Efficient Concept Drift Handling for Batch Android Malware Detection Models
Pervasive and Mobile Computing(2023)
摘要
The rapidly evolving nature of Android apps poses a significant challenge to static batch machine learning algorithms employed in malware detection systems, as they quickly become obsolete. Despite this challenge, the existing literature pays limited attention to addressing this issue, with many advanced Android malware detection approaches, such as Drebin, DroidDet and MaMaDroid, relying on static models. In this work, we show how retraining techniques are able to maintain detector capabilities over time. Particularly, we analyze the effect of two aspects in the efficiency and performance of the detectors: 1) the frequency with which the models are retrained, and 2) the data used for retraining. In the first experiment, we compare periodic retraining with a more advanced concept drift detection method that triggers retraining only when necessary. In the second experiment, we analyze sampling methods to reduce the amount of data used to retrain models. Specifically, we compare fixed sized windows of recent data and state-of-the-art active learning methods that select those apps that help keep the training dataset small but diverse. Our experiments show that concept drift detection and sample selection mechanisms result in very efficient retraining strategies which can be successfully used to maintain the performance of the static Android malware state-of-the-art detectors in changing environments.
更多查看译文
关键词
Android malware detection,Machine learning,Mobile security,Concept drift,Static analysis
AI 理解论文
溯源树
样例
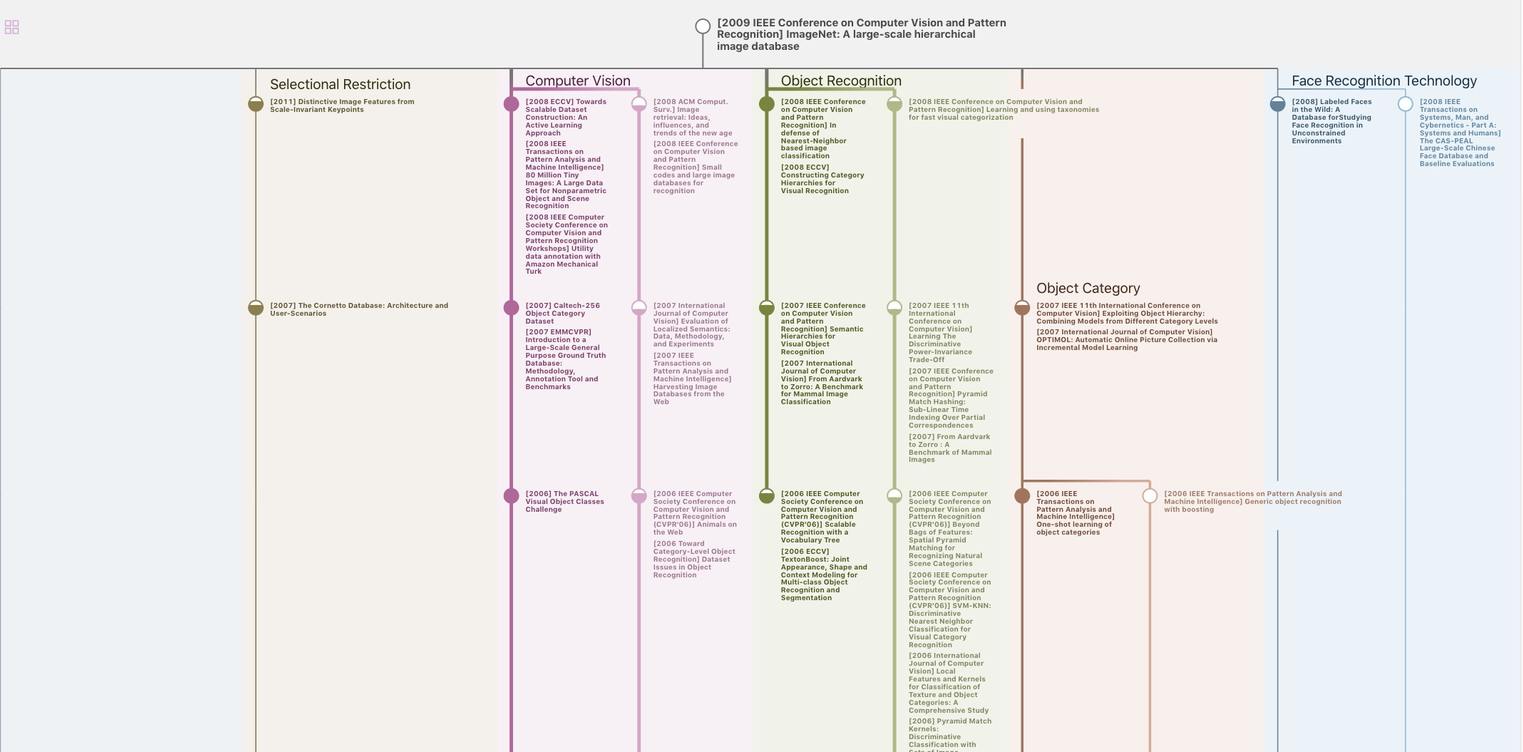
生成溯源树,研究论文发展脉络
Chat Paper
正在生成论文摘要