High-Dimensional Bernstein Von-Mises Theorems for Covariance and Precision Matrices
arXiv (Cornell University)(2023)
摘要
This paper aims to examine the characteristics of the posterior distribution of covariance/precision matrices in a "large $p$, large $n$" scenario, where $p$ represents the number of variables and $n$ is the sample size. Our analysis focuses on establishing asymptotic normality of the posterior distribution of the entire covariance/precision matrices under specific growth restrictions on $p_n$ and other mild assumptions. In particular, the limiting distribution turns out to be a symmetric matrix variate normal distribution whose parameters depend on the maximum likelihood estimate. Our results hold for a wide class of prior distributions which includes standard choices used by practitioners. Next, we consider Gaussian graphical models which induce sparsity in the precision matrix. Asymptotic normality of the corresponding posterior distribution is established under mild assumptions on the prior and true data-generating mechanism.
更多查看译文
关键词
bernstein,covariance,theorems,high-dimensional,von-mises
AI 理解论文
溯源树
样例
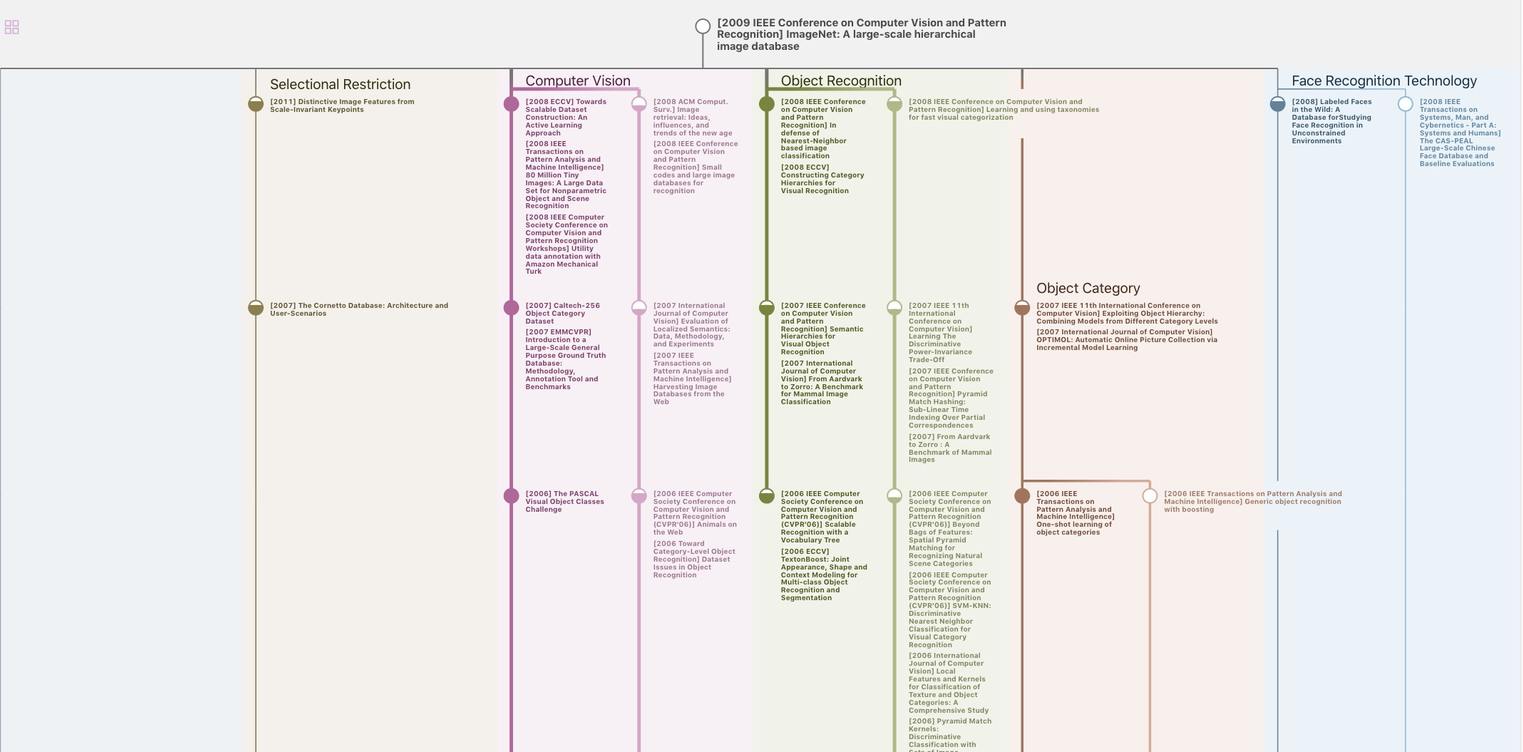
生成溯源树,研究论文发展脉络
Chat Paper
正在生成论文摘要