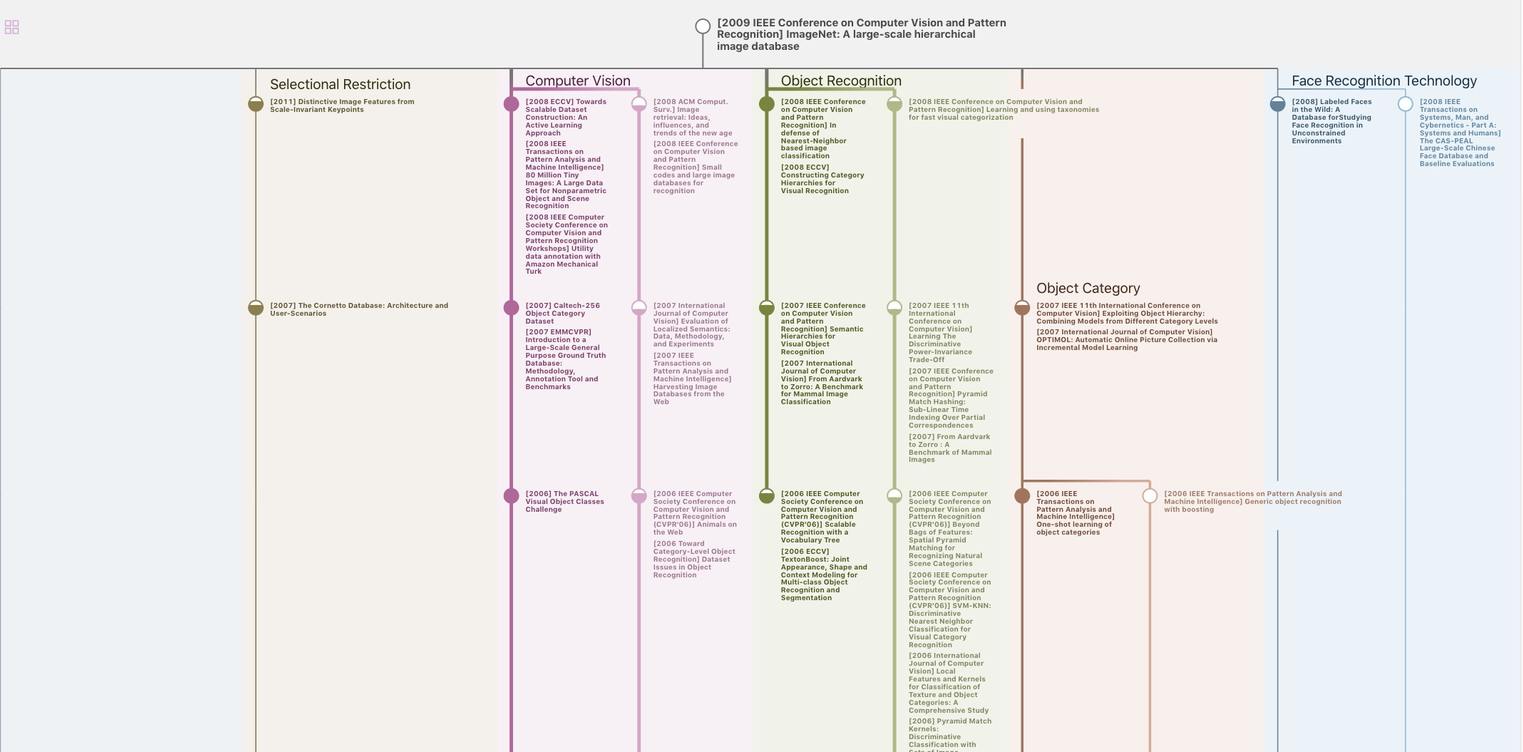
生成溯源树,研究论文发展脉络
Chat Paper
正在生成论文摘要