Distributionally Robust Model Predictive Control: Closed-loop Guarantees and Scalable Algorithms
CoRR(2023)
摘要
We establish a collection of closed-loop guarantees and propose a scalable, Newton-type optimization algorithm for distributionally robust model predictive control (DRMPC) applied to linear systems, zero-mean disturbances, convex constraints, and quadratic costs. Via standard assumptions for the terminal cost and constraint, we establish distribtionally robust long-term and stage-wise performance guarantees for the closed-loop system. We further demonstrate that a common choice of the terminal cost, i.e., as the solution to the discrete-algebraic Riccati equation, renders the origin input-to-state stable for the closed-loop system. This choice of the terminal cost also ensures that the exact long-term performance of the closed-loop system is independent of the choice of ambiguity set the for DRMPC formulation. Thus, we establish conditions under which DRMPC does not provide a long-term performance benefit relative to stochastic MPC (SMPC). To solve the proposed DRMPC optimization problem, we propose a Newton-type algorithm that empirically achieves superlinear convergence by solving a quadratic program at each iteration and guarantees the feasibility of each iterate. We demonstrate the implications of the closed-loop guarantees and the scalability of the proposed algorithm via two examples.
更多查看译文
关键词
control,predictive,closed-loop
AI 理解论文
溯源树
样例
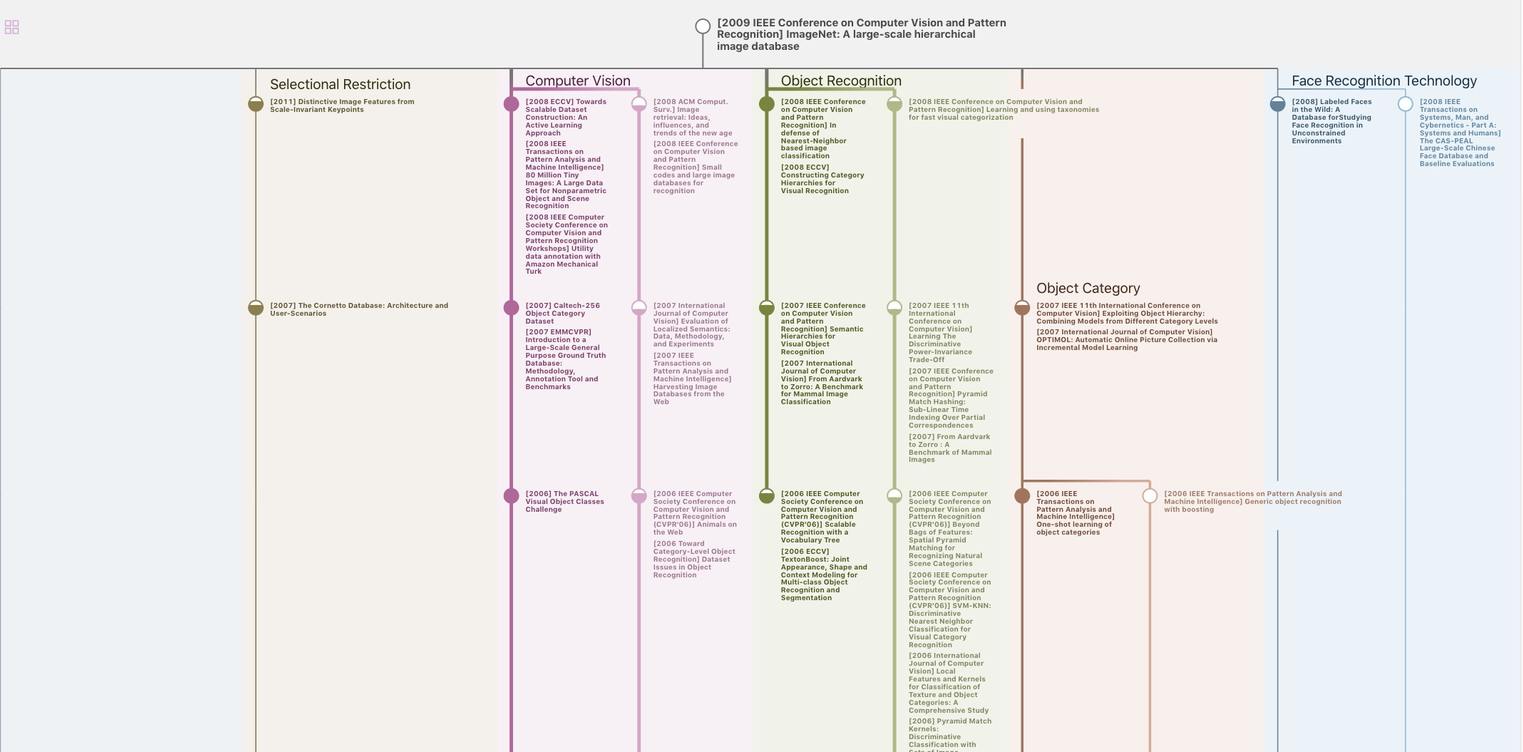
生成溯源树,研究论文发展脉络
Chat Paper
正在生成论文摘要