Noise-Augmented Boruta: The Neural Network Perturbation Infusion with Boruta Feature Selection
CoRR(2023)
摘要
With the surge in data generation, both vertically (i.e., volume of data) and horizontally (i.e., dimensionality), the burden of the curse of dimensionality has become increasingly palpable. Feature selection, a key facet of dimensionality reduction techniques, has advanced considerably to address this challenge. One such advancement is the Boruta feature selection algorithm, which successfully discerns meaningful features by contrasting them to their permutated counterparts known as shadow features. However, the significance of a feature is shaped more by the data's overall traits than by its intrinsic value, a sentiment echoed in the conventional Boruta algorithm where shadow features closely mimic the characteristics of the original ones. Building on this premise, this paper introduces an innovative approach to the Boruta feature selection algorithm by incorporating noise into the shadow variables. Drawing parallels from the perturbation analysis framework of artificial neural networks, this evolved version of the Boruta method is presented. Rigorous testing on four publicly available benchmark datasets revealed that this proposed technique outperforms the classic Boruta algorithm, underscoring its potential for enhanced, accurate feature selection.
更多查看译文
关键词
boruta feature selection,neural network perturbation infusion,neural network,noise-augmented
AI 理解论文
溯源树
样例
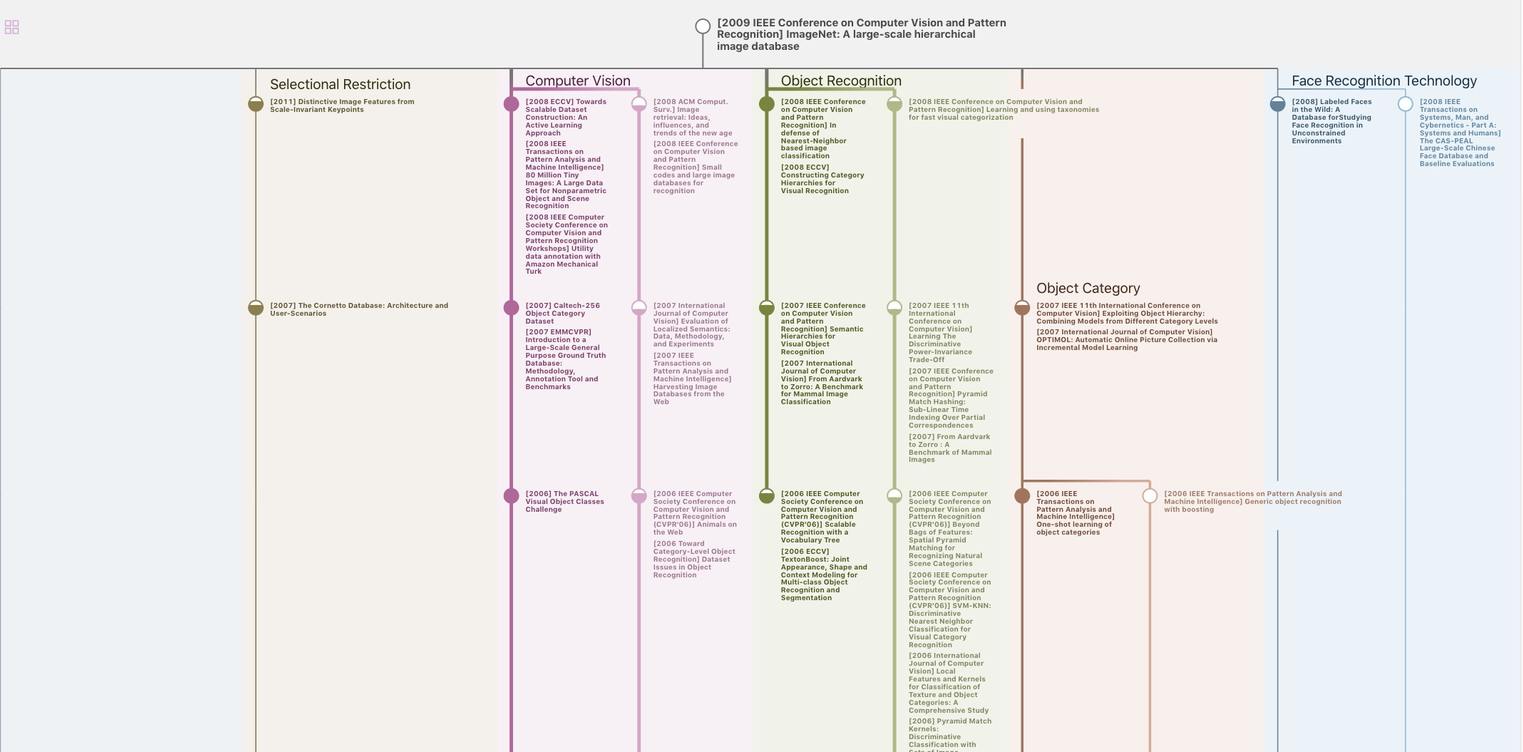
生成溯源树,研究论文发展脉络
Chat Paper
正在生成论文摘要