Dual selective knowledge transfer for few-shot classification
Applied Intelligence(2023)
摘要
Few-shot learning aims at recognizing novel visual categories from very few labelled examples. Different from the existing few-shot classification methods that are mainly based on metric learning or meta-learning, in this work we focus on improving the representation capacity of feature extractors. For this purpose, we propose a new two-stage dual selective knowledge transfer (DSKT) framework, to guide models towards better optimization. Specifically, we first exploit an improved multi-task learning approach to train a feature extractor with robust representation capability as a teacher model. Then, we design an effective dual selective knowledge distillation method, which enables the student model to selectively learn knowledge from the teacher model and current samples, thereby improving the student model’s ability to generalize on unseen classes. Extensive experimental results show that our DSKT achieves competitive performances on four well-known few-shot classification benchmarks.
更多查看译文
关键词
Knowledge transfer,Few-shot classification,Feature extractor
AI 理解论文
溯源树
样例
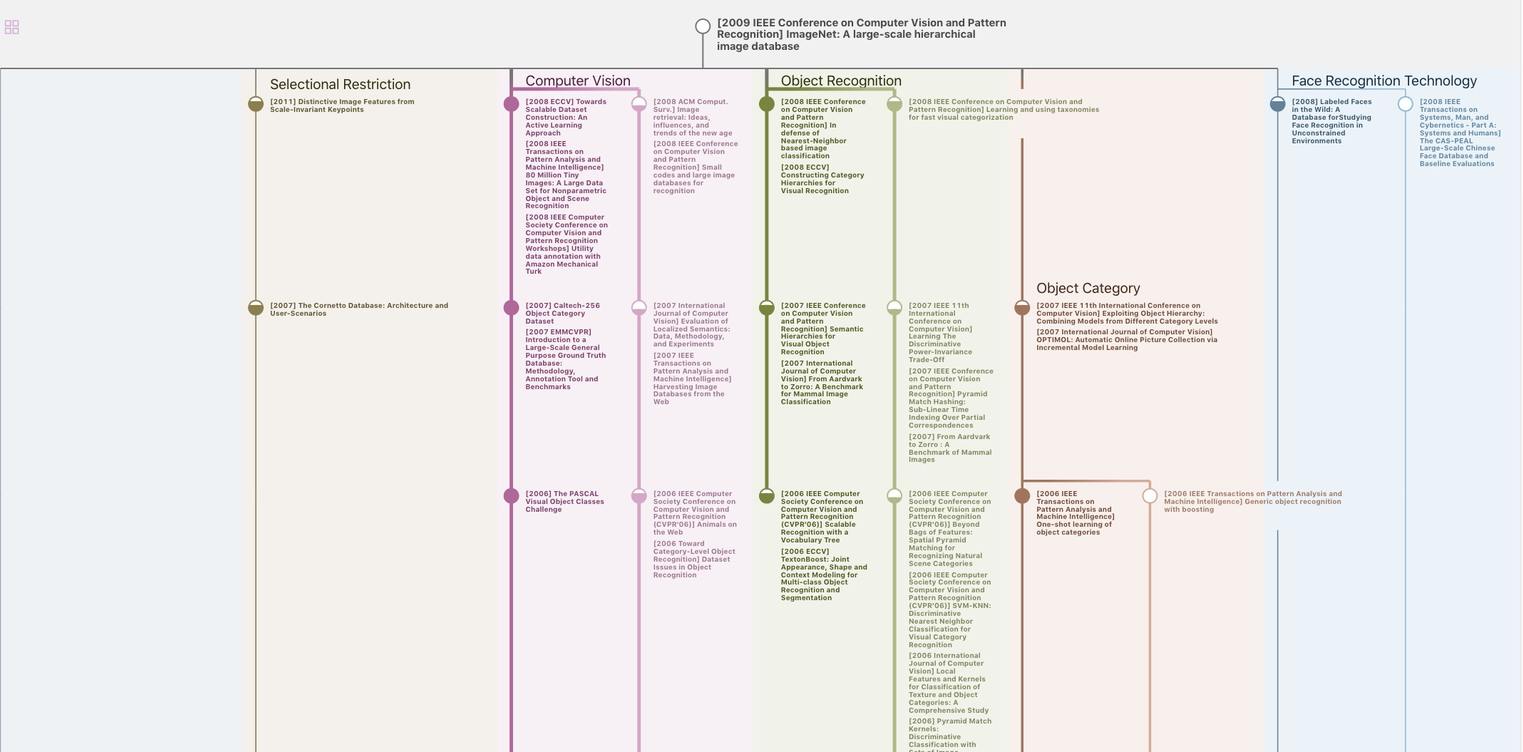
生成溯源树,研究论文发展脉络
Chat Paper
正在生成论文摘要