Diffusion-based speech enhancement with a weighted generative-supervised learning loss
ICASSP 2024 - 2024 IEEE International Conference on Acoustics, Speech and Signal Processing (ICASSP)(2023)
摘要
Diffusion-based generative models have recently gained attention in speech enhancement (SE), providing an alternative to conventional supervised methods. These models transform clean speech training samples into Gaussian noise centered at noisy speech, and subsequently learn a parameterized model to reverse this process, conditionally on noisy speech. Unlike supervised methods, generative-based SE approaches usually rely solely on an unsupervised loss, which may result in less efficient incorporation of conditioned noisy speech. To address this issue, we propose augmenting the original diffusion training objective with a mean squared error (MSE) loss, measuring the discrepancy between estimated enhanced speech and ground-truth clean speech at each reverse process iteration. Experimental results demonstrate the effectiveness of our proposed methodology.
更多查看译文
关键词
Speech enhancement,diffusion models,generative modeling,supervised learning
AI 理解论文
溯源树
样例
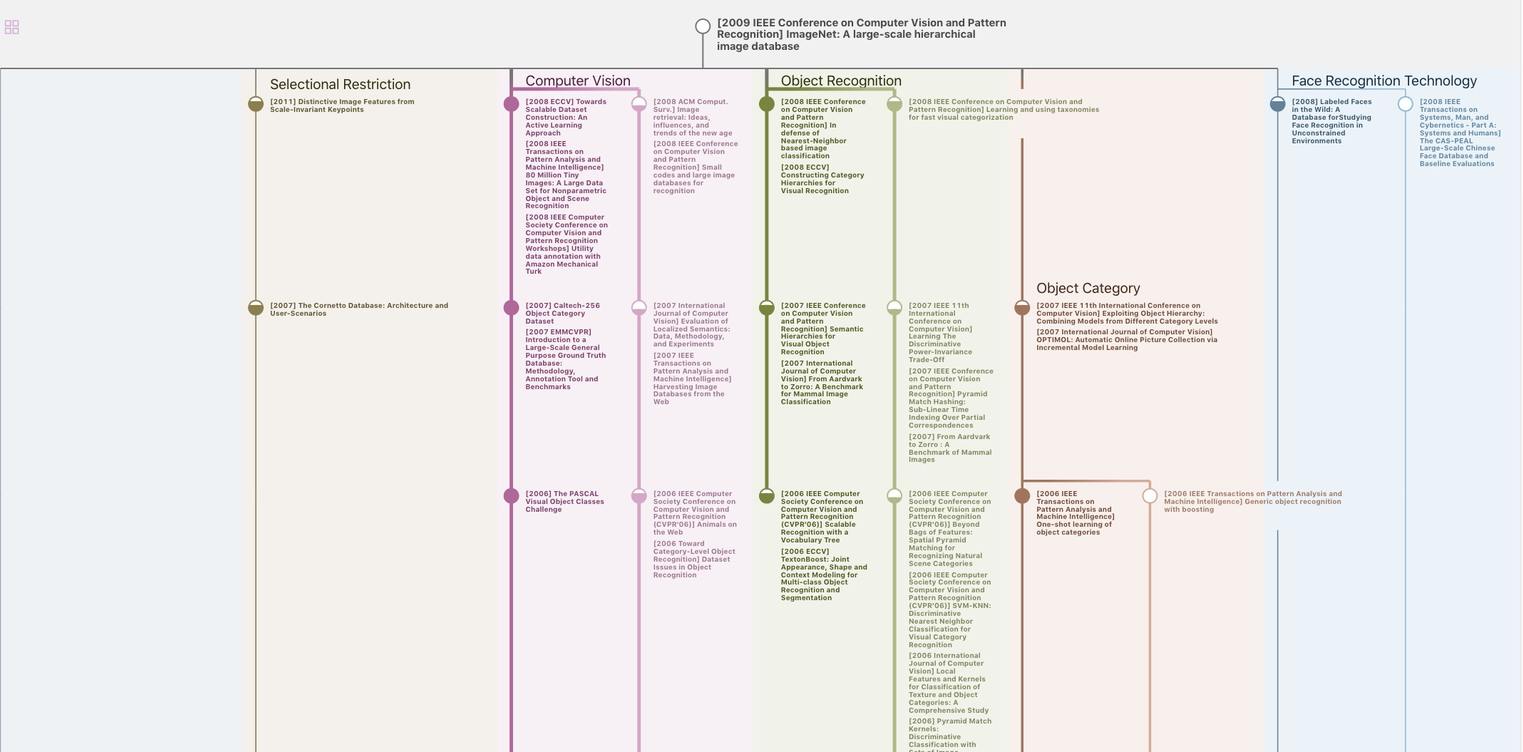
生成溯源树,研究论文发展脉络
Chat Paper
正在生成论文摘要