T-UDA: Temporal Unsupervised Domain Adaptation in Sequential Point Clouds
2023 IEEE/RSJ INTERNATIONAL CONFERENCE ON INTELLIGENT ROBOTS AND SYSTEMS (IROS)(2023)
摘要
Deep perception models have to reliably cope with an open-world setting of domain shifts induced by different geographic regions, sensor properties, mounting positions, and several other reasons. Since covering all domains with annotated data is technically intractable due to the endless possible variations, researchers focus on unsupervised domain adaptation (UDA) methods that adapt models trained on one (source) domain with annotations available to another (target) domain for which only unannotated data are available. Current predominant methods either leverage semi-supervised approaches, e.g., teacher-student setup, or exploit privileged data, such as other sensor modalities or temporal data consistency. We introduce a novel domain adaptation method that leverages the best of both approaches. Our approach combines input data's temporal and cross-sensor geometric consistency with the mean teacher method. Dubbed T-UDA for "temporal UDA", such a combination yields massive performance gains for the task of 3D semantic segmentation of driving scenes. Experiments are conducted on Waymo Open Dataset, nuScenes, and SemanticKITTI, for two popular 3D point cloud architectures, Cylinder3D and MinkowskiNet. Our codes are publicly available on https://github.com/ctu-vras/T- UDA.
更多查看译文
AI 理解论文
溯源树
样例
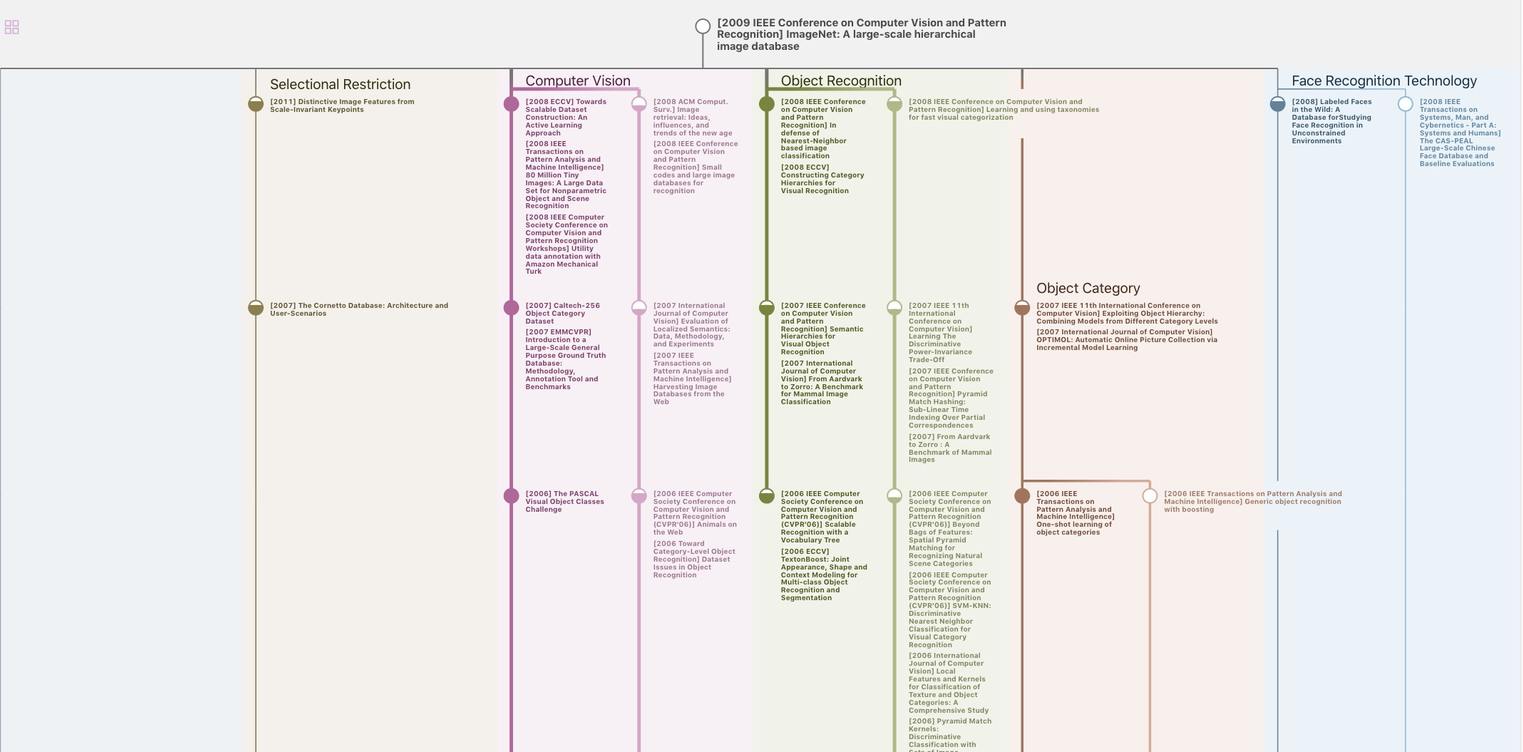
生成溯源树,研究论文发展脉络
Chat Paper
正在生成论文摘要