Bridging the Gap Between Spiking Neural Networks & LSTMs for Latency & Energy Efficiency
2023 IEEE/ACM International Symposium on Low Power Electronics and Design (ISLPED)(2023)
摘要
Spiking Neural Networks (SNNs) have emerged as an attractive spatio-temporal computing paradigm for complex vision tasks. However, most existing works yield models that require many time steps and do not leverage the inherent temporal dynamics of spiking neural networks, even for sequential tasks. Motivated by this observation, we propose an optimized spiking long short-term memory networks (LSTM) training framework that involves a novel ANN-to-SNN conversion framework, followed by SNN fine-tuning via backpropagation through time (BPTT). In particular, we propose novel activation functions in the source LSTM architecture and convert a judiciously selected subset of them to leaky-integrate-and-fire (LIF) activations with optimal bias shifts. Moreover, we propose a pipelined parallel processing scheme that hides the SNN time steps, significantly improving system latency, especially for long sequences. The resulting SNNs have high activation sparsity and require only accumulate operations (AC), in contrast to expensive multiply-and-accumulates (MAC) needed for ANNs, except for the input layer when using direct encoding, yielding significant improvements in energy efficiency. We evaluate our framework on sequential learning tasks including temporal MNIST, Google Speech Commands (GSC), and UCI Smartphone datasets on different LSTM architectures. We obtain test accuracy of 94.75 % with only 2 time steps on the GSC dataset with
$\sim 4.1\times$
lower energy than an iso-architecture standard LSTM.
更多查看译文
AI 理解论文
溯源树
样例
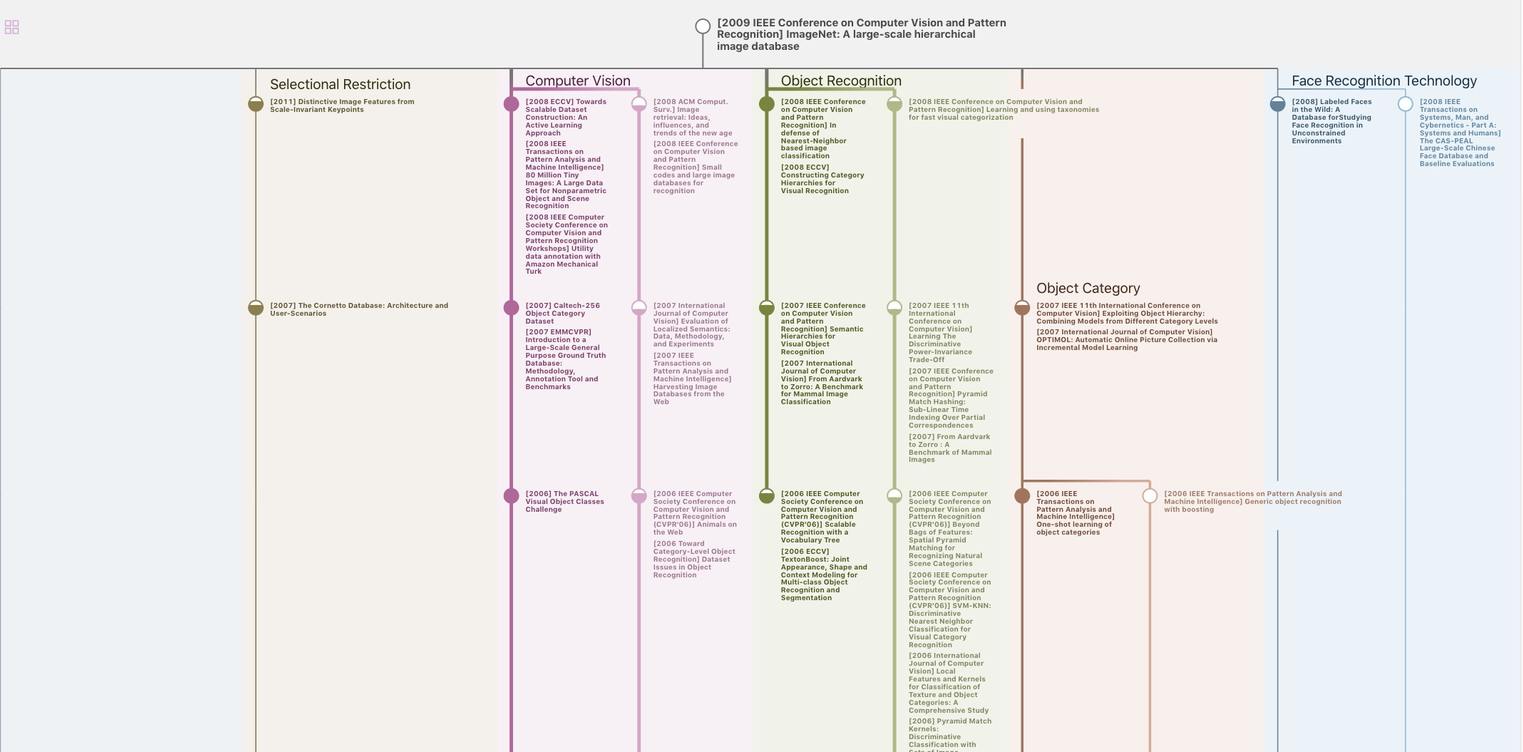
生成溯源树,研究论文发展脉络
Chat Paper
正在生成论文摘要