Self-supervised TransUNet for Ultrasound regional segmentation of the distal radius in children
CoRR(2023)
摘要
Supervised deep learning offers great promise to automate analysis of medical images from segmentation to diagnosis. However, their performance highly relies on the quality and quantity of the data annotation. Meanwhile, curating large annotated datasets for medical images requires a high level of expertise, which is time-consuming and expensive. Recently, to quench the thirst for large data sets with high-quality annotation, self-supervised learning (SSL) methods using unlabeled domain-specific data, have attracted attention. Therefore, designing an SSL method that relies on minimal quantities of labeled data has far-reaching significance in medical images. This paper investigates the feasibility of deploying the Masked Autoencoder for SSL (SSL-MAE) of TransUNet, for segmenting bony regions from children's wrist ultrasound scans. We found that changing the embedding and loss function in SSL-MAE can produce better downstream results compared to the original SSL-MAE. In addition, we determined that only pretraining TransUNet embedding and encoder with SSL-MAE does not work as well as TransUNet without SSL-MAE pretraining on downstream segmentation tasks.
更多查看译文
关键词
ultrasound,distal radius,regional segmentation,transunet,self-supervised
AI 理解论文
溯源树
样例
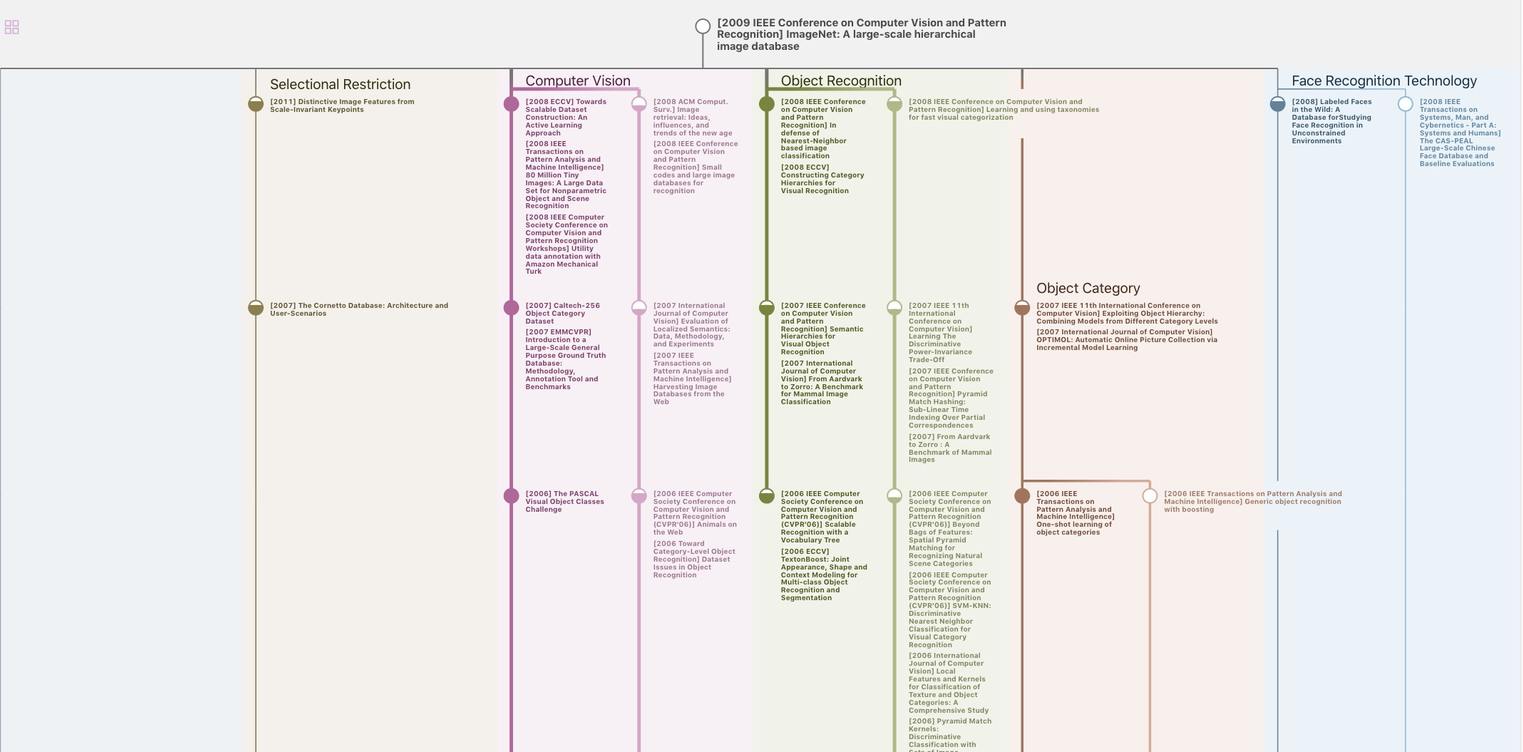
生成溯源树,研究论文发展脉络
Chat Paper
正在生成论文摘要