Enhancing SAEAs with unevaluated solutions: a case study of relation model for expensive optimization
Science China Information Sciences(2024)
摘要
Surrogate-assisted evolutionary algorithms (SAEAs) hold significant importance in resolving expensive optimization problems. Extensive efforts have been devoted to improving the efficiency of SAEAs through the development of proficient model-assisted solution selection methods, which, however, first requires generating high-quality solutions. The fundamental paradigm of evaluating a limited number of solutions in each generation within SAEAs reduces the variance of adjacent populations, thereby affecting the quality of offspring solutions. This is a frequently encountered issue yet receives little attention. To address the issue, this paper presents a framework using unevaluated solutions to enhance the efficiency of SAEAs. The surrogate model is employed to identify high-quality solutions for the direct generation of new solutions without evaluation. To ensure dependable selection, we have introduced two tailored relation models to select the optimal solution and the unevaluated population. A comprehensive experimental analysis is performed on two test suites, which showcases the superiority of the relation model over regression and classification models in the solution selection phase. Furthermore, the surrogate-selected unevaluated solutions with high potential have been shown to enhance the efficiency of the algorithm significantly.
更多查看译文
关键词
expensive optimization,unevaluated solutions,relation model,surrogate-assisted evolutionary algorithm
AI 理解论文
溯源树
样例
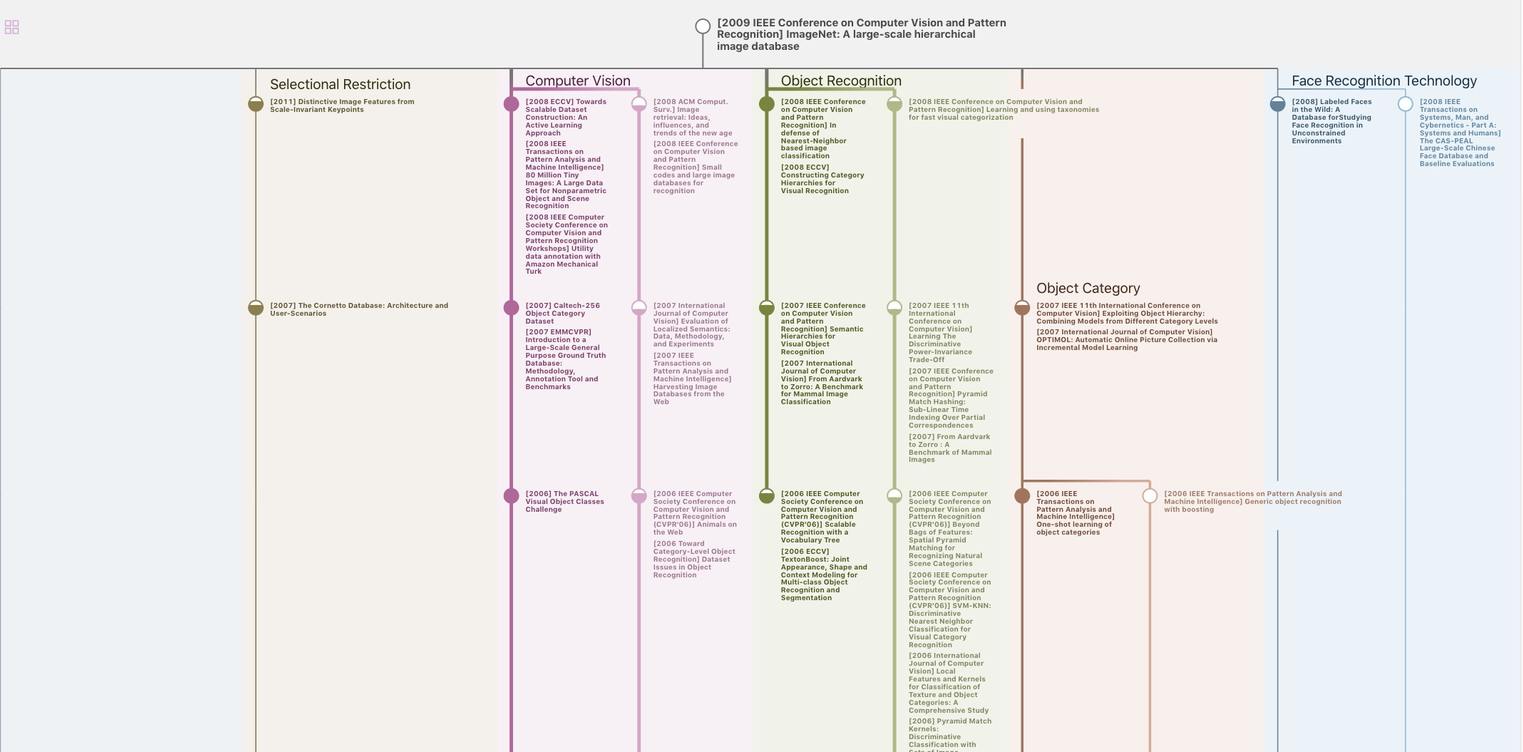
生成溯源树,研究论文发展脉络
Chat Paper
正在生成论文摘要