Decentralized Configuration of TSCH-Based IoT Networks for Distinctive QoS: A Deep Reinforcement Learning Approach
IEEE Internet of Things Journal(2023)
摘要
The IEEE 802.15.4 time-slotted channel hopping (TSCH) is widely used as a reliable, low-power, and low-cost communication technology for many industrial Internet of Things (IoT) networks. In many applications, Quality-of-Service (QoS) requirements are different for heterogeneous nodes, necessitating nonequal parameter settings per node. This results in a very large configuration space making space exploration complex and time consuming. Moreover, network state and QoS requirements may change over time. Thus, run-time configuration mechanisms are needed for making decisions about proper node settings to consistently satisfy diverse and dynamic QoS requirements. In this article, we propose a run-time decentralized self-optimization framework based on deep reinforcement learning (DRL) for parameter configuration of a multihop TSCH network. DRL adopts neural networks as approximate functions to speed up the process of converging to QoS-satisfying configurations. Simulation results show that our proposed framework enables the network to use the right configuration settings according to the diverse QoS demands of different nodes. Moreover, it is shown that the convergence time of the learning framework is in the order of a few minutes which is acceptable for many IoT applications.
更多查看译文
关键词
Deep reinforcement learning (DRL),IEEE 802.15.4 time-slotted channel hopping (TSCH),Internet of Things (IoT),Quality-of-Service (QoS),wireless sensor network (WSN)
AI 理解论文
溯源树
样例
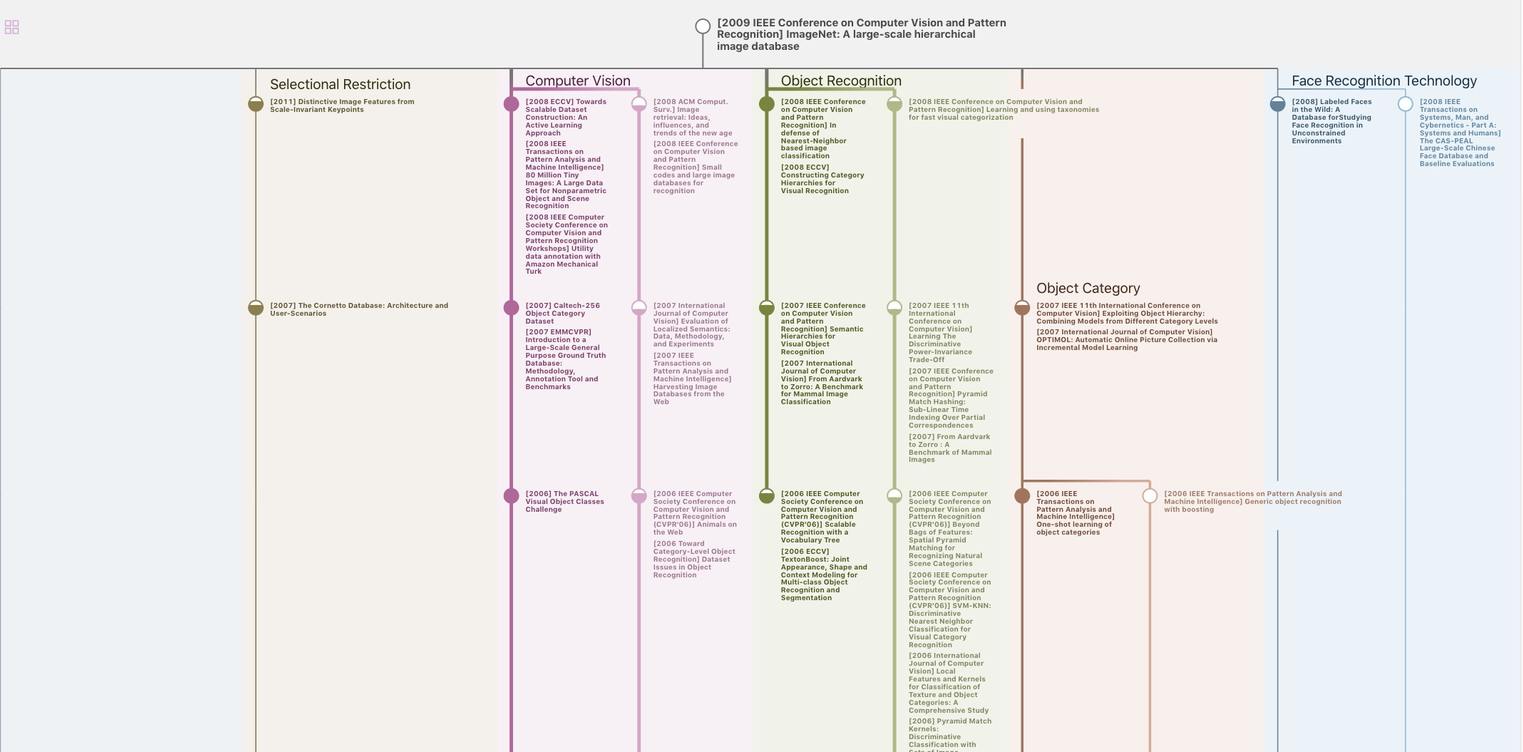
生成溯源树,研究论文发展脉络
Chat Paper
正在生成论文摘要