Rethinking STS and NLI in Large Language Models
arXiv (Cornell University)(2023)
摘要
In this study, we aim to rethink STS and NLI in the era of large language models (LLMs). We first evaluate the accuracy of clinical/biomedical STS and NLI over five datasets, and then we assess LLM predictive confidence and their capability of capturing collective human opinions. We find that LLMs may be able to provide personalised descriptions for a specific topic, or to generate semantically similar content in different tones, but that this is hard for current LLMs to make personalised judgements or decisions. We further find that zero-shot ChatGPT achieves competitive accuracy over clinical and biomedical STS/NLI, constraining to the fine-tuned BERT-base. However, there is a large variation in sampling, ensembled results perform the best.
更多查看译文
关键词
sts,nli,language,models
AI 理解论文
溯源树
样例
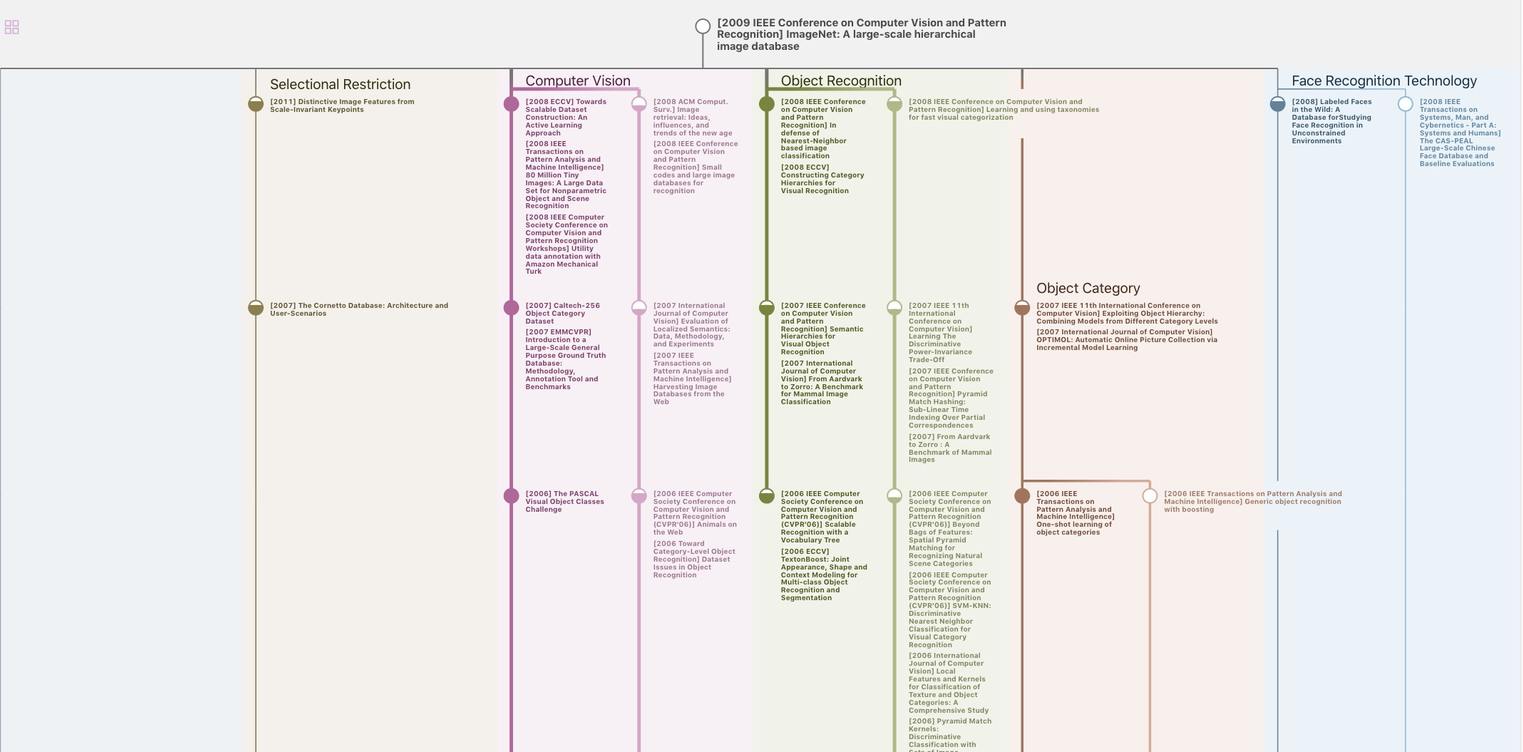
生成溯源树,研究论文发展脉络
Chat Paper
正在生成论文摘要