MTANet: Multi-Task Attention Network for Automatic Medical Image Segmentation and Classification.
IEEE transactions on medical imaging(2024)
摘要
Medical image segmentation and classification are two of the most key steps in computer-aided clinical diagnosis. The region of interest were usually segmented in a proper manner to extract useful features for further disease classification. However, these methods are computationally complex and time-consuming. In this paper, we proposed a one-stage multi-task attention network (MTANet) which efficiently classifies objects in an image while generating a high-quality segmentation mask for each medical object. A reverse addition attention module was designed in the segmentation task to fusion areas in global map and boundary cues in high-resolution features, and an attention bottleneck module was used in the classification task for image feature and clinical feature fusion. We evaluated the performance of MTANet with CNN-based and transformer-based architectures across three imaging modalities for different tasks: CVC-ClinicDB dataset for polyp segmentation, ISIC-2018 dataset for skin lesion segmentation, and our private ultrasound dataset for liver tumor segmentation and classification. Our proposed model outperformed state-of-the-art models on all three datasets and was superior to all 25 radiologists for liver tumor diagnosis.
更多查看译文
关键词
medical image segmentation,medical image classification,attention,one-stage
AI 理解论文
溯源树
样例
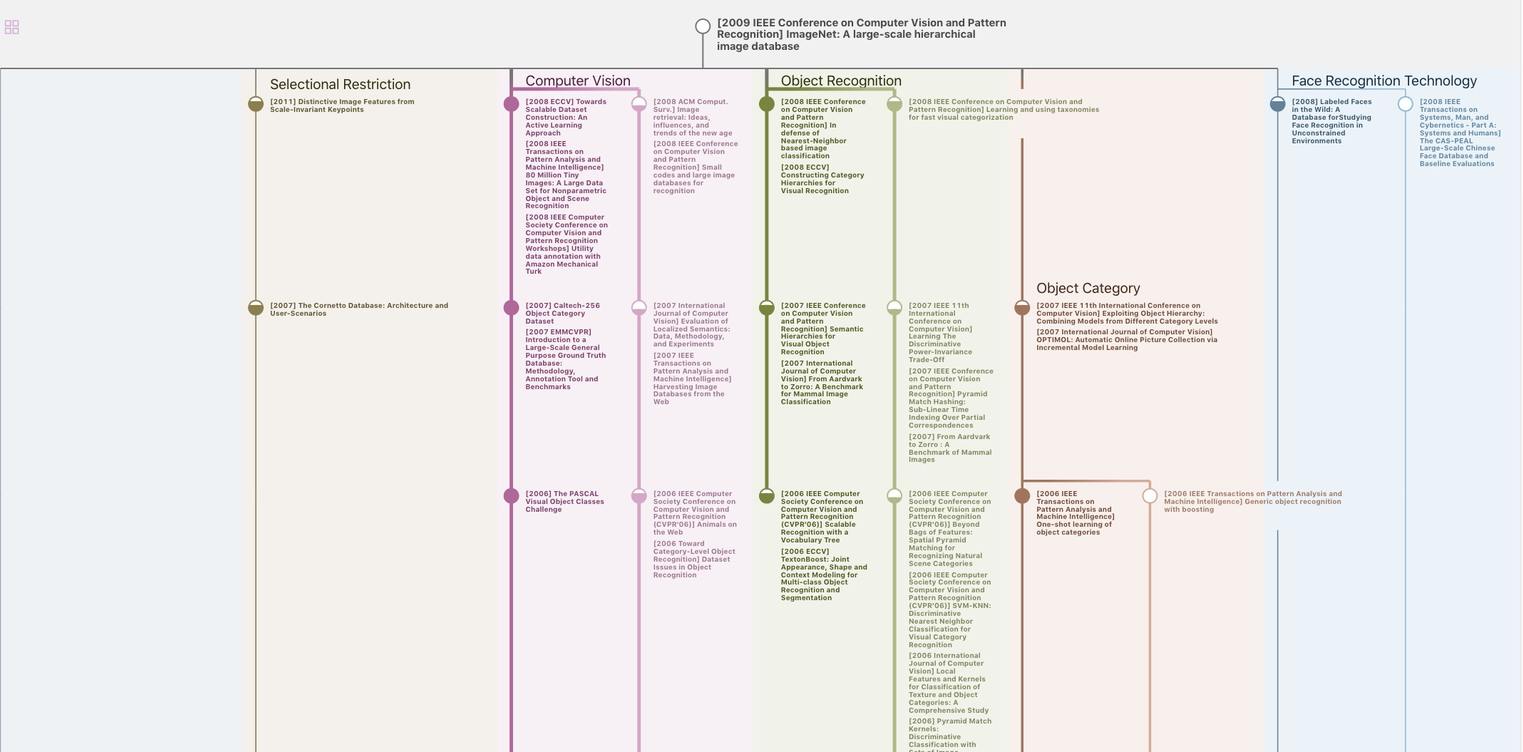
生成溯源树,研究论文发展脉络
Chat Paper
正在生成论文摘要