Hierarchical Attention and Graph Neural Networks: Toward Drift-Free Pose Estimation
CoRR(2023)
摘要
The most commonly used method for addressing 3D geometric registration is the iterative closet-point algorithm, this approach is incremental and prone to drift over multiple consecutive frames. The Common strategy to address the drift is the pose graph optimization subsequent to frame-to-frame registration, incorporating a loop closure process that identifies previously visited places. In this paper, we explore a framework that replaces traditional geometric registration and pose graph optimization with a learned model utilizing hierarchical attention mechanisms and graph neural networks. We propose a strategy to condense the data flow, preserving essential information required for the precise estimation of rigid poses. Our results, derived from tests on the KITTI Odometry dataset, demonstrate a significant improvement in pose estimation accuracy. This improvement is especially notable in determining rotational components when compared with results obtained through conventional multi-way registration via pose graph optimization. The code will be made available upon completion of the review process.
更多查看译文
关键词
graph neural networks,attention,drift-free
AI 理解论文
溯源树
样例
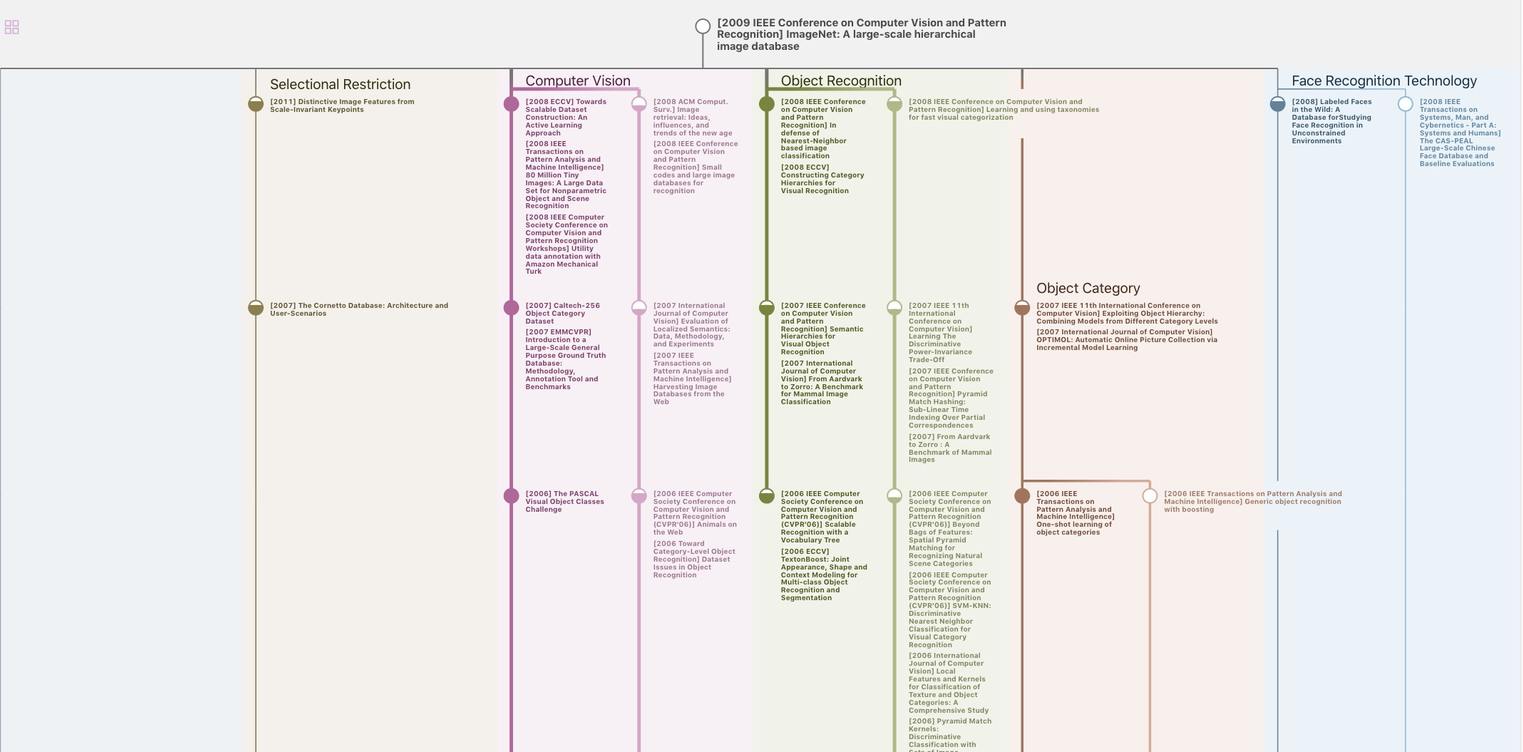
生成溯源树,研究论文发展脉络
Chat Paper
正在生成论文摘要