Deep Learning Estimation of 10-2 Visual Field Map Based on Macular Optical Coherence Tomography Angiography Measurements
AMERICAN JOURNAL OF OPHTHALMOLOGY(2024)
摘要
center dot PURPOSE: To develop deep learning (DL) models estimating the central visual field (VF) from optical coherence tomography angiography (OCTA) vessel density center dot DESIGN: Development and validation of a deep learning model. center dot METHODS: A total of 1051 10-2 VF OCTA pairs from healthy, glaucoma suspects, and glaucoma eyes were included. DL models were trained on en face macula VD images from OCTA to estimate 10-2 mean deviation (MD), pattern standard deviation (PSD), 68 total deviation (TD) and pattern deviation (PD) values and compared with a linear regression (LR) model with the same input. Accuracy of the models was evaluated by calculating the average mean absolute error (MAE) and the R 2 (squared Pearson correlation coefficients) of the estimated and actual VF values. center dot RESULTS: DL models predicting 10-2 MD achieved R 2 of 0.85 (95% confidence interval [CI], 74-0.92) for 102 MD and MAEs of 1.76 dB (95% CI, 1.39-2.17 dB) for MD. This was significantly better than mean linear estimates for 10-2 MD. The DL model outperformed the LR model for the estimation of pointwise TD values with an average MAE of 2.48 dB (95% CI, 1.99-3.02) and R 2 of 0.69 (95% CI, 0.57-0.76) over all test points. The DL model outperformed the LR model for the estimation of all sectors. center dot CONCLUSIONS: DL models enable the estimation of VF loss from OCTA images with high accuracy. Applying DL to the OCTA images may enhance clinical decision mak- ing. It also may improve individualized patient care and risk stratification of patients who are at risk for central VF damage. (Am J Ophthalmol 2024;257: 187-200. (c) 2023 Elsevier Inc. All rights reserved.)
更多查看译文
AI 理解论文
溯源树
样例
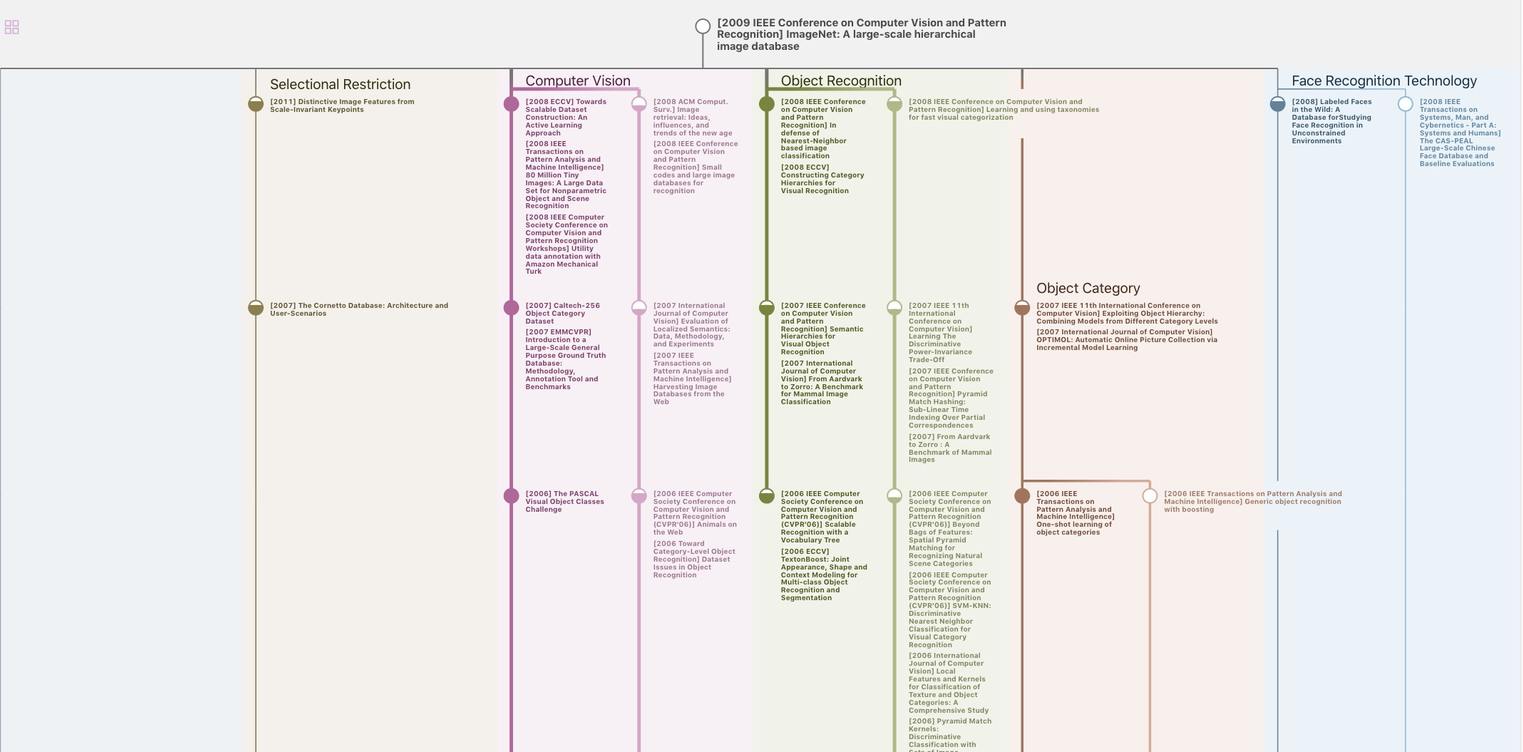
生成溯源树,研究论文发展脉络
Chat Paper
正在生成论文摘要