A More Secure Split: Enhancing the Security of Privacy-Preserving Split Learning
SECURE IT SYSTEMS, NORDSEC 2023(2024)
摘要
Split learning (SL) is a new collaborative learning technique that allows participants, e.g. a client and a server, to train machine learning models without the client sharing raw data. In this setting, the client initially applies its part of the machine learning model on the raw data to generate Activation Maps (AMs) and then sends them to the server to continue the training process. Previous works in the field demonstrated that reconstructing AMs could result in privacy leakage of client data. In addition to that, existing mitigation techniques that overcome the privacy leakage of SL prove to be significantly worse in terms of accuracy. In this paper, we improve upon previous works by constructing a protocol based on U-shaped SL that can operate on homomorphically encrypted data. More precisely, in our approach, the client applies homomorphic encryption on the AMs before sending them to the server, thus protecting user privacy. This is an important improvement that reduces privacy leakage in comparison to other SL-based works. Finally, our results show that, with the optimum set of parameters, training with HE data in the U-shaped SL setting only reduces accuracy by 2.65% compared to training on plaintext. In addition, raw training data privacy is preserved.
更多查看译文
关键词
Activation Maps,Homomorphic Encryption,Machine Learning,Privacy,Split Learning
AI 理解论文
溯源树
样例
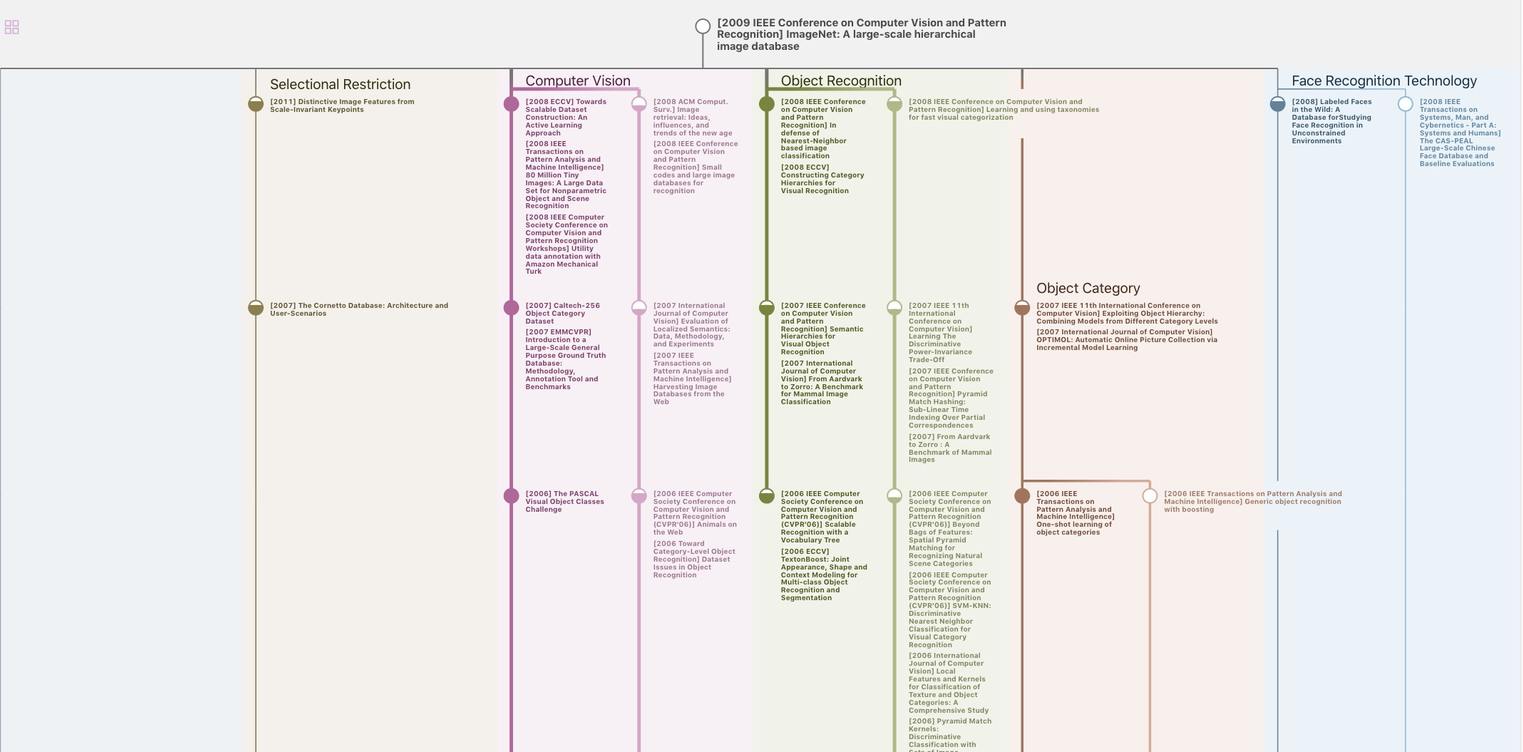
生成溯源树,研究论文发展脉络
Chat Paper
正在生成论文摘要