Cluster-aware channel estimation with deep learning method in deep-water acoustic communications.
The Journal of the Acoustical Society of America(2023)
摘要
In underwater acoustic (UWA) communications, channels often exhibit a clustered-sparse structure, wherein most of the channel impulse responses are near zero, and only a small number of nonzero taps assemble to form clusters. Several algorithms have used the time-domain sparse characteristic of UWA channels to reduce the complexity of channel estimation and improve the accuracy. Employing the clustered structure to enhance channel estimation performance provides another promising research direction. In this work, a deep learning-based channel estimation method for UWA orthogonal frequency division multiplexing (OFDM) systems is proposed that leverages the clustered structure information. First, a cluster detection model based on convolutional neural networks is introduced to detect the cluster of UWA channels. This method outperforms the traditional Page test algorithm with better accuracy and robustness, particularly in low signal-to-noise ratio conditions. Based on the cluster detection model, a cluster-aware distributed compressed sensing channel estimation method is proposed, which reduces the noise-induced errors by exploiting the joint sparsity between adjacent OFDM symbols and limiting the search space of channel delay spread. Numerical simulation and sea trial results are provided to illustrate the superior performance of the proposed approach in comparison with existing sparse UWA channel estimation methods.
更多查看译文
关键词
deep learning method,deep learning,communications,cluster-aware,deep-water
AI 理解论文
溯源树
样例
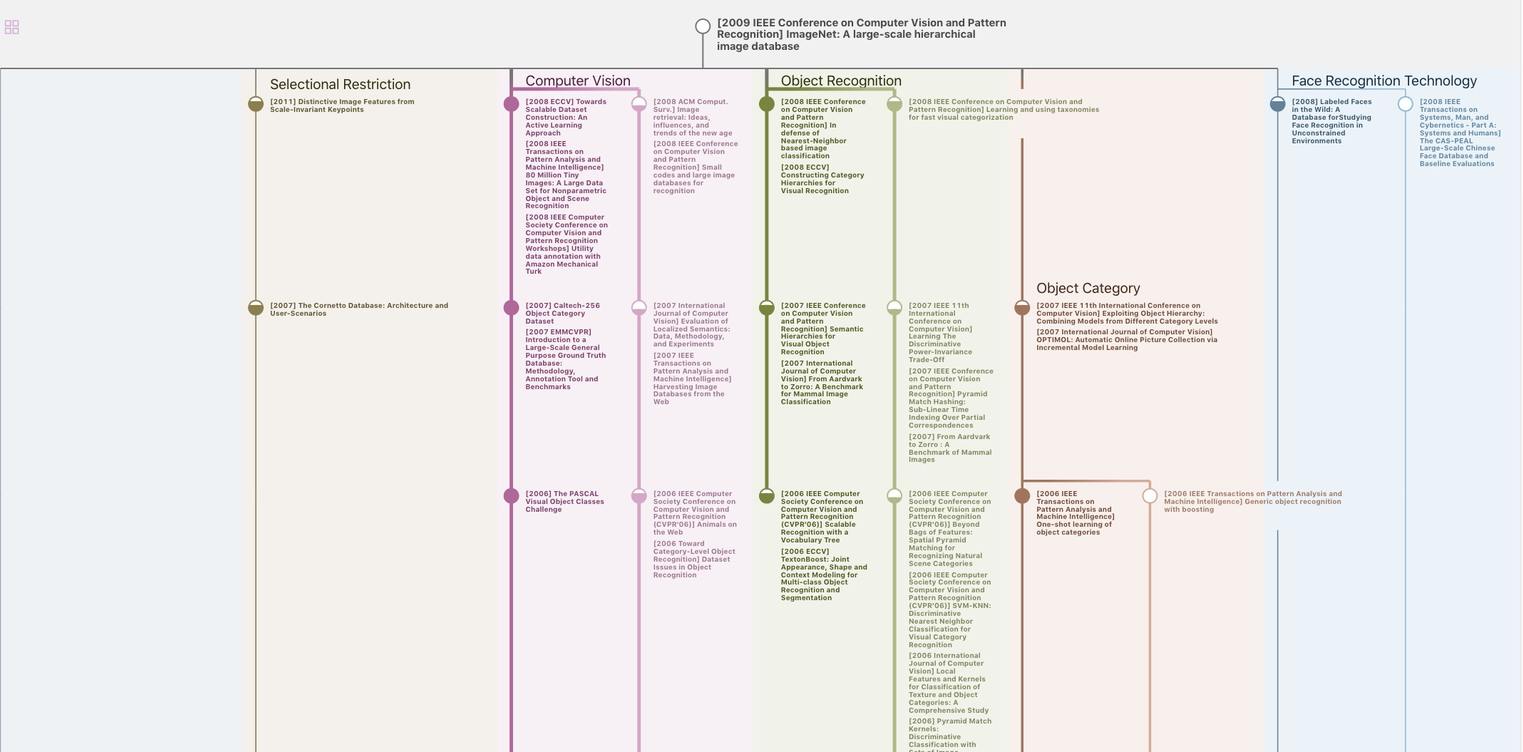
生成溯源树,研究论文发展脉络
Chat Paper
正在生成论文摘要