SupeRBNN: Randomized Binary Neural Network Using Adiabatic Superconductor Josephson Devices
56TH IEEE/ACM INTERNATIONAL SYMPOSIUM ON MICROARCHITECTURE, MICRO 2023(2023)
摘要
Adiabatic Quantum-Flux-Parametron (AQFP) is a superconducting logic with extremely high energy efficiency. By employing the distinct polarity of current to denote logic '0' and '1', AQFP devices serve as excellent carriers for binary neural network (BNN) computations. Although recent research has made initial strides toward developing an AQFP-based BNN accelerator, several critical challenges remain, preventing the design from being a comprehensive solution. In this paper, we propose SupeRBNN, an AQFP-based randomized BNN acceleration framework that leverages softwarehardware co-optimization to eventually make the AQFP devices a feasible solution for BNN acceleration. Specifically, we investigate the randomized behavior of the AQFP devices and analyze the impact of crossbar size on current attenuation, subsequently formulating the current amplitude into the values suitable for use in BNN computation. To tackle the accumulation problem and improve overall hardware performance, we propose a stochastic computing-based accumulation module and a clocking scheme adjustment-based circuit optimization method. To effectively train the BNN models that are compatible with the distinctive characteristics of AQFP devices, we further propose a novel randomized BNN training solution that utilizes algorithm-hardware co-optimization, enabling simultaneous optimization of hardware configurations. In addition, we propose implementing batch normalization matching and the weight rectified clamp method to further improve the overall performance. We validate our SupeRBNN framework across various datasets and network architectures, comparing it with implementations based on different technologies, including CMOS, ReRAM, and superconducting RSFQ/ERSFQ. Experimental results demonstrate that our design achieves an energy efficiency of approximately 7.8 x 10(4) times higher than that of the ReRAM-based BNN framework while maintaining a similar level of model accuracy. Furthermore, when compared with superconductor-based counterparts, our framework demonstrates at least two orders of magnitude higher energy efficiency.
更多查看译文
关键词
Deep Learning,Superconducting,AQFP,BNN,Stochastic Computing
AI 理解论文
溯源树
样例
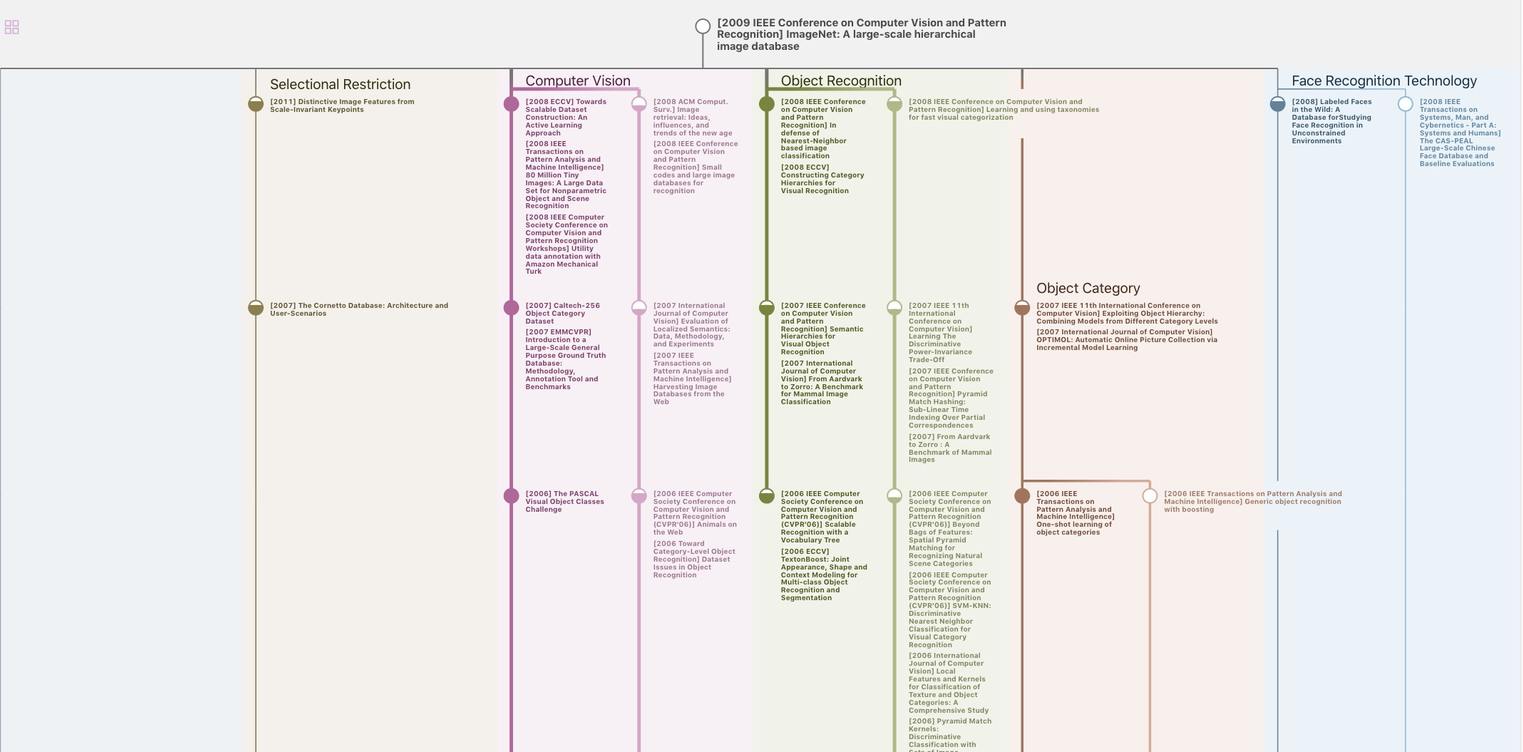
生成溯源树,研究论文发展脉络
Chat Paper
正在生成论文摘要