Grey Wolf Optimization Algorithm Based on Follow-Controlled Learning Strategy
IEEE Access(2023)
摘要
This paper analyzes the OBL strategy’s impact on optimizing the GWO algorithm and identifies three shortcomings. the specific limitations of the OBL optimization approach. To address these three shortcomings and enhance both global optimization and local exploration capabilities of GWO, this paper introduces a follow-controlled opposition learning strategy. then, the paper analyzes the control parameter C of the grey wolf algorithm to investigate its impact on global optimization and local exploration. Based on these properties, a new control parameter C is proposed. The proposed learning strategy and control parameter C are introduced into the traditional grey wolf algorithm to obtain the FCGWO algorithm. Finally, this paper conducts a comparative analysis of the FCGWO algorithm in comparison to other meta-heuristic algorithms, as well as the enhanced grey wolf algorithm, utilizing 23 benchmark test functions and 2 engineering problems. The results indicate that FCGWO effectively avoids the shortcomings of the traditional OBL, while also outperforming other algorithms significantly in terms of solution quality.
更多查看译文
关键词
Statistics, Sociology, Optimization, Behavioral sciences, Convergence, Particle swarm optimization, Metaheuristics, Meta-heuristic algorithm, grey wolf optimizer, opposition-based learning, optimization
AI 理解论文
溯源树
样例
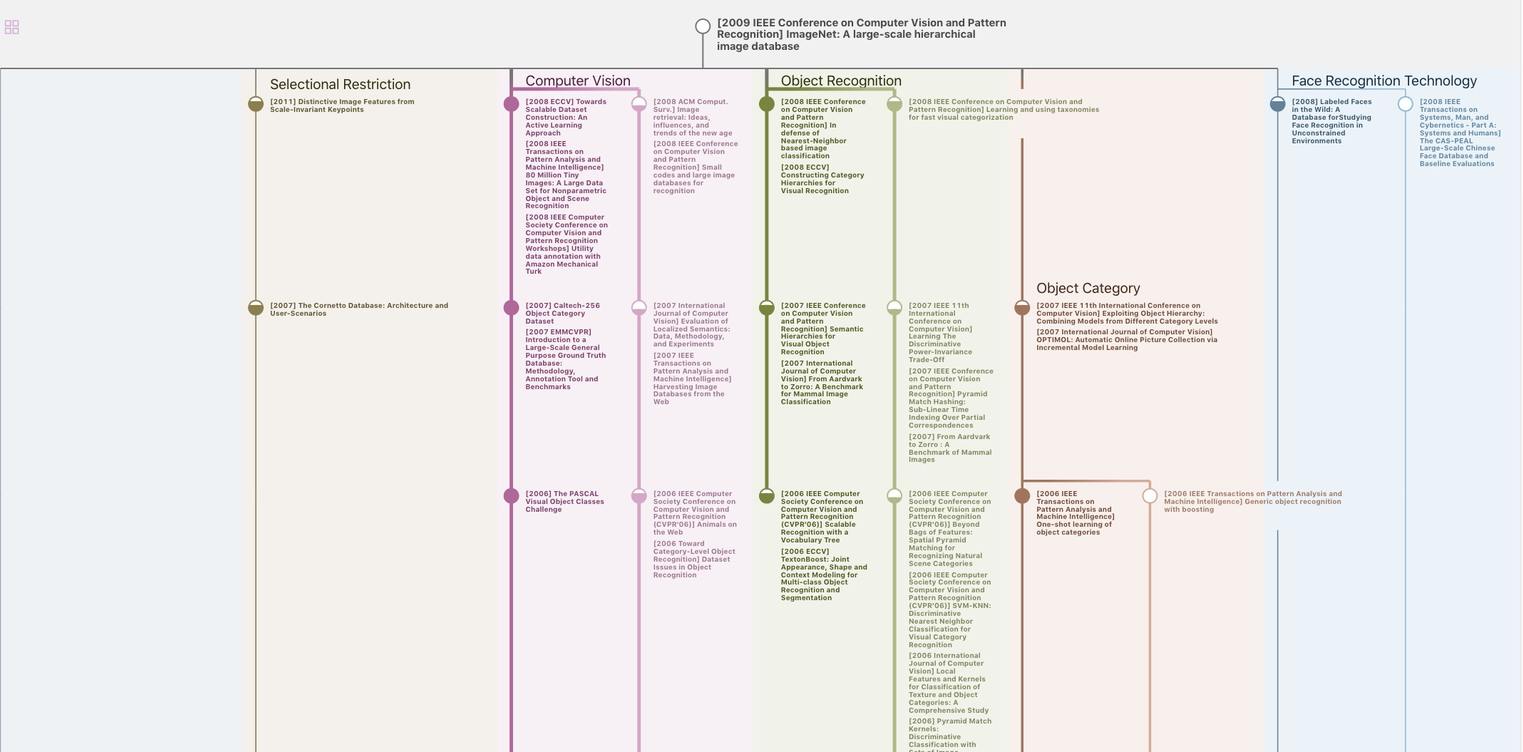
生成溯源树,研究论文发展脉络
Chat Paper
正在生成论文摘要